Table of Contents
Introduction
AI is the simulation of the human intelligence process by numerous machines, particularly computer systems. This technology generally works by ingesting a large volume of labeled training data, analyzing all that data for patterns and correlations, and using those patterns to make predictions for future states.
The transformation of machine learning (ML) and artificial intelligence (AI) from computer science theories into various real-world technologies is the primary enabler of Industry 4.0, to the extent that it integrates these emerging technologies to transform industry. Industries and governments worldwide have successfully addressed the strategic implications of AI/ML technologies and launched many initiatives seeking to capitalize on and explore this innovative revolution by incorporating artificial intelligence and machine learning in manufacturing industry. In a landscape where innovation and competitiveness are paramount, scaling AI in manufacturing operations emerges as not just a choice, but an imperative for a smarter, greener future. This article uncovers the driving forces behind the escalating integration of AI in manufacturing operations.
Importance of scaling AI in manufacturing Operations
- Artificial Intelligence in manufacturing can help with real-time intelligence and remediation required on the manufacturing floor for quick corrective actions. AI can swiftly detect anomalies, deviations, or potential issues by continuously monitoring various aspects of the manufacturing process. This early recognition empowers manufacturers to take proactive measures before minor glitches snowball into significant disruptions. Whether it’s identifying machinery malfunctions, irregularities in production lines, or variations in product quality, AI excels at providing timely alerts. Moreover, AI extends beyond just highlighting problems; it assists in devising effective remediation strategies. Leveraging historical data and patterns, AI suggests appropriate corrective actions, enabling operators to rectify issues promptly. This not only minimizes downtime but also bolsters overall efficiency and product quality. In essence, AI’s real-time intelligence and its seamless integration with remediation processes empower manufacturing operations to swiftly address challenges, optimize workflows, and maintain a seamless production pace.
- AI can help automate tasks, optimize processes, and identify areas for improvement and cost reductions. Manufacturers use this technology to analyze sensor data and predict accidents and breakdowns. Synthetic intelligence systems assist production facilities in identifying the chances of future failures in operational machinery, allowing for repairs to be scheduled in advance and preventative maintenance. Through advanced algorithms, AI can automate repetitive and time-consuming tasks, freeing up human resources for more strategic and creative endeavors. Its process optimization capabilities stem from its ability to analyze intricate data patterns. It identifies inefficiencies, bottlenecks, and areas ready for enhancement, guiding organizations to refine workflows and bolster operational efficiency. Additionally, its data-driven insights illuminate avenues for cost reduction. By identifying resource wastage or redundant processes, businesses can implement targeted strategies that curtail expenditures.
- AI can analyze complex manufacturing data and identify patterns that are impossible normally and supports product design, product planning, and manufacturing ops with insights. Artificial Intelligence (AI) emerges as a pivotal force in manufacturing by unraveling intricate data intricacies that elude conventional analysis. Its prowess in deciphering vast datasets enables the identification of patterns unattainable through manual means. This analytical acumen finds applications across the product lifecycle. During product design, AI dissects consumer behavior, market trends, and performance metrics to inform innovative design choices. In product planning, it optimizes supply chain logistics, production schedules, and resource allocation, minimizing bottlenecks. In manufacturing operations, AI’s real-time data analysis monitors production lines, spotting anomalies and predicting maintenance requirements before disruptions occur. This confluence of AI-driven insights transforms product development, planning, and manufacturing into dynamic, data-powered domains. As industries strive for efficiency and competitiveness, AI becomes an indispensable tool, steering them toward precision and excellence in every phase of the manufacturing journey.
Challenges in implementing AI in manufacturing process:
- Embedding within the manufacturing process requires a cultural shift and change management. Embedding AI within the manufacturing process necessitates a fundamental shift in the organizational culture and a well-orchestrated change management strategy. This transformation is not merely technological but also deeply human. The infusion of AI demands a workforce open to innovation, willing to adapt to new ways of working, and comfortable collaborating with AI systems. Employees need to perceive AI as an aid that amplifies their capabilities, not as a threat to their roles. Change management becomes paramount in facilitating this transition. Communication, training, and fostering a sense of ownership in the AI integration process are essential. Leadership must articulate the benefits of AI in streamlining processes, enhancing quality, and enabling data-driven decisions. Moreover, a culture of continuous learning and experimentation must be fostered, encouraging employees to explore AI’s potential and contribute to its improvement.
- It requires data to be captured across different nodes at granular frequencies and combined with other data. To effectively harness the power of AI in manufacturing, a robust data capture strategy is imperative. This entails collecting data from various points or nodes within the manufacturing process at finely detailed intervals, known as granular frequencies. This data includes information about machine operations, production rates, quality metrics, and more. However, the real potency of AI emerges when this data is aggregated and combined with other relevant datasets. This amalgamation provides a holistic view of the manufacturing ecosystem, enabling AI algorithms to discern intricate patterns, correlations, and anomalies that might otherwise remain hidden. The synergy between granular data and broader datasets empowers AI to offer actionable insights. These insights drive process optimization, predictive maintenance, and informed decision-making. Ultimately, this data-driven approach propels manufacturing operations towards higher efficiency, reduced downtime, and enhanced overall quality, solidifying AI’s role as a transformational force within the industry.
