Written By: Sudeshna Ghosh
Table of Contents
Key Takeaways
- Quantzig’s data strategy framework helped a US-based retail brand to improve existing data quality, increase new customer acquisition and enhance data-driven decision-making across stakeholders.
- A data strategy framework is a systematic method that guides the development and implementation of an effective data strategy, integrating a structured approach to managing and utilizing raw data as a strategic information asset within an organization.
- Implementing an effective data strategy requires defining clear business objectives, capturing accurate and relevant data, modernizing data architecture, establishing robust controls, creating integrated solutions, and scaling teams and processes to harness the full potential of data assets and drive business success.
- Quantzig offers a wide range of data analytics solutions including Data Engineering, Data Strategy Consulting, Business Analytics Services, Data Visualization & Reporting, and Business Process Automation.
Introduction to Data Strategy Framework
Today’s data leaders are continuously shifting their focus from traditional business intelligence to predictive models and real-time decision-making that help their organizations stay ahead. To achieve this, they must develop a comprehensive data strategy framework that can effectively manage data, align with business goals, and integrate data analytics and AI solutions across the entire organization. This strategy should empower people, define use cases that meet business needs, and span operational analytics, traditional analytics, data science, AI initiatives, IoT sensor data, data visualization, and new product development.
A clear data strategy is crucial for scaling AI effectively. However, fully leveraging the potential of data and AI requires creative decision-making, compelling storytelling, and cross-functional support. This article will shed light on how to develop and implement an effective data strategy framework tailored to the organization’s unique needs and goals, incorporating insights from industry leaders and best practices in data management and analytics.
Book a demo to experience the meaningful insights we derive from data through our analytical tools and platform capabilities. Schedule a demo today!
Request a Free DemoData Strategy Framework: Overview
A data strategy framework is a dynamic, comprehensive, and structured approach to managing and utilizing raw data as a strategic information asset within an organization. It defines the processes, technologies, principles, methodologies, and people necessary to support the collection, organization, assessment, and delivery of data to achieve the organization’s goals. This framework is designed to align data initiatives with business objectives, ensuring that data management practices are adaptable to constant change. By implementing this framework, data-driven organizations can streamline their data architecture, improve operational efficiency, enhance data integrity and compliance, and foster higher levels of innovation.
What is The Importance of a Data Strategy Framework?
Virtually every B2B business collects data from multiple forms and an efficient data strategy framework enables a business to manage and interpret all those data. It mainly puts the businesses to solve several challenges such as:
- Inefficient and slow business processes
- Data privacy, data quality, and data integrity issues that undercut your ability to analyze data
- A Lack of understanding of all the critical parts of the business (such as competitive landscape, customers, supply chain, etc.) and the processes that make them tick
- A lack of clarity about present business requirements (the problems that descriptive analytics can solve) and business goals (which both prescriptive and predictive analytics can identify)
- Inefficient data movement between different business parts, or data duplication by multiple business units
In a nutshell, a B2B business without an efficient data strategy framework is poorly positioned to grow successfully and operate efficiently and profitably.
Data Strategy Goals
Crafting an effective data strategy requires a multifaceted approach that addresses several key goals.
- First, innovation is crucial, as it enables businesses to create new value and efficiency. This means that data strategy must support and empower users across the organization, ensuring that they have the tools and insights needed to drive business success.
- Additionally, data strategy must address the critical issue of risk and regulations, which can vary significantly depending on the industry.
- Finally, IT resources and capabilities play a vital role in implementing and overseeing the tools and infrastructure that power the data strategy.
By focusing on these goals, organizations can create a comprehensive data strategy that drives business growth, improves operational efficiency, and ensures compliance with regulatory requirements.
What are the Key Components of An Effective Data Strategy Roadmap?
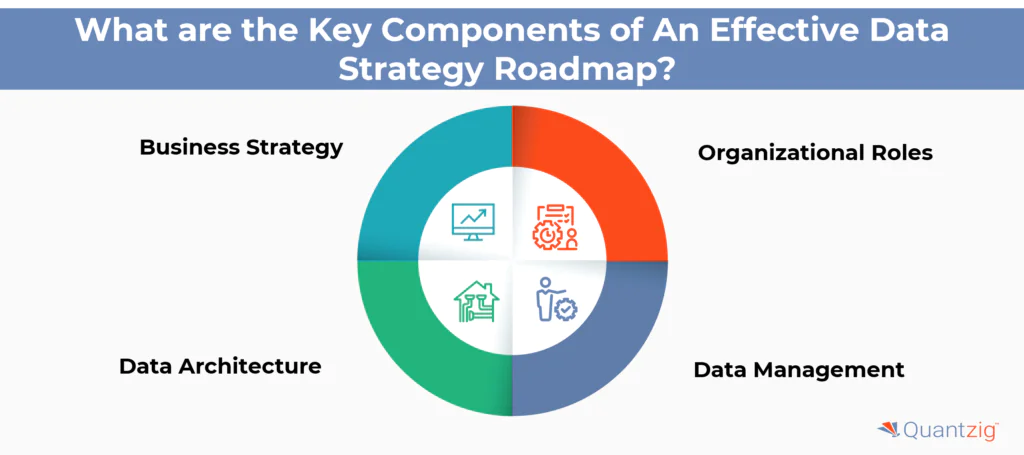
A data strategy is a crucial component of any organization’s overall business strategy. It outlines how data will be collected, managed, and analyzed to support business goals and objectives. While no two data strategies are identical, all successful data strategies include four key components that work together to put the strategy into practice.
