Written By: Associate Vice President, Analytics and Data Strategy, Quantzig.
Table of Contents
Introduction to Cloud Data Strategy
While most organizations understand that their business data is a strategic asset, many are not taking complete benefits of it to get ahead. Even though organizations are investing more than ever in data and analytics initiatives, long-standing challenges like fragmented and unreliable data, inefficient data management approaches, and a shortage of impactful insights continue to hinder the realization of your data’s full potential.
Implementing a robust data strategy framework has been shown to help companies overcome these hurdles and define the path towards becoming more data-driven. In this blog, we’ll be discussing the major elements of an effective robust data and cloud strategy that will help you make data-driven business decisions depending on data analysis rather than intuition.
Book a demo to experience the meaningful insights we derive from data through our analytical tools and platform capabilities. Schedule a demo today!
Request a Free DemoWhat is Cloud Data Strategy?
Data in today’s businesses is crucial for planning, optimizing operations, and making strategic decisions. Companies must establish effective ways to collect, store, access, analyze, and govern their data. This involves developing a comprehensive data strategy that encompasses data management, data analysis, ‘ data Architecture, data pipelines, data governance, data compliance, data democratization, and data virtualization. The data strategy should align with overall business goals and objectives. It’s important to define key performance indicators to measure progress.
A data strategy serves as a roadmap, outlining an organization’s long-term vision for collecting, storing, sharing, and utilizing its data. By having a clear and concise data strategy in place, businesses can streamline their data-related processes, making it easier for everyone who needs access to the data to work with it effectively throughout the data journey.
Moreover, a robust data strategy is essential for organizations looking to leverage advanced technologies such as machine learning (ML) and generative artificial intelligence (AI). These cutting-edge tools rely on high-quality, well-structured data to deliver accurate insights and predictions. By aligning their data strategy with their ML and AI initiatives, organizations can ensure that they have the necessary data infrastructure and governance in place to support these technologies.
Why Cloud Data Strategy Matters: 3 Important Pillars
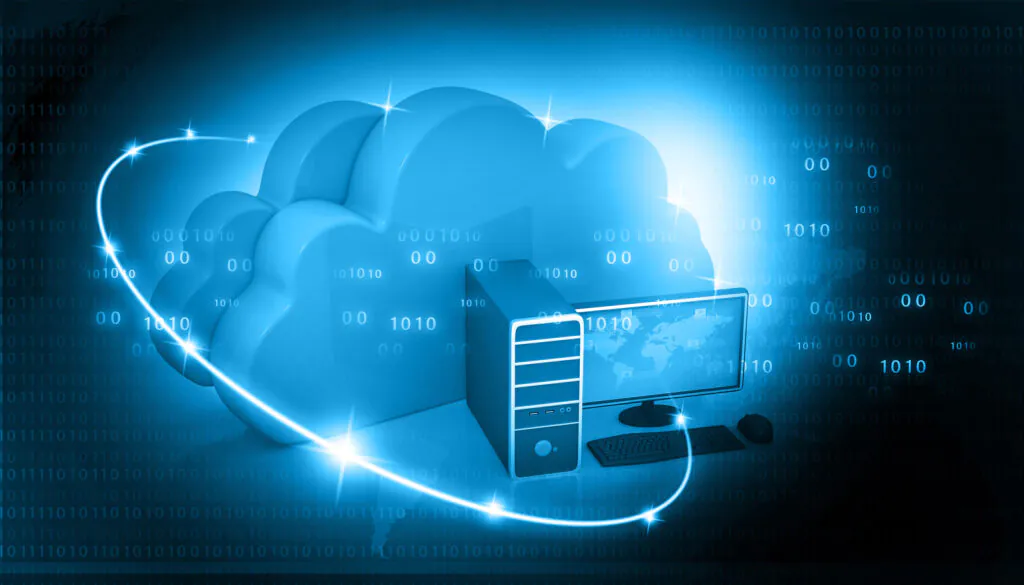
A cloud data strategy is an organized plan that enables organizations to effectively manage and utilize data within a cloud migration environment. This strategy involves decisions on data collection, storage, security, analysis, and overall utilization within the realm of cloud computing, aiming to enhance data accessibility, scalability, reliability, and cost-effectiveness. It typically incorporates cloud-based technologies and services such as data lakes, databases, data maturity evaluation, analytics strategy tools, business strategy, and machine learning. A well-crafted cloud data strategy is crucial for unlocking the complete transformation of an organization’s data assets, facilitating improved decision-making, data maturity evaluation, and fostering innovation in a dynamic business landscape.
