Written by: Sudeshna Ghosh
Table of Contents
Introduction to Data Governance Strategy
Data governance is the systematic procedure of managing the usability, integrity, availability, and security of the enterprise data, based on internal policies and standards that also control data usage. The entire processes determine data security measures, data owners, and intended data uses. The purpose of effective data governance and data quality strategy is to properly maintain high data quality that’s both easily accessible and secure for deeper insights.
What does Data Governance Strategy mean?
A data governance strategy serves as a comprehensive blueprint that establishes the objectives, principles, and direction for managing data assets within an organization. This strategic plan guides decision-making processes and resource allocation, ensuring that data is treated as a valuable corporate resource.
Key Elements of a Successful Data Governance Strategy
Implement a data governance framework aligned with organizational goals and industry standards. Define roles and responsibilities for data stewardship and establish policies and procedures for data management. Assess the organization’s size and complexity to determine the appropriate level of governance. Choose between enterprise-wide or iterative approaches based on business needs. Develop a roadmap with clear steps, priorities, timelines, and metrics for success.
By incorporating these key elements into the data governance strategy, organizations can effectively manage their data assets, ensure data quality, and leverage data as a strategic resource to drive business value and achieve their objectives.
Book a demo to experience the meaningful insights we derive from data through our analytical tools and platform capabilities. Schedule a demo today!
Request a Free DemoQuantzig’s Data Governance Solution for a Leading Retail Brand
Client Details | A leading retail and e-commerce giant operating business in 22 global markets. |
Challenges Faced by the Client | Latency to react to data issues, highly manual resolution, and the cascading effect of BI tools. |
Solutions Offered by Quantzig | Quantzig’s solutions included self-healing data governance systems, ML-based data quality management, and visual workflow management apps. |
Impact Delivered | 80% reduction in time to resolve a data issue,10x lesser manual intervention, and 25% improvement in ROI. |
Challenges Faced by the Client
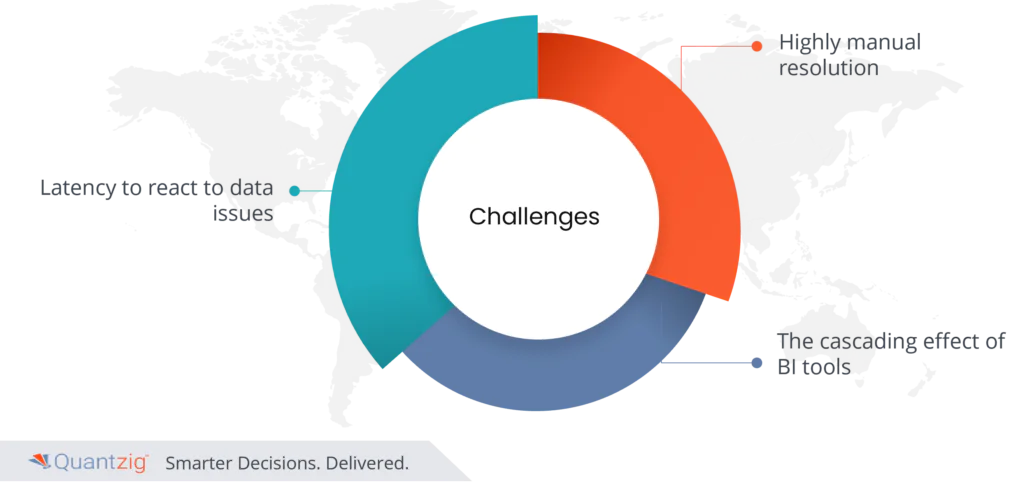
The client experienced significant delays in addressing data issues due to scattered data and lack of governance. Manual problem resolution consumed resources and hindered operational efficiency. Proliferation of BI tools led to a complex data landscape with siloed data and inconsistent reporting. Standardized practices were absent, impacting data accuracy and compliance, and complicating decision-making.
Solutions Offered by Quantzig
1. Self-healing data governance systems:
Quantzig designed an innovative self-healing data quality and data governance framework that automated data validation, cleansing, and reconciliation processes. Integrating machine learning algorithms and advanced analytics, the system proactively detected and rectified data issues, and ensured continuous data accuracy and reliability.
With real-time data monitoring and automated alerts, the client gained in-depth data insights, facilitated faster issue resolution, and enhanced data-driven decision-making. This transformative solution from Quantzig resulted in enhanced productivity, streamlined data management, a data-centric culture, and positioned the client for sustained growth and success.
2. ML-based data quality management:
Implementing ML-based data quality management proved to be a game-changer for the client, spearheaded by innovative solutions from Quantzig. Our team harnessed the power of Machine Learning algorithms to automatically identify and rectify data anomalies and ensured data accuracy and reliability. The ML-driven approach enabled the client to proactively detect potential data issues, reduce manual efforts in data validation and cleansing.
