Highlights of the Case Study:
Particulars | Description |
Client | A leading retailer with a diverse product portfolio and multiple stores across the globe, our client was facing issues resulting from inaccurate retail demand forecast models. |
Business Challenge | The implications of its inaccurate methods resulted in either overstocking or understocking of the inventory, which led to reduced margins. |
Impact | Quantzig helped the client revamp its demand and supply forecast strategy using machine learning models. This solution provided by Quantzig helped the client forecast the demand for the next five years. |
Table of Contents
Game-Changing Solutions for the Retail Industry
In the retail industry, demand forecasting inventory stock levels is challenging as they are volatile, subjective, and primarily dependent on customer demand. Ensuring stock availability is crucial for a retailer to ensure on-time order fulfillment of the customers. Therefore, optimum forecasting of need is one of the most critical components that companies in the manufacturing industry use to optimize inventory budgeting and distribution. Accurate method also provides retailers with valuable information about their potential in the current market to make informed decisions on the following:
- Pricing
- Market potential
- Business growth strategies
Traditional Demand Forecasting Methods
• Time Series Analysis:
Time series analysis is a cornerstone among traditional methods, leveraging historical data through AI-driven techniques to identify patterns and trends over time. By employing machine learning algorithms, businesses can enhance the accuracy of their predictions, refining demand planning processes and staying ahead of the competition. This approach addresses the challenge of rapidly changing consumer preferences and assists in mitigating potential risks within the complexity of modern supply chains. Accurate demand forecasts achieved through Artificial Intelligence techniques contribute to reducing excess inventory, optimizing promotional and marketing campaign planning, and ultimately improving overall customer satisfaction.
• Qualitative Forecasting:
Qualitative forecast, a traditional method, incorporates expert judgment and market research, and can be bolstered by AI-driven solutions for improved accuracy. Machine learning algorithms can analyze qualitative data from interviews, focus groups, and surveys to anticipate future customer demand. Shifting consumer behaviors are better understood through AI, allowing businesses to tailor their product assortments and promotional strategies accordingly. While qualitative forecast might face challenges related to gaps in data collection, the integration of AI addresses these gaps, providing a more comprehensive understanding of consumer preferences and facilitating efficient and sustainable business practices.
• Causal Models:
Causal models, another traditional forecast method, establish relationships between demand and various influencing factors, with AI-powered predictive models offering a more nuanced analysis. Machine learning algorithms, such as regression analysis, enable businesses to quantify the impact of economic indicators, marketing campaigns, and external events on future demand. This integration helps businesses navigate the complexity of supply chains and respond to the challenges posed by the dynamic nature of consumer preferences. By embracing AI in causal models, organizations can achieve accurate demand forecasts and improve Gross Margin Return on Investment (GMROI) through data-driven decision-making.
• Moving Averages:
Moving averages, a straightforward traditional method, calculate average demand over a specified time period and benefit from AI-driven enhancements for increased precision. Machine learning-based systems can optimize the efficiency of moving averages by incorporating sophisticated algorithms. This integration addresses the constraints of traditional forecast methods, particularly in handling new product forecast and adapting to modern methodologies. Implementing AI Demand Forecasting solutions improve the accuracy of moving averages, enabling businesses to reduce excess inventory, optimize promotional strategies, and enhance the overall effectiveness of demand planning processes.
AI Techniques in Retail Demand Forecasting:
• Machine Learning Algorithms:
Implementing machine learning algorithms in retail is pivotal for accurate predictions and staying ahead of the competition. These algorithms, including decision trees, neural networks, and ensemble methods, enable businesses to refine demand planning processes and transition towards AI-driven forecasting. By leveraging historical data and considering factors such as shifting consumer behaviors, businesses can anticipate future customer demand and manage supply chain planning more effectively. Machine learning contributes to demand planning accuracy, reducing excess inventory and optimizing promotional strategies for improved overall customer satisfaction.
• Predictive Models for Future Demand Prediction:
AI-driven predictive models play a crucial role in future demand prediction within retail operations. These models, powered by advanced analytics and machine learning techniques, analyze rapidly changing consumer preferences and the complexity of supply chains. By mitigating potential risks through accurate demand forecasts, businesses can optimize inventory levels and enhance the efficiency of promotion and marketing campaign planning. The integration of AI in predictive models addresses specific demand planning challenges, such as gaps in data collection, facilitating a more comprehensive understanding of customer behavior.
• Custom AI-Powered System:
Developing this system tailors the solution to the unique needs of a business. By combining AI techniques and machine learning-based systems, organizations can create a sustainable and efficient forecast system. This approach considers factors like sustainability, product assortments, and promotional strategies to optimize business practices. The system improves Gross Margin Return on Investment (GMROI) by providing accurate demand forecasts, overcoming constraints associated with traditional methods. Retail big data analytics further supports the customization of the system, ensuring it aligns with modern methodologies.