- Reproducibility of the recommendation and business case for the decision is a key challenge for the business to build trust. In the realm of business decisions guided by AI, ensuring the reproducibility of recommendations and the underlying business case is a critical challenge for fostering trust. When AI generates recommendations or supports decisions, stakeholders require confidence that the outcomes can be consistently replicated. This is vital for validating the credibility and accuracy of AI-driven insights. Reproducibility hinges on transparency in AI algorithms and data processing. If the process is opaque, it can breed skepticism and hinder buy-in. Establishing clear documentation of the data used, the algorithms applied, and the logic behind recommendations is essential. A reproducible approach not only builds trust but also aids in troubleshooting and continuous improvement. It allows stakeholders to verify outcomes, identify issues, and refine models or strategies as needed. Ultimately, by addressing the challenge of reproducibility, businesses pave the way for informed decisions, informed adjustments, and a stronger partnership between human expertise and AI capabilities.
Benefits of implementing AI in manufacturing process:
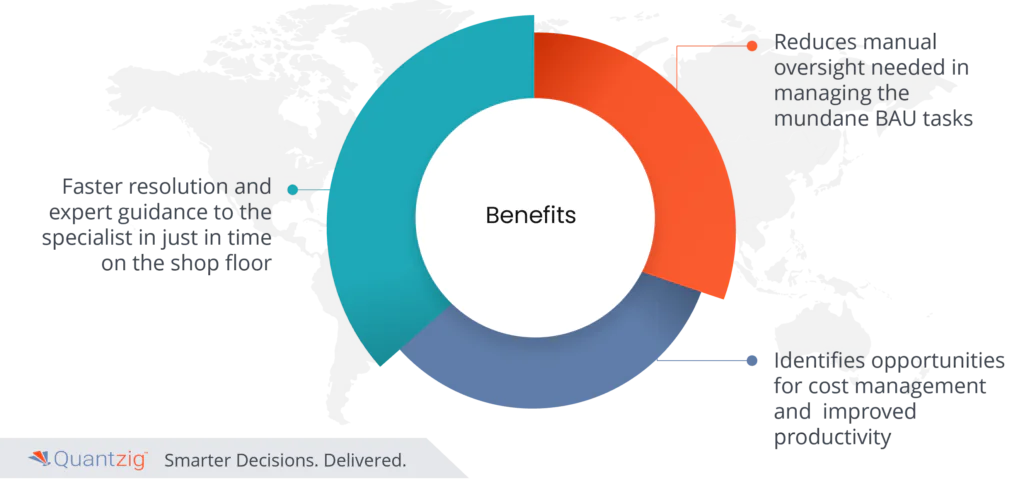
- Faster resolution and expert guidance to the specialist in just in time on the shop floor:
Artificial Intelligence (AI) offers a multitude of benefits in the manufacturing process, notably exemplified by its ability to provide rapid solutions and expert guidance to specialists on the shop floor. Through real-time data analysis, AI identifies issues and bottlenecks promptly, expediting problem-solving and reducing downtime. AI’s predictive maintenance capabilities further amplify these advantages, as it forecasts equipment failures before they occur, enabling preemptive actions. Additionally, AI acts as a virtual expert, offering contextual insights and recommendations to on-site specialists, ensuring informed decision-making in critical moments. The integration of AI augments quality control by spotting deviations and defects in real time, enhancing overall product quality. Furthermore, AI-driven automation optimizes workflows, enhancing efficiency and productivity.
- Reduces manual oversight needed in managing the mundane BAU tasks:
AI’s integration into the manufacturing process brings forth a cascade of advantages, prominently including the reduction of manual oversight required for managing routine Business-As-Usual (BAU) tasks. By automating repetitive and mundane activities, this technology liberates human resources to focus on strategic and value-added initiatives. Through AI-driven process automation, tasks such as data entry, monitoring, and inventory management are streamlined, minimizing errors and accelerating operations. This reduction in manual intervention not only boosts efficiency but also curtails operational costs and enhances overall productivity. Moreover, AI’s ability to learn and adapt over time refines its performance in managing BAU tasks and optimizing processes iteratively. This adaptive capability ensures consistent output and empowers personnel to allocate time and energy to more intricate and creative aspects of manufacturing.
- Identifies opportunities for cost management, improved productivity, etc.:
Through meticulous data analysis, AI discerns patterns, inefficiencies, and areas of improvement within production processes. It illuminates cost-saving prospects by optimizing resource allocation, minimizing waste, and refining supply chain logistics. Its predictive analytics fosters proactive maintenance, averting costly downtime and amplifying productivity. Additionally, real-time monitoring ensures stringent quality control, reduces defects, and enhances overall product excellence. Furthermore, AI-driven insights empower informed decision-making, steering manufacturers toward data-supported strategies. This data-driven approach catalyzes innovation and operational agility, key drivers of growth in the modern manufacturing landscape.