Real-time data monitoring and predictive analytics capabilities facilitated quick identification of data quality deviations, allowing the client to take corrective actions promptly. As a result, they experienced streamlined processes, improved data integrity, and fortified decision-making, driving their business toward greater efficiency and competitive advantage.
3. Visual workflow management apps:
Visual workflow management apps proved to be a transformative solution for the client, revolutionizing their business processes with the assistance of cutting-edge technologies. Prior to adopting these apps, the client struggled with manual, time-consuming workflows that led to inefficiencies and bottlenecks. The introduction of visual workflow management apps streamlined their operations by providing a user-friendly interface that allowed for easy visualization and optimization of processes.
The apps facilitated seamless collaboration, automated task allocation, and real-time progress tracking, boosting productivity and accountability. With clear insights into workflow performance, the client gained the ability to identify and address inefficiencies promptly, resulting in improved operational efficiency, reduced turnaround times, and enhanced overall business performance.
Impact Delivered
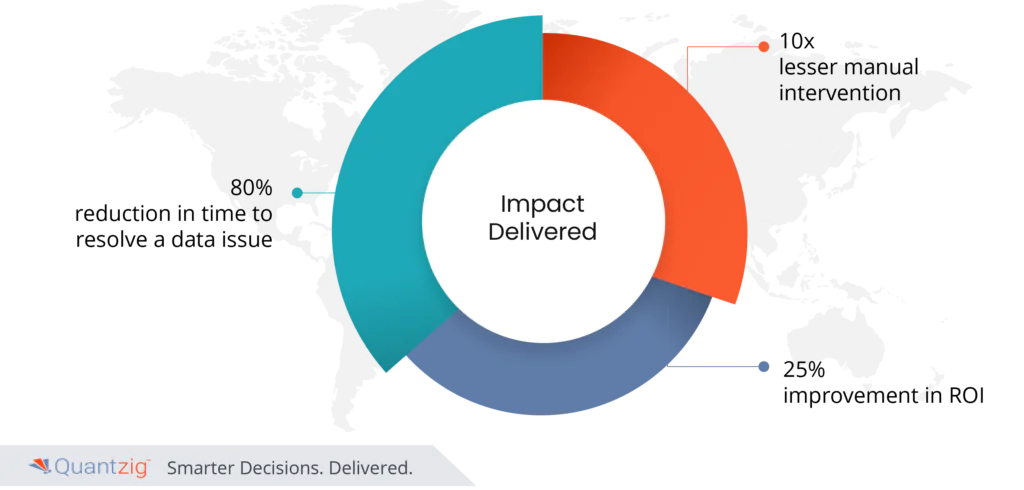
- 80% reduction in time to resolve a data issue
- 10x lesser manual intervention
- 25% improvement in ROI
Get started with your complimentary trial today and delve into our platform without any obligations. Explore our wide range of customized, consumption driven analytical solutions services built across the analytical maturity levels.
Start your Free Trial TodayBenefits of an Effective Data Governance Strategy
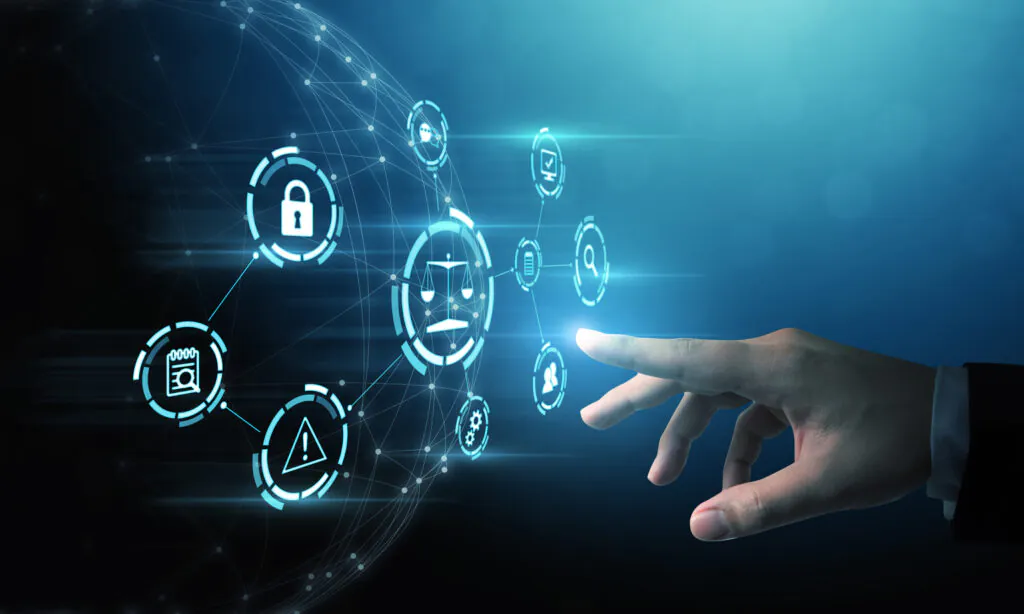
An effective data governance strategy offers numerous benefits, including enhanced data security through stringent data security measures and robust data storage protocols. It ensures data accuracy, data integrity, and data consistency by implementing comprehensive data standards and master data management practices. Assigning data owners and forming dedicated data management teams promotes accountability and data literacy across the organization.