• Retail AI Systems for Inventory Optimization:
Retail AI systems focus on inventory optimization by utilizing AI techniques to analyze the techniques. These systems adapt to new product forecast challenges and address the complexity of supply chains. By incorporating AI-driven solutions, businesses can efficiently manage inventory levels, reduce excess inventory, and enhance the accuracy of demand planning. This not only improves overall customer satisfaction but also facilitates process optimization in retail operations. The integration of AI in retail AI systems ensures adaptability to rapidly changing consumer preferences, making them indispensable for the modern retail landscape.
The Importance of Demand Forecast in Retail Big Data Analytics
• Strategic Inventory Management:
This tool is of paramount importance in retail big data analytics as it guides strategic inventory management. By utilizing AI techniques and machine learning algorithms, businesses can accurately predict future customer demand, allowing for optimized inventory levels. This not only ensures adequate stock to meet consumer needs but also helps reduce excess inventory, improving demand planning accuracy. The transition to AI-driven solutions enables retailers to stay ahead of the competition by efficiently managing inventory based on rapidly changing consumer preferences and mitigating potential risks associated with the complexity of modern supply chains.
• Enhanced Promotion and Marketing Campaign Planning:
This tool in retail big data analytics is instrumental in enhancing promotion and marketing campaign planning. AI-driven predictive models analyze vast datasets, anticipating future customer demand and facilitating targeted promotional strategies. This ensures that promotions align with shifting consumer behaviors and preferences, contributing to improved overall customer satisfaction. The integration of AI techniques addresses specific demand planning challenges, such as gaps in data collection, providing retailers with a comprehensive understanding of their customer base and enabling efficient and sustainable business practices.
• Optimizing Gross Margin Return on Investment (GMROI):
The accurate modern demand forecasting methodology derived from retail big data analytics, powered by AI and machine learning algorithms, play a crucial role in optimizing Gross Margin Return on Investment (GMROI). Retailers can implement custom AI-powered systems to tailor forecasts to their unique needs, incorporating sustainability, product assortments, and effective promotional strategies. This not only improves GMROI but also helps overcome demand forecasting constraints associated with traditional methods. Retail big data analytics further supports the customization of these techniques, ensuring retailers adopt modern methodologies and adapt to new product forecast challenges.
• Adaptation to Modern Methodologies:
Retail big data analytics emphasizes the importance of this tool in adapting to modern methodologies. AI-powered forecast solutions facilitate the transition from traditional forecast methods to more advanced approaches. By incorporating machine learning-based systems, businesses can efficiently navigate the rapidly changing landscape of consumer preferences and behaviors. This adaptability is crucial for retailers to implement new product forecast strategies, optimize promotional plans, and refine demand planning processes, ensuring they are at the forefront of the industry with accurate and forward-looking demand forecasts.
Demand Forecasting Techniques & Methodologies
• Time Series Analysis:
Time series analysis remains a foundational technique, employing historical data and AI techniques to identify patterns over time. Machine learning algorithms enhance the accuracy of time series analysis, allowing businesses to anticipate future customer demand with precision. This method is essential for refining demand planning processes, staying ahead of the competition, and navigating shifting consumer behaviors. By implementing AI-driven solutions, organizations can effectively manage inventory levels, reduce excess inventory, and optimize promotion and marketing campaign planning, thereby improving overall customer satisfaction.
• Qualitative Forecasting and AI Integration:
Qualitative forecast, complemented by AI techniques, involves expert judgment and market research to predict future demand. AI-driven predictive models analyze qualitative data, enabling businesses to refine demand planning processes and transition to AI-driven demand forecasting. This approach addresses specific demand planning challenges, such as gaps in data collection, and contributes to sustainable business practices. The integration of machine learning algorithms ensures accurate forecasts, allowing organizations to adapt to rapidly changing consumer preferences and efficiently manage inventory levels, ultimately enhancing customer satisfaction.
• Causal Models with Machine Learning:
Causal models establish relationships between demand and influencing factors, and their integration with machine learning algorithms provides a more sophisticated analysis. Businesses can leverage this technique to mitigate potential risks associated with the complexity of supply chains and ensure accurate demand forecasts. Machine learning enhances the accuracy of causal models, aiding in demand planning accuracy and the reduction of excess inventory. Additionally, the approach supports efficient promotion and marketing campaign planning, contributing to the optimization of Gross Margin Return on Investment (GMROI) through AI-powered decision-making.
• Custom AI-Powered Demand Forecasting System:
Developing a custom AI-powered system tailors the approach to the specific needs of a business. This methodology incorporates AI-driven techniques, sustainability considerations, and optimization of product assortments and promotional strategies. By implementing a machine learning-based system, organizations can efficiently navigate modern methodologies and adapt to new product forecast challenges. The system’s customization addresses constraints associated with traditional methods, ensuring accurate forecasts that lead to efficient and sustainable business practices, ultimately contributing to improved GMROI and overall business success.