Conclusion:
Going forward, all manufacturers need to keep pace with advanced developments in artificial intelligence to remain viable and competitive in a highly competitive landscape. In this sense, AI is a highly powerful tool enabling manufacturers to stay flexible, successful, and agile in today’s ever-changing business environment. With AI’s prowess in optimizing operations, automating tasks, and uncovering valuable insights, manufacturers are positioned to unlock new levels of efficiency, quality, and innovation. As AI seamlessly integrates predictive analytics and real-time monitoring, it propels production into an era of data-driven excellence. The symbiotic relationship between AI and manufacturing stands as a testament to human ingenuity and technological advancement that promises a future where precision and progress converge for the ultimate benefit of industry and society.
Success Story:
Revolutionizing Manufacturing Operations: How AI-Driven Insights transformed a leading manufacturing company:
Client Details: A prominent player in the manufacturing industry with operations spanning the US and Western Europe grappled with intricate challenges that hampered their efficiency and profitability. They sought to leverage Artificial Intelligence (AI) to revolutionize their operations and stay ahead in the competitive landscape.
Challenges:
The client grappled with intricate challenges due to their globally dispersed manufacturing operations. Balancing minimal inventory stock requirements to curtail storage costs resulted in losses attributed to product obsolescence. The seasonal fluctuation of demand in North America and supply disruptions in Southeast Asia compounded the predicament, straining operational continuity. The absence of real-time insights hindered proactive decision-making in adjusting production and supply strategies. Addressing these issues necessitated a comprehensive approach. Data acquisition across diverse nodes at detailed intervals was crucial, enabling the identification of demand patterns and supply chain fluctuations. Merging this granular data with other relevant information empowered the client to predict demand variations, optimize inventory levels, and streamline manufacturing, thereby mitigating losses and enhancing overall operational resilience.
Solutions:
- At first, we built a multi-echelon-based recommendation solution to identify the exact amount of inventory required at each warehouse for each SKU. This data-driven approach optimized inventory allocation and minimized overstocking and stockouts. By aligning stock levels with demand patterns, the client significantly reduced holding costs and avoided missed sales opportunities. The solution fostered efficient resource allocation by enhancing operational agility and profitability.
- The integration of early warning alerts based on on-shelf availability for SKUs proved immensely beneficial to the client. By promptly identifying low stock levels and potential shortages, the client could initiate swift replenishment actions. This preemptive approach minimized disruptions in the supply chain and ensured consistent product availability. Customer satisfaction improved as stockouts decreased, leading to enhanced brand trust. The alerts bolstered operational efficiency, enabling the client to proactively address inventory shortfalls, optimize replenishment strategies, and maintain uninterrupted sales flow.
- Finally, our team devised translocation strategies for unmoving inventory between locations. The implementation of translocation strategies for stagnant inventory yielded substantial advantages for the client. By reallocating unmoving inventory from one location to another, the client could optimize stock levels and mitigate losses due to obsolescence. This dynamic approach streamlined inventory management, freeing up valuable storage space and reducing carrying costs. The strategy enhanced operational efficiency, preventing resources from being tied up in non-performing stock. This reallocation not only minimized financial impact but also improved overall inventory turnover rates, contributing to cost savings, and maximizing the client’s utilization of resources.
Impact Delivered:
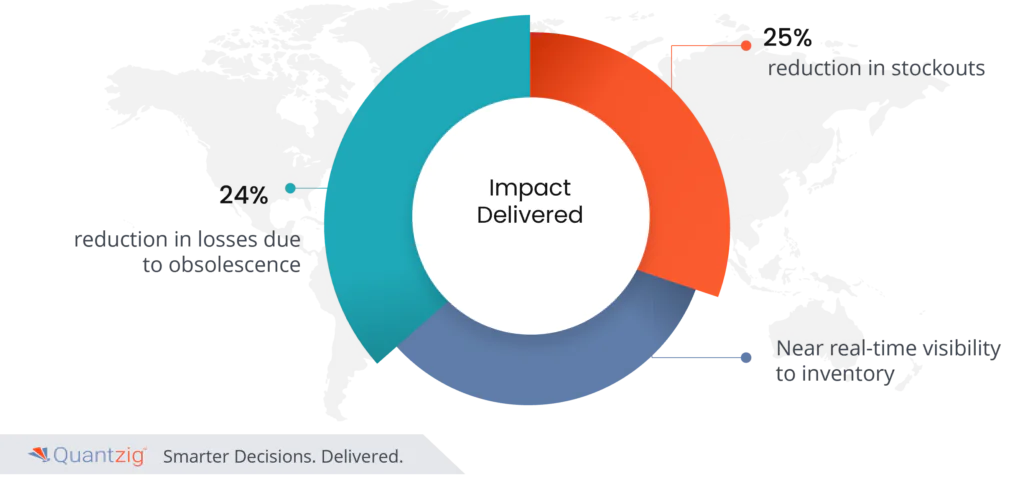
- 24% reduction in losses due to obsolescence.
- 25% reduction in stockouts
- Near real-time visibility to inventory
Looking to revolutionize your manufacturing operations through AI-driven insights? Contact Quantzig today to explore how our expertise can transform your challenges into opportunities for growth and success.