This strategy supports compliance with regulatory policies, facilitates efficient data classification, data mapping, and data integration. Moreover, it leverages generative AI models to derive actionable insights, thereby optimizing the overall data management strategy and driving informed decision-making.
1. Enable enterprise scaleup and deployment:
It enables enterprise scaleup and deployment by providing a structured framework for managing data assets across the organization. It ensures that data is accurate, consistent, and secure, allowing for confident decision-making and improved operational efficiency. By establishing clear data ownership, accountability, and access controls, this technique minimizes the risk of data breaches and regulatory non-compliance.
Furthermore, it facilitates seamless collaboration between different departments and business units, fostering a culture of data-driven insights. Ultimately, this strategy empowers enterprises to harness the full potential of their data, optimize processes, enhance customer experiences, and gain a competitive edge in the ever-evolving business landscape.
2. Reduced Manual Dependency:
This approach leads to reduced manual dependency by streamlining data processes and ensuring data accuracy. With clearly defined data quality standards and automated data validation mechanisms in place, organizations can trust their data, reducing the need for extensive manual data verification and correction.
This technique also establishes data access controls and permissions, minimizing the risk of unauthorized access and manipulation, thus reducing manual interventions for data security. Additionally, by implementing best practices, organizations can eliminate redundant data handling and enhance data integration, enabling seamless data flow and quicker decision-making. This reduction in manual efforts optimizes resource allocation, enhances productivity, and allows teams to focus on more strategic tasks, leading to improved overall efficiency and business outcomes.
3. Faster issue resolution time:
Self-healing data quality management systems leverage automated processes to identify and resolve complex data issues and lead to faster resolution times. When aligned with an effective strategy, it contributes to faster issue resolution time by providing a well-organized and accurate data infrastructure. With clear data definitions, standardized data formats, and established data quality controls, data governance ensures data consistency and reliability.
This leads to quicker identification of data-related issues and enables data teams to address them promptly. Moreover, this approach facilitates better data documentation and traceability, allowing for efficient root cause analysis. By reducing data-related bottlenecks and minimizing the time spent on data troubleshooting, organizations can expedite issue resolution processes, leading to improved operational agility, enhanced customer satisfaction, and a more proactive approach to data management and problem-solving.
Why Data Governance Matters?
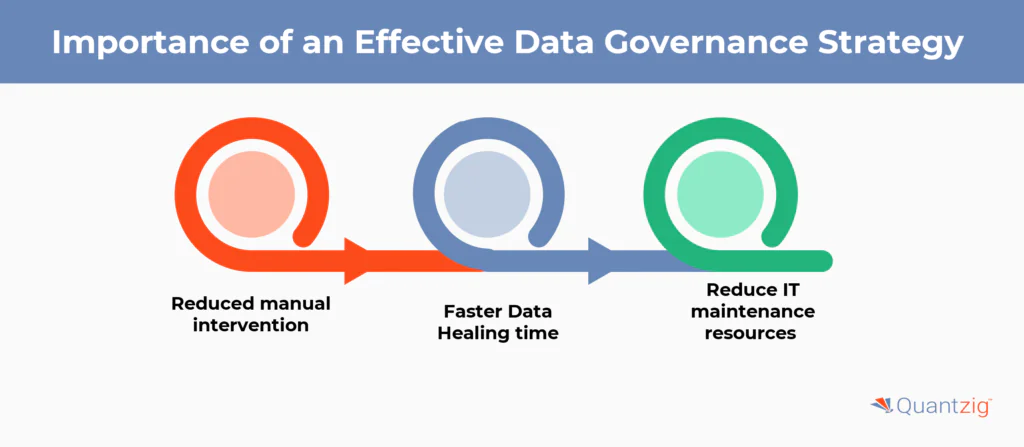
An effective data governance strategy is essential for maintaining data integrity, accuracy, and consistency, protecting sensitive information, and ensuring regulatory compliance. By defining roles, implementing security measures, and fostering data literacy, organizations optimize data utilization while safeguarding security. Reduced manual intervention through robust practices and automation enhances operational efficiency and data-driven decision-making. Moreover, faster data healing time and reduced IT maintenance resources bolster business continuity and productivity.