The Challenges of the Retail Client
A leading retailer approached Quantzig with a diverse product portfolio and multiple stores across the globe. The client faced several challenges due to inaccurate models. The implications of their incorrect methods resulted in either overstocking or understocking of the inventory, which led to reduced margins. The client could not anticipate when the demand would be high and was having difficulty establishing a long-term model that could help in business growth. This also affected its margins. The client approached Quantzig to leverage its data-driven demand forecasting solutions to improve forecast accuracy and achieve a better order fulfillment ratio.
Retail Demand Forecasting Solutions
Quantzig’s experts identified that the client’s approach’s inefficiency resulted in inventory pile-ups and frequent stockouts for some SKUs, resulting in a net revenue loss. To help the client address this issue, Quantzig’s production planning and forecasting analytics team revamped its demand and supply forecasting strategy. Quantzig used machine learning models to build an advanced manufacturing demand forecasting tool that would forecast demand by week and over a month for the client’s most significant and volatile product lines. The Quantzig team came up with the “Ensemble Machine Learning” solution, which has combined predictions from multiple predictive models. Each method has some prerequisites, advantages, assumptions, and characteristics. This solution provided by Quantzig helped the company forecast the demand for the next five years.
Optimize your business strategies with Quantzig’s advanced demand forecast solutions, powered by cutting-edge analytics and machine learning algorithms. Drive profitability, reduce excess inventory, and stay ahead in the competitive market by leveraging our precision in anticipating and meeting future customer demand. To know more,
Request a free proposalImpact Analysis of Quantzig’s Ensemble Machine Learning Solution
The demand forecasting helped the company optimize the overall process (downstream) as the company was now able to make more precise sales decisions. The other advantages of this solution, Ensemble, were as follows:
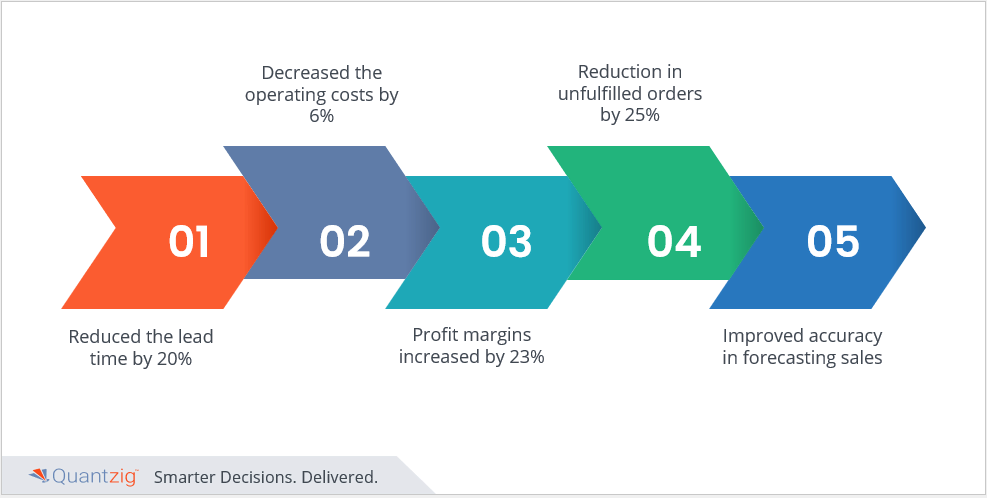
- Reduced the lead time by 20%
- Decreased operating costs by 6%
- Profit margins increased by 23%
- Achieved a 25% reduction in unfulfilled orders
- Improved accuracy for weekly and monthly sales demand by 7% and 9%, respectively
Key Outcomes
Accurate demand projection is essential for retailers to ensure customer product availability while ensuring minimal inventory accumulation. However, maintaining this precarious balance is a tall order, which may vary for each store location and channel on any given day. Retail demand planners struggle to consider many variables such as seasonal demand, promotions, in-store displays, and external factors, such as local competition, changes in the neighborhood, and even the climate.
The sheer number of variables makes it necessary for modern retailers to rely on machine learning to evaluate the impact of these variables on demand and, consequently, product inventory. Thus, most retailers are transitioning their technology strategies toward machine learning-based demand forecasting.
A Broad Perspective on the role of Retail Demand Forecasting Solutions:
Retailers have aggressive performance goals related to delivery time reliability, inventory optimization, demand management, order fulfillment rate, order cycle time, and inventory turnover; however, they cannot forecast demand and the factors affecting it accurately. Quantzig offers demand forecasting solutions that enable the supply chain, sales, product, and finance teams to collaborate and create accurate demand and capacity plans that could smoothen an organization’s inventory management and supply chain operation and increase its margins.
Key Takeaways
- Developed an analytics-based demand-supply forecast plan
- Increased margins
- Improved supply chain operations
- Optimized operations
- Improved cashflows
- Improved inventory management
Related articles:
How Inventory Management Solutions Helped a Pharma Retailer To Improve Overall Supply Chain Agility?
Supply Chain Optimization Helped a F&B Brand to Reduce Overhead Costs by 14%