1. Data Experiences
Providing productive user experiences that enable all users to access and create value from relevant data is crucial. To achieve this, organizations should:
- Establish a data university to advance data literacy for everyone in the organization
- Pivot from role-oriented to product-oriented structures with cross-functional teams
- Define clear data principles that align with organizational priorities and guide decision-making
2. Data Economy
Implementing principles and practices to ensure that data can be published, discovered, built upon, and relied on is essential. Key steps include:
- Staffing a data platform team dedicated to enhancing the developer and analyst experience
- Prioritizing the integration of external and enterprise source data, such as customer, transaction, and product data
- Fostering data sharing as data products among teams that depend on each other
- Implementing foundational data governance practices, including stewardship, discovery, quality, reliability, and privacy
3. Data Ecosystem
Establishing a unified, open, and intelligent platform with end-to-end data capabilities for all users and needs is the final pillar. Organizations should:
- Choose a data ecosystem that provides comprehensive capabilities out of the box, with open standards to integrate other components as needed
- Develop a plan to enable everyone in the organization to apply AI in their work using the capabilities in the ecosystem
- Minimize operational overhead by selecting serverless tools for the capabilities in the data ecosystem
By focusing on these three pillars – data experiences, data economy, and data ecosystem – organizations can build a modern data strategy that empowers users, fosters collaboration, and drives innovation through data-driven decision-making.
Creating an Effective Data Strategy Roadmap
Step 1: Assess Current Data Maturity (0-3 months)
- Conduct a thorough audit of existing data assets, systems, and processes
- Identify strengths, weaknesses, and areas for improvement
- Establish a baseline for current data maturity
Step 2: Define Strategic Goals (3-6 months)
- Align data strategy with overall business objectives and priorities
- Identify key use cases and potential value drivers
- Set measurable goals and KPIs to track progress
Step 3: Implement Data Governance (6-12 months)
- Establish clear roles, responsibilities, and decision-making processes
- Develop data policies, standards, and guidelines
- Implement data quality management processes
Step 4: Enhance Data Architecture (12-18 months)
- Modernize data infrastructure and adopt cloud-based solutions
- Implement data catalog tools for asset discovery and metadata management
- Integrate data management tools for data integration, visualization, and reporting
Step 5: Leverage Advanced Analytics (18-24 months)
- Develop advanced analytics capabilities, including ML and AI
- Ensure ethical and responsible use of data and analytics
- Upskill employees in data literacy and data science
Step 6: Continuously Optimize and Evolve (ongoing)
- Regularly review and assess data strategy effectiveness
- Adapt to changing business needs and emerging technologies
- Foster a data-driven culture and promote data-informed decision making
By following this roadmap and incorporating the key components of an effective data strategy, organizations can unlock the full potential of their data assets, drive innovation, and achieve sustainable competitive advantage.
Why is Manufacturing Industry Implementing Data and Cloud Strategy?