Aligning Data Governance with Data Strategy for Business Success
Data governance plays a crucial role in supporting and enabling an organization’s data strategy by ensuring the alignment of data and analytics initiatives with the overarching business objectives. When data governance and data strategy are properly aligned, organizations can effectively leverage data as a strategic asset to drive value and achieve their goals.
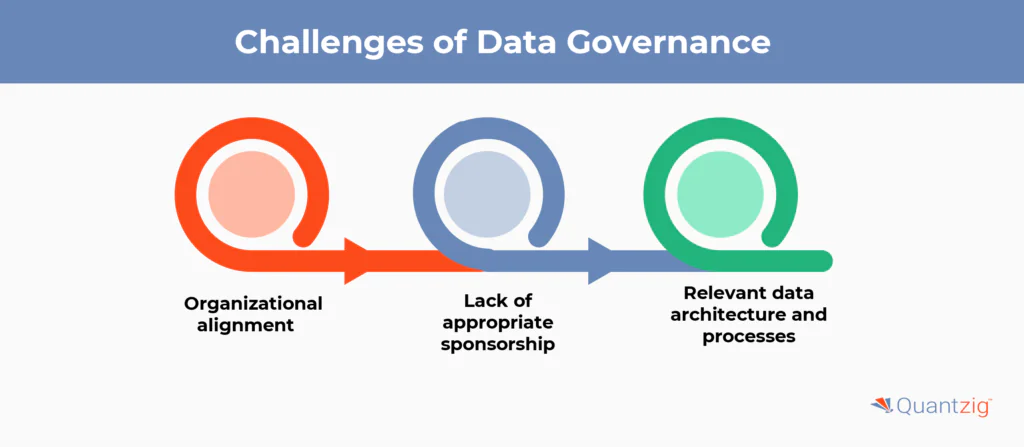
The Importance of Effective Data Governance in Data Strategy
When data governance is implemented correctly, it becomes an essential foundation for a successful data strategy. Here’s how data governance supports and enhances a data strategy:
- Data governance establishes framework, processes, guidelines ensuring data quality, integrity, confidentiality, availability.
- Framework ensures consistent data management, aligning with strategy objectives.
- Defines roles, responsibilities, accountabilities for data management, driving stewardship and accountability.
- Sets and enforces data standards, policies, procedures for consistency, quality, integrity.
- Facilitates data-driven decision-making by ensuring availability, reliability, usability of data.
- Alignment of data governance and strategy enables effective data utilization, innovation, goal achievement.
Best Practices for Managing Data Governance Initiatives
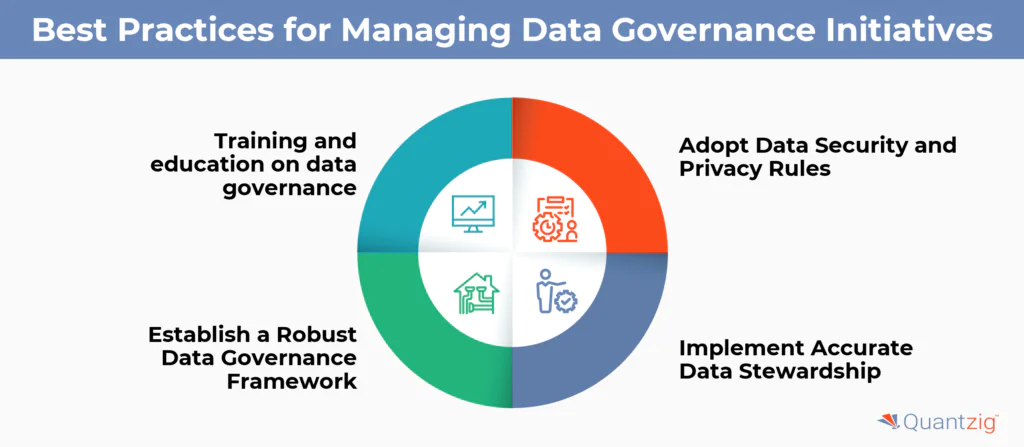
- Train data analysts and business users on privacy mandates and data usage rules, ensuring consistency through ongoing communication and outreach efforts.
- Adopt data privacy and security rules at the source system, implement governance policies throughout the organization, and review them regularly.
- Establish a robust data governance framework with defined policies, roles (Data Owners, Stewards, Custodians), and compliance measures.
- Implement accurate data stewardship by assigning dedicated stewards to oversee data quality, integrity, and compliance, utilizing advanced tools for automation and streamlining processes.
Experience the advantages firsthand by testing a customized complimentary pilot designed to address your specific requirements. Pilot studies are non-committal in nature.
Request a free pilotAn effective data governance strategy is vital for unleashing the potential of data assets, ensuring security, compliance, and accuracy. It streamlines processes, reduces manual intervention, enhances operational efficiency and decision-making, and leads to cost savings and increased productivity.