1. Business Strategy
Business strategy involves aligning data initiatives with the organization’s overall business strategy, which includes the processes used to operate and improve the business. Clear goals and measurable objectives should be established to serve the larger business strategy. For example, a data strategy might include a goal of keeping data storage costs below a certain threshold. To achieve this goal, the strategy might define storage tools or services that meet the cost requirements, as well as best practices for optimizing storage costs. Metrics should be established to track success in achieving these goals.
2. Organizational Roles
A data strategy should document who does what with the data to facilitate collaboration and avoid duplication. Different roles within the organization will use data in different ways, and their roles in data collection, management, and analytics will vary. Data engineers oversee the data pipeline, data scientists work with the data, data analysts analyze and interpret data and business managers manage data operations and review data reports. When coordinating roles, consider everyone in the organization who uses data, even if working with data is not a primary part of their job responsibilities.
3. Data Architecture
Data architecture includes the tools and processes that allow data to be worked with and analyzed. Data architecture involves determining what datasets exist across the company, storing data in a single repository, integrating or transforming data for analysis, and creating a data pipeline to ingest raw data from disparate sources. Documenting and implementing data architecture is essential for a consistent and predictable data strategy.
4. Data Management
Data management encourages all team members to think of data as a business asset rather than a byproduct of business operations. Data governance establishes processes and responsibilities to ensure data quality and security. Policies should be updated as business needs change, and data management should be integrated with data architecture to ensure seamless data operations.
How to Implement an Effective Enterprise Data Strategy?
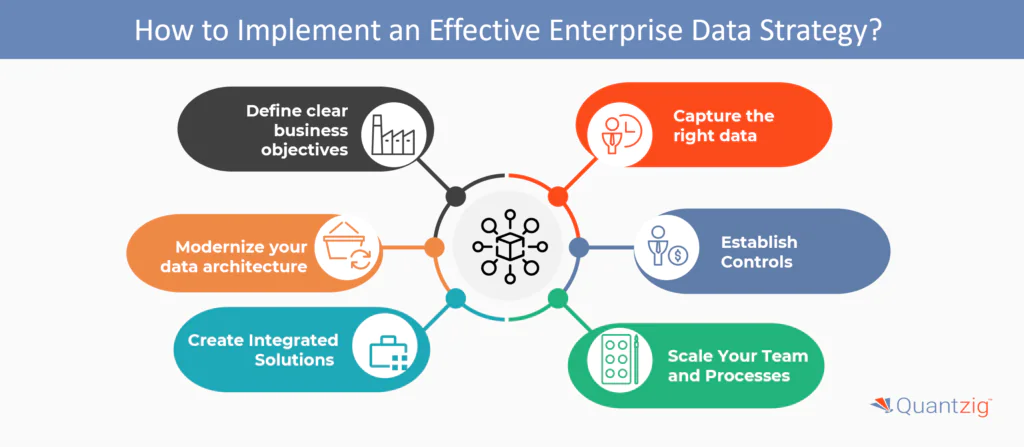
Implementing an effective enterprise data strategy is crucial for organizations seeking to harness the full potential of their data assets. A well-designed data strategy enables businesses to make informed decisions, drive innovation, and stay competitive in today’s data-driven landscape. By aligning data initiatives with business objectives, organizations can unlock new revenue streams, improve operational efficiency, and enhance customer experiences. In this section, we will outline the key steps and best practices for implementing a successful enterprise data strategy, empowering organizations to make the most of their data and drive business success.
Step 1
Define clear business objectives
This requires an in-depth understanding of the downstream and executive goals of your organization. In the end, having well-defined business objectives makes it easier to find key performance indicators (KPIs) and metrics that affect data-driven decisions. They also direct the identification and analysis of data sources.