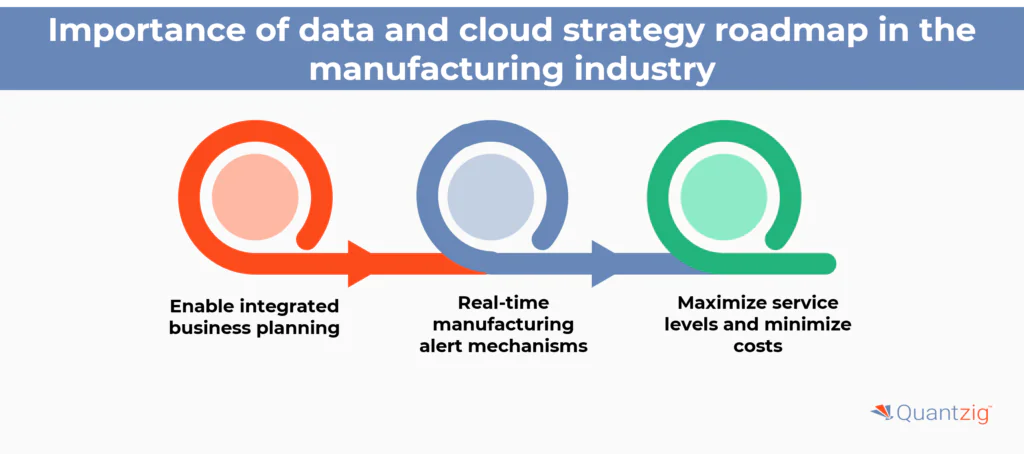
1. Enable integrated business planning:
The outbreak of the COVID-19 pandemic and its long-lasting impacts exposed numerous gaps in business planning. Many manufacturing companies have been continuously trying to strengthen their existing business value planning capabilities with decidedly mixed results. Business and function units often continue to plan in silos, but the assumptions behind most of the planning business strategy forecasts still aren’t transparent.
A well-crafted data strategy framework strategy facilitates smooth data integration from diverse systems and sources, providing a consolidated view of information. This capability enables the manufacturing industry to eliminate data silos and gain comprehensive insights for strategic planning and informed decision-making. Besides, cloud technology ensures seamless data accessibility, transformation, collaboration, and scalability that enhance the efficiency of integrated business planning across geographically dispersed units.
2. Real-time manufacturing alert mechanisms:
Visibility is the key advantage that manufacturers get from manufacturing data collection. By analyzing real-time data, or “shop floor data,” manufacturers can better understand how to comprehend, assess, and improve their existing plant operations. It’s important to define key performance indicators to measure progress. After that, they can make more informed decisions based on detailed real-time data. This is why having real-time, precise production data for manufacturers is critical.
The integration of a data and cloud strategy in the manufacturing sector paves the way for real-time alert mechanisms that revolutionize operational responsiveness. By harnessing the power of data analytics and ai, manufacturers can monitor critical production variables in real-time, and detect anomalies and deviations instantly.
Cloud technology acts as the conduit that swiftly transmits this data to centralized platforms accessible by stakeholders across the organizations. This synergy enables the creation of automated alert systems that trigger notifications for potential issues or opportunities. Whether it’s machine malfunctions, quality deviations, or supply chain disruptions, these mechanisms empower manufacturing data analytics teams to swift corrective actions, minimize downtime, and enhance efficiency.
3. Maximize service levels and minimize costs:
Integrating data and cloud strategy services in manufacturing optimizes service levels and minimizes costs. This involves developing a comprehensive data strategy that encompasses data management, data analysis, data Architecture, data pipelines, data governance, data compliance, data democratization, and data virtualization. As cloud technology enables real-time data access, innovation, and collaboration across the supply chain, the manufacturers can minimize stockouts, reduce excess inventory, and streamline operations.
A data and cloud strategy provides cost savings to manufacturing organizations through pay-as-you-go pricing models and effective resource use. Besides, manufacturers can take benefit of multiple cloud providers and ai while minimizing expenses by choosing the most appropriate cloud services for their specific needs. The result is a harmonious balance between exceptional service and cost-effectiveness, that empowers companies to thrive in a competitive market landscape.
Experience the advantages firsthand by testing a customized complimentary pilot designed to address your specific requirements. Pilot studies are non-committal in nature.
Request a Free PilotDifferent approaches to Implementing Data Strategy
Creating a data strategy for an organization involves adopting either a centralized or decentralized approach, or a combination of both. Here are the key aspects of each approach:
Centralized Approach
A centralized approach to data management emphasizes control and a single source of truth for broad data categories. This approach involves:
- Single Source of Truth: A single repository for all data, ensuring consistency and integrity.
- Data Defense: Identifying, standardizing, and governing authoritative data sources to prevent data corruption and ensure compliance.
- Prioritized Activities: Focus on compliance, fraud detection, and security measures to protect the data.
