Data is important to how businesses operate and function. B2B businesses should make sense of data and find relevance in the noise that’s made by diverse technologies and systems supporting today’s highly connected digital global economies. In this regard, data becomes paramount. However, data alone is useless- B2B businesses require a robust enterprise data strategy, data management models, and governance to integrate all types of data for efficient and practical use across employee networks, supply chains, and customer and partner ecosystems. This comprehensive guide will help you understand how your businesses can effectively organize, store, and analyze their data to drive decision-making and enhance operational efficiency.
Book a demo to experience the meaningful insights we derive from data through our analytical tools and platform capabilities. Schedule a demo today!
Request a DemoTable of Contents
What are Data Management Solutions
This data management process integrates the practice of collecting, organizing, managing, and accessing data to support efficiency, decision-making, and productivity. Given the pivotal role data plays in today’s global business, an accurate data management platform and an effective enterprise data strategy are crucial for every B2B business regardless of industry or size. It is essential for a wide range of data-driven use cases, including regulatory compliance, end-to-end business process execution, data migration, accurate analytics and AI, and digital transformation.
Key Features of Data Management Solutions
01
- Data Integration and Connectivity
- Connects data from various sources—cloud, on-premises, or hybrid—into a unified system, enabling consistent, real-time access across the organization.
02
- Data Quality and Governance
- Ensures accuracy and consistency through cleansing, validation, and enrichment tools, while enforcing data policies and roles to maintain control and compliance
03
- Scalability and Performance
- Designed to handle growing data volumes efficiently, these solutions maintain high performance and support diverse data types without compromising speed.
04
- Security and Compliance
- Protects sensitive information with encryption, user access controls, and audit capabilities, while supporting adherence to regulations like GDPR and HIPAA.
05
- Advanced Analytics and AI Readiness
- Enables seamless data preparation and access for analytics, machine learning, and AI, helping businesses turn data into actionable insights faster.
Data Integration and Connectivity
These solutions enable seamless integration across multiple data sources—cloud-based, on-premises, or hybrid—ensuring data flows smoothly between systems, departments, and tools. This connectivity allows for real-time data access and a unified view of business information.
Data Quality and Governance
High-quality data is non-negotiable. Advanced solutions come equipped with tools for data cleansing, validation, deduplication, and enrichment, along with built-in governance frameworks to enforce policies, roles, and accountability for data usage and access.
Scalability and Performance
Whether handling terabytes or petabytes of data, robust data management platforms are built to scale with business growth. They maintain performance and responsiveness even as data volumes increase, supporting both structured and unstructured data types.
Security and Compliance
Enterprise-grade platforms offer robust security features like encryption, access control, and audit trails. They also support compliance with global data regulations (e.g., GDPR, HIPAA, CCPA) through automated policy enforcement and data lifecycle management.
Advanced Analytics and AI Readiness
Modern data management systems are designed to support advanced analytics, machine learning, and artificial intelligence. They enable data scientists and analysts to easily access, prepare, and analyze data, accelerating innovation and strategic insight.
Importance of Effective Data Management Solutions
In an increasingly data-driven world, effective data management ensures businesses can trust, access, and utilize their data efficiently. It supports informed decision-making, operational efficiency, compliance, and innovation across all functions.
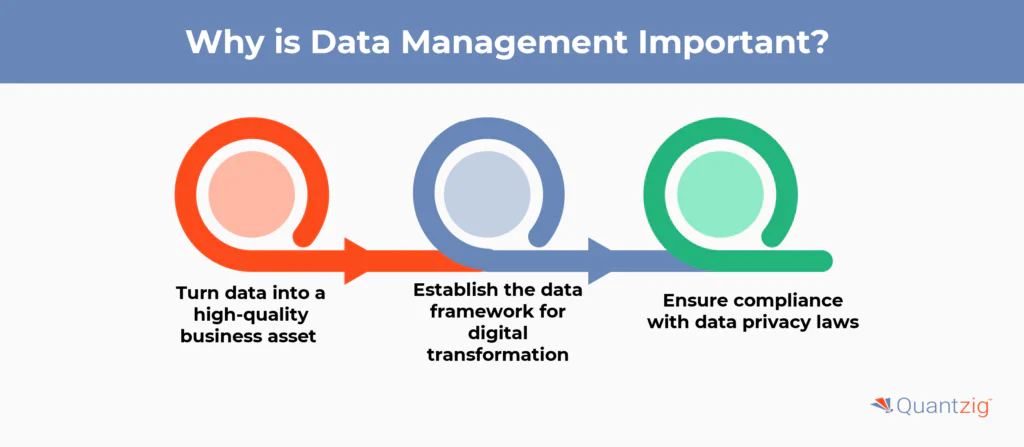
Advantages
Improved Decision-Making
Reliable data enables leadership to make faster, data-backed decisions, minimizing risks and maximizing opportunities based on real-time insights and historical trends.
Regulatory Compliance
Helps businesses meet regulatory requirements by organizing data properly, maintaining audit trails, and automating compliance reporting, reducing legal and financial risk.
Operational Efficiency
Streamlined access and reduced data duplication lower manual workloads, speed up workflows, and enhance productivity across departments.