Step 2
Capture the right data to support your objectives
To achieve success, B2B organizations must prioritize capturing the right data, ensuring that it is accurate, reliable, and relevant to their objectives. This involves not only collecting data but also ensuring that the sources are cleaned and certified. Without a well-structured data capture strategy, companies risk drowning in a sea of unorganized data, making it challenging to identify and utilize data that support various use cases and drive business growth. Effective data capture enables companies to develop a comprehensive data strategy that addresses both customer-focused business functions and legal, financial, compliance, and IT concerns. By implementing a robust data capture process, organizations can ensure that all reports, whether internal or external, are based on trusted, accurate data sources that adhere to standardized processes, fostering confidence and transparency across the organization.
Step 3
Modernize your data architecture
To effectively address complex business questions, a single data source is insufficient. Instead, organizations must leverage data from various sources and enrich it by combining different data sets. This approach shifts the focus from traditional, single-bucket data warehouses to a multi-bucket mindset that enables speed, agility, and high volumes of data. By adopting this approach, organizations can unlock the full potential of their data and make more informed decisions.
Step 4
Establish Controls
Implementing an effective enterprise data strategy requires establishing robust controls to ensure data quality, security, and compliance. This involves defining clear policies, roles, and responsibilities for data governance, data quality, and data security. Effective controls also include data access management, data encryption, and data backup and recovery processes. By establishing these controls, organizations can ensure that their data is trustworthy, secure, and compliant with regulatory requirements, thereby reducing the risk of data breaches and other data-related incidents.
Step 5
Create Integrated Solutions
To achieve an effective enterprise data strategy, organizations must create integrated solutions that seamlessly connect various data sources, systems, and applications. This involves integrating data from different departments, such as sales, marketing, and finance, to provide a unified view of the organization. Integrated solutions also include data warehousing, data lakes, and data visualization tools that enable data analysis and reporting across the organization. By creating integrated solutions, organizations can streamline data management, improve data quality, and enhance decision-making capabilities.
Step 6
Scale Your Team and Processes
Implementing an effective enterprise data strategy requires scaling your team and processes to handle the increased demands of data management. This involves hiring data professionals, such as data scientists, data engineers, and data analysts, to manage and analyze data. Additionally, organizations must develop processes for data governance, data quality, and data security to ensure that data is managed effectively and efficiently. By scaling their team and processes, organizations can ensure that they have the necessary resources to manage their data effectively and make data-driven decisions.
Data Management Supports Scale for an Enterprise Data Strategy
With a modernized data architecture in place, organizations must now focus on extracting value from their diverse data sources. However, each enterprise has unique requirements and solutions for its data strategy. To address this, businesses should adopt a flexible, self-service analytics platform that caters to a variety of use cases. This solution should also provide a mechanism for certifying data sources, ensuring that users have confidence in the data they utilize for analysis. Furthermore, the platform should offer seamless sharing of insights, enabling employees across the organization to answer questions, explore data further, and make informed business decisions.
Governance serves as the foundation for a successful self-service analytics deployment, working in tandem with data management tools like data catalogs. As organizations grapple with enterprise-wide data access and implement a unified data strategy, data catalogs play a crucial role. Data curation, a process that captures, cleanses, defines, and aligns disparate information, creates a bridge between data and its real-world applications. A data catalog defines and exposes an organization’s data terminology to enhance comprehension, outlines user permissions, tracks usage metrics, and provides lineage information.
When data governance and data management are effectively balanced within an organization’s data strategy, significant benefits emerge. The right data is placed in the hands of the right people, processes become more transparent, documented, and understood, and data-driven decisions are consistently made throughout the enterprise, driving success and innovation.
What Success Looks Like with an Effective Data Strategy
After implementing and scaling a data strategy, organizations can expect to see several key indicators of success. These include:
- Executive Advocacy: Strong support from senior leadership is crucial for a data strategy to be successful. This involves aligning business and data priorities and ensuring that the organization’s goals and needs are met through process and technology.
- Agility: The ability to adapt quickly to changing business needs and technological advancements is essential for a data strategy to remain effective.
- Data Proficiency: Organizations must ensure that their staff have the necessary skills and knowledge to effectively use data and analytics tools.
- Active Community: A broad, active community is needed to ensure the organization’s mission, goals, and needs are met.