Decentralized Approach
A decentralized approach recognizes that different business units may interpret data differently. It accommodates these differences by allowing controlled data transformations that can be mapped back to the single source of truth. This approach is suitable for real-time operations and customer-focused functions like marketing and sales.
Balancing Centralized and Decentralized Approaches
To achieve a successful data strategy, organizations should strike a balance between centralized and decentralized approaches. Centralized approaches are more relevant for legal, financial, compliance, and IT departments, while decentralized approaches are better suited for real-time operations and customer-focused functions. A balanced strategy provides the flexibility to adapt the single source of truth to meet business needs effectively.
Key benefits of data and cloud strategy roadmap in the manufacturing industry:
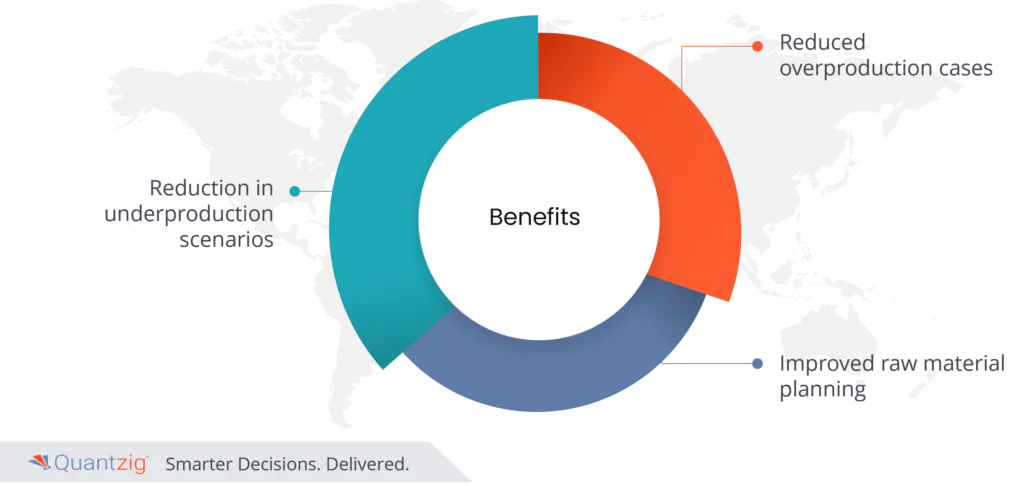
1. Reduction in underproduction scenarios:
A hybrid data and cloud strategy in manufacturing mitigates underproduction scenarios by leveraging data analytics for accurate demand forecasting and real-time monitoring. As cloud technology facilitates rapid data processing and sharing, it becomes easy to swift responses to demand fluctuations. With an accurate cloud strategy, manufacturers gain insights into market trends and customer preferences that help them to optimize their production planning.
2. Reduced overproduction cases:
Cloud-based hybrid platforms enable the manufacturing industry to share real-time data across various departments. This facilitates better coordination between production, inventory, and sales management teams and prevents excessive production based on outdated information. You can get accurate demand forecasting through cloud-based data analytics that will help you to align production with actual market requirements. Real-time production monitoring process through IoT devices connected to the cloud helps the manufacturing team to detect the production bottlenecks and inefficiencies that prevent overproduction due to unexpected delays.
3. Improved raw material planning:
Materials planning is the process of controlling, scheduling, and organizing the flow of raw materials within the organizations to ensure that the right materials are available at the right place, time, and quantity to fulfill the customer and production demands efficiently. This process involves coordinating each activity of production, procurement, and distribution. Cloud technology serves as the backbone that facilitates the storage, processing, and sharing of vast datasets across departments and supply chain partners. Real-time data sharing with cloud adoption strategies ensures that information is up-to-date and reduces the risks of over-ordering and under-ordering materials. As suppliers maintain real-time communication through cloud platforms, they make responsive adjustments to orders based on changing production needs.
Get started with your complimentary trial today and delve into our platform without any obligations. Explore our wide range of customized, consumption driven analytical solutions services built across the analytical maturity levels.
Start your Free TrialHow Quantzig Helps you to plan your cloud data strategy?