Enhanced Customer Experience
Clean, accurate customer data allows for personalized interactions, timely service, and better customer satisfaction across marketing, sales, and support channels.
Scalability and Growth Support
Robust systems can grow with the business, easily handling increased data volume and complexity without sacrificing performance or data integrity.
Disadvantages
High Implementation Costs
Initial setup of enterprise-grade solutions can be costly, involving licensing, infrastructure, training, and integration with legacy systems.
Complex Integration Challenges
Connecting data from multiple systems, especially legacy or third-party tools, can be complex and time-consuming, requiring skilled resources.
Maintenance and Upkeep
Continuous updates, data governance, and system monitoring require ongoing effort and investment to keep the platform effective and secure.
Data Security Risks
Despite safeguards, large centralized data systems can become prime targets for breaches if not managed with strong security practices.
Change Management Resistance
Employees may resist adopting new processes or technologies, making successful implementation dependent on training, communication, and leadership support.
Strategies to Implement Effective Data Management Solutions
Develop a Clear Data Strategy
Start with a well-defined data management strategy aligned to business goals. Identify data needs, key stakeholders, and long-term objectives. Outline how data will be collected, stored, accessed, and governed. A strong strategy ensures consistency across teams, reduces redundancy, and provides a framework for measuring data performance and ROI over time. Involve leadership and prioritize cross-functional collaboration from the beginning for better adoption and clarity.
Invest in Scalable and Flexible Technology
Choose data management platforms that can grow with your business and support both structured and unstructured data. Look for cloud-native or hybrid solutions with open architecture for seamless integration. Scalable systems reduce the need for frequent upgrades and support emerging needs like AI, analytics, or real-time processing. Flexibility also ensures smooth adoption of future tools and technologies without major system overhauls.
Establish Strong Data Governance
Implement governance policies that define data ownership, access rights, data standards, and usage protocols. A governance framework ensures data is accurate, consistent, and secure throughout its lifecycle. Assign data stewards or governance leads to monitor compliance and resolve data quality issues. Clear governance helps reduce risk, supports compliance requirements, and builds trust across the organization in the data being used for decision-making.
Prioritize Data Quality Management
Regularly monitor, clean, and enrich your data to ensure it’s accurate, up-to-date, and usable. Implement tools and processes to automate data validation, deduplication, and error correction. Encourage teams to flag data inconsistencies and empower data owners to take corrective actions. High data quality enhances analytics, improves operational efficiency, and ensures reliable outcomes in reporting, forecasting, and AI initiatives.
Train Teams and Promote Data Literacy
Empower employees by providing training on data tools, policies, and best practices. Promote a culture of data literacy so that everyone—from entry-level staff to executives—can understand, interpret, and use data effectively. Offer regular workshops, resources, and support to increase adoption and reduce resistance. A data-literate workforce is essential for maximizing the value of your data and making informed business decisions.
Applications of Data Management Solutions
Data management solutions support business intelligence by integrating and organizing data from various sources. This enables companies to create accurate reports, dashboards, and visualizations for faster, more informed decision-making. By providing a single source of truth, they help identify trends, uncover insights, and forecast future outcomes, which boosts performance across departments such as finance, marketing, operations, and executive leadership.
These solutions are essential for maintaining regulatory compliance and minimizing risk. They provide tools for data classification, access control, and audit logging, ensuring businesses meet standards like GDPR, HIPAA, and CCPA. With built-in governance and security, organizations can manage sensitive data responsibly, automate compliance tasks, and respond quickly to legal or regulatory inquiries with confidence and accuracy.
In customer-facing applications, data management improves the quality and personalization of engagement. By unifying customer data from sales, marketing, and support systems, businesses can deliver consistent and relevant experiences. Clean, accessible data reduces errors, enhances service delivery, and supports targeted campaigns, ultimately strengthening customer relationships and loyalty over time.
Experience the advantages firsthand by testing a customized complimentary pilot designed to address your specific requirements. Pilot studies are non-committal in nature.
Start your trial nowWhat the Future Holds for Data Management Solutions
The future of data management is increasingly intelligent and automated. With the rise of artificial intelligence, machine learning, and real-time analytics, data systems will evolve to be more proactive—automatically identifying patterns, correcting errors, and recommending actions. This shift will reduce manual effort and allow businesses to react faster to market changes, customer behavior, and operational challenges, all while maintaining higher levels of accuracy and efficiency.
As data environments grow more complex with multi-cloud, edge computing, and IoT integrations, future data management solutions will prioritize adaptability and resilience. Concepts like data fabric and unified data governance will become standard, enabling seamless data access and control across diverse platforms. Organizations that embrace these innovations will unlock greater agility, foster innovation, and gain a competitive edge in increasingly data-driven industries.
Conclusion:
We understand that information is extracted from data. And if information is power, then skillfully managing and profiting from your data could potentially be your company’s greatest asset. Consequently, data management responsibilities are progressing to become crucial catalysts for change within the organization – in leveraging emerging data management trends and technologies, promoting cloud migration, and providing strategic value to the business.
Get started with your complimentary trial today and delve into our platform without any obligations. Explore our wide range of customized, consumption driven analytical solutions services built across the analytical maturity levels.
Start your Trial