Table of Contents
Introduction
In the age of data driven decision-making, companies are leveraging the power of AI to gain a competitive advantage, streamline processes, and transform innovation. However, the integration of AI into core business operations is not always easy. That’s where ML-Ops comes in. ML-Ops is a bridge between machine learning and operations. It has the potential to accelerate the AI journey.
In this article, we will explore the areas of the ML-Ops roadblocks — obstacles that, if left unchecked, can hinder your business’s AI capabilities. Join us on a journey to uncover these challenges as well as actionable solutions, providing organizations with the tools and expertise they need to navigate the complex journey of ML-Ops and ensure that AI becomes a driving force for success and innovation.
Importance of ML-Ops for Enterprises
- To achieve a logical and optimized ML-Ops approach, high-quality data streams and effective data categorization are two essential components. High-Quality Data Streams guarantee the accuracy, reliability, and timeliness of the data utilized for machine learning model inference and training. High-quality data reduces errors and enhances the performance of models.
- Data cataloging system provides a comprehensive, intuitive, and comprehensive inventory of your data resources. High-quality data streams and an efficient data cataloguing system are used to strengthen and streamlined ML-Ops process, which is intended to not only optimize present operations but also prepare organization for the uncertainties and challenges of the future.
- The three crucial pillars of Audit Trails, Reproducibility, and Explainability help organizations meet the imperative for transparency, control, and accountability as they adopt AI and ML models to boost innovation and competitiveness in their key operations.
Challenges during Implementation of ML-Ops for Enterprises
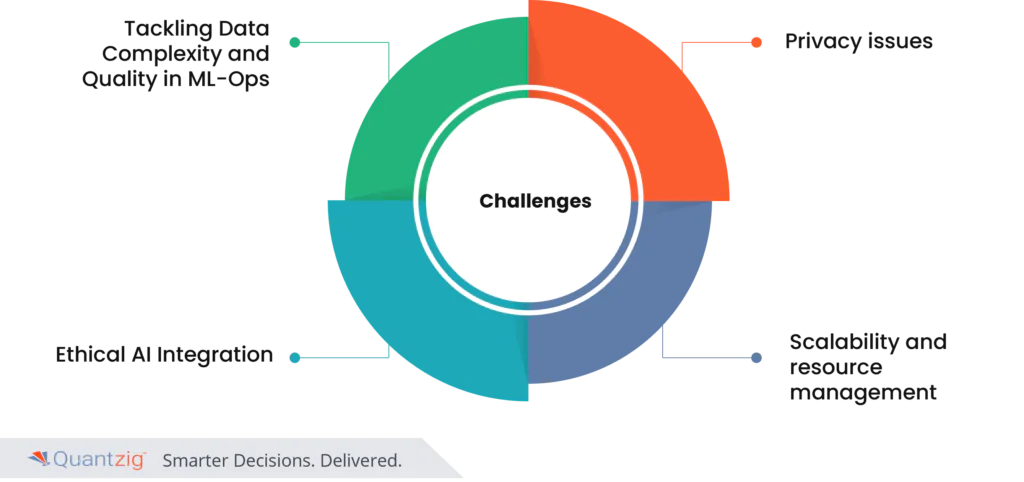
- Tackling Data Complexity and Quality in ML-Ops- Handling data from various sources with different formats and qualities can be quite challenging in ML-Ops. It often leads to delays due to complex integration, and ensuring data remains reliable and compliant is an ongoing challenge. To succeed in the Business AI Journey while minimizing risks, it is crucial to take a comprehensive approach, encompassing data preparation, governance, and monitoring.
- Privacy issues – Strong Privacy preserving strategies are necessary to safeguard sensitive consumer data and ensure ethical AI practices, particularly in healthcare and finance.
- Ethical AI Integration-In ML-Ops, a significant challenge is to ensure the ethical use of AI models. This requires solutions that are just and responsible. Fairness implies that AI systems should not discriminate, and accountability means AI decisions should be traceable and reproducible. To achieve ethical AI, ML-Ops must carefully handle data, employ bias mitigation techniques, and establish transparent model governance.
- Scalability and resource management – As AI and ML models are increasingly employed in companies, it becomes more difficult to manage infrastructure and effectively manage resources. ML-Ops must manage growing data quantities, complicated models, and the need for resource allocation that is economical.
Benefits of implementing ML-OPS for Enterprises
1. Ensuring Trustworthy AI Integration
Consistent data and decision-making across ML models not only enhance operational effectiveness, but also make it easier to integrate AI into crucial operations that depend on humans and manual work. This coordinated approach ensures a smoother business AI journey by reducing errors, enhancing decision-making, and increasing trust.
2. Accelerating Data-Driven Transformations
The deployment of advanced analytics and machine learning models is simplified and accelerated by ML-Ops, enabling Enterprises to quickly transform data-driven insights into effective business strategies. Due to this efficiency, Enterprises can remain ahead in the evolving analytics landscape and reduce their time to value.