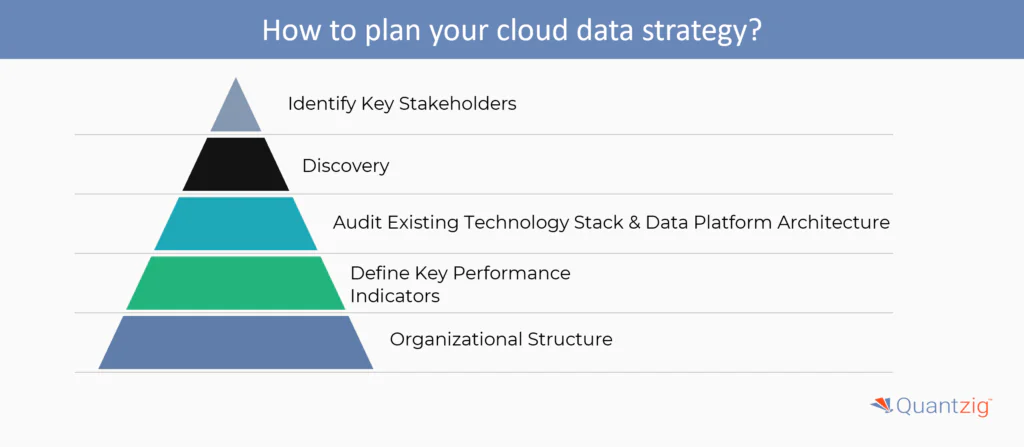
Organizations can position themselves for success in the digital age, lay the groundwork for efficient data management, and match data initiatives with business objectives by implementing the actions listed below.
1. Identify Key Stakeholders
Integrating the distinct perspectives and knowledge of your stakeholders into business procedures is essential to a successful data strategy roadmap. They get a sense of ownership from this, which boosts stakeholder empowerment and raises the possibility that the data strategy will be successfully implemented and adopted.
2. Discovery
When the organization’s data objectives are well understood, it is easier to develop a targeted data strategy that adds value and satisfies business needs. To reduce uncertainty and foster trust in data, for example, an organization can decide which data lineage, metadata management, and data catalog tools will be used.
Alternatively, they can decide to create a centralized, unified data ecosystem that will do away with silos and enhance team communication within. To gain deeper insights from their data, businesses can also include a data analytics strategy in their overall data strategy. It establishes the framework for investigating data as a strategic asset and enabling decision-making procedures consistent with the organizational strategy.
3. Audit Existing Technology Stack & Data Platform Architecture
In this phase, companies carry out evaluations to determine the efficacy and capabilities of their current technology stack, which includes storage technology, data architecture, and data management components. Data integration and migration capabilities must be identified to enable smooth data flow between systems. Infrastructure factors including scalability, compatibility, security, and usability are also considered. This evaluation ensures that teams can grow the stack in accordance with the organization’s data strategy and are aware of the current stack’s costs and capabilities.
4. Define Key Performance Indicators
Key performance indicators (KPIs) are critical for providing analytical answers when assessing how well an organization is managing its data. They could be numerical or qualitative. KPIs are used as benchmarks to evaluate the success and advancement of data-related projects. Businesses can pinpoint many areas that need improvement, including data integration, quality, storage, and delivery. Then, they can establish pertinent KPIs that support their corporate goals.
5. Organizational Structure
Creating a team structure that defines roles and responsibilities and encourages cross-functional collaboration is the task at hand. A chief data officer, chief information officer, or chief technology officer (CDO, CIO, or CTO) leads the teams of various data-specific roles (e.g., solution architects, implementation architects, data engineers, data scientists, DevOps engineers, etc.) in a typical team structure. By establishing these specialized data teams and filling any skill gaps, organizations can enhance collaboration and knowledge sharing by having these teams capable of executing short sprint cycles with achievable milestones. This enables them to make strategic plans, test ideas, and progressively scale up projects for optimal effect.