Quantzig’s Expertise in Data Strategy Framework for a US-Based E-commerce Retail Brand
Category | Details |
---|---|
Client Details | An e-commerce retailer based in the United States with annual revenue of $10bn+. |
Challenges Faced by The Client | The client faced numerous hurdles, such as data silos that impeded access and integration, major problems with data quality that diminished the dependability of insights, and a lack of data governance framework that led to unclear and inconsistent data management practices. |
Solutions Offered by Quantzig | The client’s requirements were fulfilled by Quantzig’s data strategy framework solution, which integrated various data sources into a unified platform, putting in place a strong data quality management system, and establishing an extensive data governance framework with clear roles, duties, and guidelines. |
Impact Delivered | Better data accessibility, improved data quality, enhanced efficiency, and better decision-making. |
Client Details
One of our most recent clients is a well-known online retailer in the United States. Their business was expanding quickly, which caused a data explosion from many sources. However, the company’s inability to effectively leverage its data was being hampered by the absence of a structured data management approach, which resulted in inefficiencies and lost opportunities.
Challenges in Data Strategy Framework Faced by the Client
- Data Silos: It was challenging to access and integrate the company’s data for analysis because it was dispersed among several departments and systems.
- Data Quality Issues: The client’s data-driven insights were less reliable due to serious data quality issues, such as missing values, inaccuracies, and inconsistencies.
- Absence of Data Governance: The organization’s data management procedures were unclear and inconsistent due to the absence of a clear data governance framework.
Data Strategy Framework Offered by Quantzig for the Client
The data strategy framework solution from Quantzig was created to specifically address the needs and challenges of the client. The solution comprised:
- Data Integration: To facilitate easy access to and analysis of the client’s data, Quantzig combined its various data sources into a single, cohesive platform.
- Data Quality Management: To guarantee high-quality data, our team put in place a strong framework for data quality management that included data cleansing, profiling, and validation.
- Data Governance: Quantzig created a thorough framework for data governance that specified roles, duties, and guidelines for managing data throughout the company.
Impact Delivered Using Quantzig’s Expertise
Our client saw several noteworthy advantages from Quantzig’s data strategy framework solution, including:
- Better Data Accessibility: The client’s data are now integrated and readily available, allowing for a quicker and more precise analysis.
- Improved Data Quality: By putting data quality management procedures into place, high-quality data was guaranteed, with fewer errors and inconsistencies.
- Enhanced Efficiency: By eliminating effort duplication and streamlining data management procedures, the data governance framework enhanced efficiency all around.
- Better Decision-Making: The client was able to leverage its data more effectively, enabling more informed decision-making and driving business growth.
Quantzig’s data strategy framework solutions empowered the e-commerce retailer to streamline operations, enhance customer experiences, and drive business growth. By leveraging automation, data-driven insights, and agility, the client was able to stay competitive and thrive in a rapidly evolving market.
Also Read: Understanding Omnichannel Marketing Analytics for Businesses
Get started with your complimentary trial today and delve into our platform without any obligations. Explore our wide range of customized, consumption driven analytical solutions services built across the analytical maturity levels.
Start your Free Trial TodayHow Can Quantzig’s Data Strategy Framework Help B2B Businesses?
Quantzig’s comprehensive data management solutions empower organizations to harness the full potential of their data. Their portfolio includes a range of services designed to streamline data infrastructure, enhance accessibility, and ensure data integrity. Key offerings include:
- Data Engineering: Leveraging state-of-the-art industry frameworks, Quantzig simplifies data stacks, optimizing data flow and ensuring data integrity.
- Data Strategy Consulting: Quantzig crafts tailored analytics roadmaps, identifies process gaps, and introduces plug-and-play business acceleration modules to drive sustainable growth and informed decision-making.
- Business Analytics Services: Quantzig specializes in identifying solutions to complex business problems, enhancing performance across the entire value chain, and driving informed decisions.
- Data Visualization & Reporting: Intuitive, three-click visualizations simplify decision-making, providing actionable insights and driving efficiency across the organization.
- Business Process Automation: Custom apps streamline and automate business processes, reducing manual intervention and unlocking substantial time and cost savings.
By investing in Quantzig’s data management solutions, organizations can unlock the full potential of their data, drive innovation, and maintain a competitive edge in the market.
Also Read: Guide to Advanced Marketing Analytics Solutions and Its Business Benefits
Experience the advantages firsthand by testing a customized complimentary pilot designed to address your specific requirements. Pilot studies are non-committal in nature.
Request a Free PilotConclusion
In conclusion, a well-crafted data strategy framework is the foundation upon which organizations can build a data-driven future. By implementing a framework that aligns with business objectives, leverages AI and analytics capabilities, and fosters collaboration across functions, organizations can unlock the full potential of their data assets. To achieve success, it is essential to empower data-driven decision-making, drive real-time insights, and continuously refine the framework to meet evolving business needs. By following the six-step framework outlined in this article, organizations can establish a robust data strategy that drives business growth, improves operational efficiency, and sets them apart from competitors in a rapidly changing market.