What our Capability does
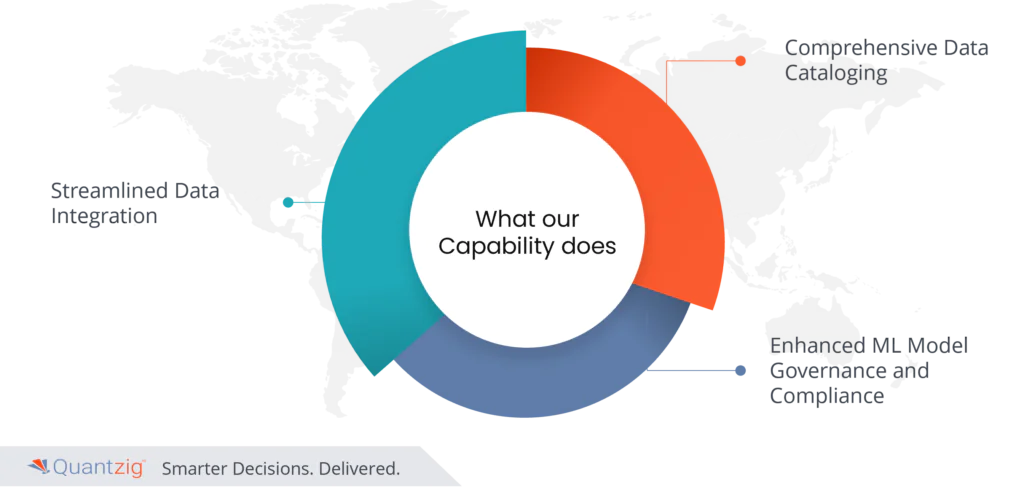
1. Streamlined Data Integration
Combining our powerful visual workflow manager and data ingestion and cataloging capabilities via a Metadata Editor, our solution provides a comprehensive solution for organizations utilizing multiple data sources.
In today’s data landscape, organizations often struggle to manage data from multiple, siloed, and discrete sources. Quantzig provided a unified approach to data integration. By providing a single platform from which data from multiple sources can be easily accessed, structured, and made available, our solution eliminates the complexity associated with dealing with multiple data formats and data structures. By streamlining data operations, our solution empowers teams with the ability to enhance data seamlessly.
2. Comprehensive Data Cataloging
Our Data Cataloging powered by Metadata editor is designed for a complete and easy-to-use view of organization’s data assets. Each asset is documented in detail, providing you with valuable insights into where it originated, how it was organized, and where it is going. Data catalog helps data professionals, analysts and business users find the data they need quickly and easily.
Data Cataloging improved data discovery and helped in gaining a deeper understanding of data resources. Not only does it reduce the time spend searching for data, but it also helps make informed decisions based on reliable and documented data assets. Data cataloging is the foundation for organizations looking to streamline their data operations and use data as a strategic resource.
3. Enhanced ML Model Governance and Compliance
Our ML-Ops solutions are designed to provide ML models with thorough governance and compliance processes. By introducing traceability and reproducibility, they ensure that each stage of the ML model’s lifecycle is completely recorded. This includes maintaining an ongoing audit trail of all model actions, from training and deployment, to promote accountability and transparency. Additionally, our systems possess strong exception management mechanisms that allow for the quick handling of unexpected circumstances while maintaining process governance. This enhances model consistency and ensures compliance with legal requirements and internal governance standards.
Why should a client opt for our offering?
1. Accelerated AI/ML Model Integration
One of the main reasons clients choose us is because of our Prebuilt solutions. Our pre-built solutions enable you to quickly and easily integrate your AI/ML model into BaaS (Business as Usual) and critical processes. Speed and efficiency are essential for any successful AI/ML integration. Our solutions simplify this process, allowing Organizations to deploy AI/ML seamlessly and quickly. With our pre-built solutions, Organizations will be able to gain the most out of their AI/ML Models, gain a competitive advantage, and maximize the value of data.
2. Enhanced Trust and Adoption of AI
Our solutions are created to bridge the gap between technology and people, assuring that AI is not only effective but also dependable and user-friendly. By utilizing AI, we increase its usability and relatability, which ultimately leads to faster user and stakeholder adoption. Our product is an attractive option for businesses seeking to fully comprehend the potential of artificial intelligence projects because of the increased trust and dependability that are essential to the successful deployment of AI technologies.
Conclusion
In Conclusion, Businesses that need to use AI to its fullest potential may find it difficult to navigate the ML-Ops realm. These ML-Ops obstacles can be overcome by employing the appropriate techniques, technological advancements, and a dedication to innovation. Adopting ML-Ops best practices is not only necessary, but also a competitive advantage that can organizations on the road to long-term success in the world of AI.