Quantzig’s Cloud Data Strategy Solutions for a Manufacturing Company
Category | Details |
---|---|
Client Details | A leading manufacturing company headquartered in Germany. |
Challenges Faced by the Client | Raw material overlooked in production planning, the capacity plan was not in line with manufacturing demand, and low asset utilization and poor production scheduling. |
Solutions Offered by Quantzig | Quantzig’s solutions included to integrate cloud data warehouse for comprehensive planning, cloud-based mini-ERP for integrated production planning, and machine learning-driven production and raw material recommendations module. |
Impact Delivered | 100% reduction in under-production scenarios 20 percentage points improvement in asset utilization 3Mn cost savings due to a reduction in inventory wastage |
The client experienced several adverse effects due to inadequate production planning, particularly in terms of raw material oversight. This oversight led to frequent disruptions in production schedules, causing delays in fulfilling customer orders and an excess of inventory when raw materials were overestimated. Consequently, the client faced elevated carrying costs, diminished cash flow, and an increased risk of material obsolescence. Furthermore, frequent changes in orders strained relationships with suppliers. Additionally, a misaligned capacity plan hindered the client’s ability to meet market demand effectively, resulting in production bottlenecks, delayed order fulfillment, dissatisfied customers, and inefficient resource utilization. These inefficiencies elevated operating costs and wasted labor, leading to higher expenses and a competitive disadvantage.
Compounding these issues were low asset utilization and poor production scheduling, which further increased costs, strained client relationships, and decreased staff morale. The client missed revenue opportunities due to an inability to promptly address market demands, thereby decreasing competitiveness. To achieve operational excellence and maintain a competitive edge, the client needed to address these challenges with effective scheduling and optimized asset utilization. Quantzig’s solutions provided the necessary interventions to transform the client’s production planning and capacity management, driving significant improvements in efficiency and market responsivenes
Impact Delivered:
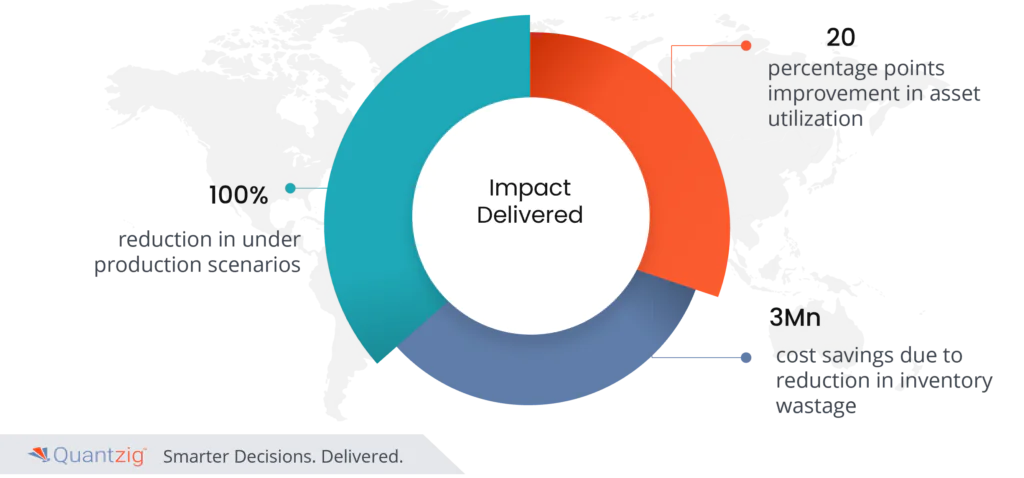
- 100% reduction in under-production scenarios
- 20 percentage points improvement in asset utilization
- 3Mn cost savings due to a reduction in inventory wastage
Conclusion:
In summary, this article delves into the key significance of the data and cloud strategy roadmap, implementation strategies, advantages, and real-world applications in manufacturing sectors. The fusion of data and the cloud not only amplifies real-time insights and data-driven decisions but also optimizes resource allocation, minimizes risks, and fosters agile collaborations across functions. This involves developing a comprehensive data strategy that encompasses data management, data analysis, data Architecture, data pipelines, data governance, data compliance, data democratization, and data virtualization.
This harmonious cloud data strategy framework not only bolsters service levels and cost-effectiveness but also positions manufacturers as pioneers in an evolving industry. As manufacturing continues to evolve in the digital age, this strategic convergence of data and cloud strategies serves as a guiding light, steering manufacturing enterprises toward sustained growth, competitiveness, and resilience.