Table of Contents
Introduction to Churn Prediction in Telecom
Customer churn, also known as customer attrition, is a critical challenge faced by companies in various industries, particularly in the telecom sector. It refers to the phenomenon where customers discontinue their services with a company and switch to a competitor or alternative provider. Churn prediction, therefore, becomes imperative for telecom operators to identify customers at risk of churn and take proactive measures to retain them.
Churn prediction models utilize advanced analytical techniques, including machine learning algorithms, to forecast which customers are likely to churn. By analyzing historical data on churned customers and their behaviors, these models can identify patterns and indicators that signal potential churn. This allows telecom companies to implement targeted retention strategies to mitigate customer churn effectively.
In the telecom industry, where competition is fierce and customer loyalty is pivotal, accurately predicting churn is crucial for maintaining profitability and sustaining market share. Companies like SyriaTel telecom company, operating in dynamic and competitive environments, heavily rely on churn prediction models to optimize customer retention efforts and enhance overall customer satisfaction.
Telecom operators leverage vast datasets encompassing customer demographics, usage patterns, billing history, customer service interactions, and more to develop robust churn prediction models. By harnessing machine learning techniques such as logistic regression, decision trees, random forests, and neural networks, these models can achieve high accuracy in forecasting customer churn.
The accuracy of churn prediction models is a key metric evaluated by telecom companies to assess the effectiveness of their predictive analytics initiatives. Improving the accuracy of prediction enables telecom operators to allocate resources efficiently, tailor retention strategies to specific customer segments, and ultimately reduce customer churn rates.
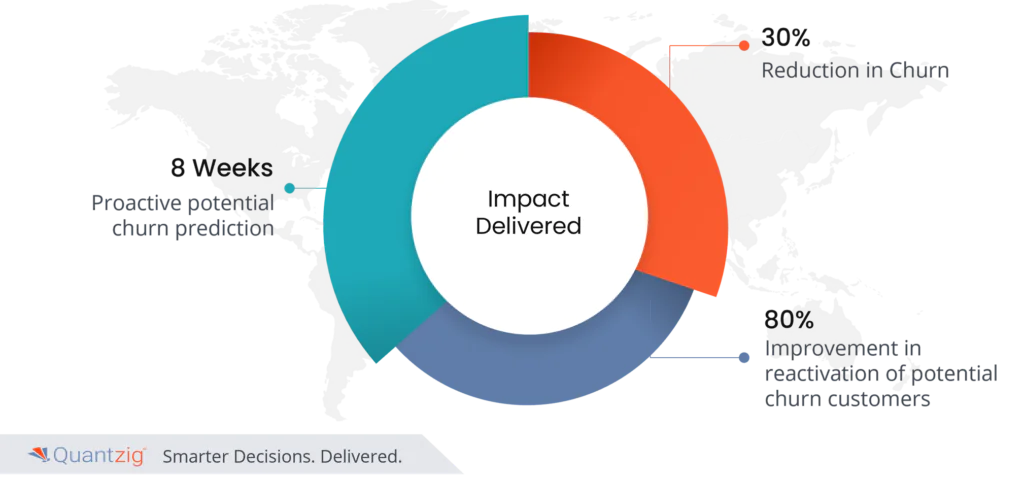
Summary
- Client: A prominent player in the telecom industry, our recent client was a leading provider of mobile services with a vast customer base. They faced significant challenges in understanding customer churn patterns, which hindered their efforts to retain subscribers and optimize profitability.
- Challenges: The client’s primary challenge was the lack of insights into customer behavior and factors leading to churn. They struggled to identify key churn drivers, resulting in an inability to proactively retain customers. Additionally, their existing churn prediction models lacked accuracy and failed to provide actionable recommendations for targeted retention strategies. Solutions: We created an end-to-end solution that combines the churn probability prediction for each customer in each of the service portfolios. It helped the client identify high-risk customers and devise targeted retention strategies, including personalized offers, proactive customer support, and service quality improvement. The solution also integrated a response model to identify the most effective nudge for the potential customer and the right message and campaign to target the customer. By leveraging SageMaker’s robust machine-learning capabilities and Python’s extensive libraries, the client gained the agility and scalability necessary to efficiently analyze large volumes of data, train complex models, and generate actionable insights for effective churn prediction and customer retention strategies.
Other Challenges in Churn Prediction for the Telecom Sector
While churn prediction is essential for telecom operators to mitigate customer attrition, several challenges must be addressed to enhance the effectiveness of churn prediction models and retention strategies. These challenges include:
1. Data Quality and Integration: Telecom companies often deal with vast amounts of data from disparate sources, including customer profiles, call records, billing information, and network performance metrics. Ensuring the quality and integration of these diverse datasets is crucial for accurate churn prediction.
2. Feature Selection and Engineering: Identifying relevant features or predictors of churn from the myriad of available data variables can be challenging. Telecom operators need to employ effective feature selection and engineering techniques to extract meaningful insights and improve the predictive power of churn models.
3. Imbalanced Data: In many cases, churned customers represent a small proportion of the total customer base, leading to imbalanced datasets. Imbalanced data can skew the performance of churn prediction models, requiring specialized techniques such as oversampling, undersampling, or synthetic data generation to address this issue.
4. Temporal Dynamics: Customer behaviors and preferences in the telecom sector can exhibit temporal dynamics, influenced by factors such as promotional offers, network quality, and market competition. Churn prediction models need to account for these temporal dynamics to maintain relevance and accuracy over time.
5. Model Interpretability: While advanced machine learning techniques can deliver high predictive accuracy, the interpretability of these models may be limited. Interpretable models are essential for gaining insights into the factors driving churn and informing targeted retention strategies.
6. Privacy and Regulatory Compliance: Telecom companies must adhere to strict privacy regulations and data protection laws when handling customer data for churn prediction purposes. Ensuring compliance with regulations such as GDPR (General Data Protection Regulation) and CCPA (California Consumer Privacy Act) is crucial to maintain customer trust and avoid legal repercussions.
7. Scalability and Real-time Processing: As telecom operators serve millions of subscribers, churn prediction models must be scalable to handle large-scale data processing and real-time predictions. Scalability ensures timely intervention and proactive retention efforts to prevent customer churn effectively.
8. Market Dynamics and External Factors: External factors such as economic conditions, industry trends, and competitive landscape can significantly impact customer churn rates. Telecom companies need to factor in these market dynamics when developing churn prediction models and adjusting retention strategies accordingly.
Addressing these challenges requires a comprehensive approach encompassing data management, advanced analytics, regulatory compliance, and strategic decision-making. By overcoming these obstacles, telecom operators can enhance the accuracy and effectiveness of churn prediction efforts, ultimately driving customer retention and business success in the competitive telecom sector.
Impact Delivered
All of the above initiatives resulted in:
- Potential churn prediction about 8 weeks prior to churn
- 30% reduction in churn
- 80% improvement in reactivation of potential churn customers
About the Client
Our recent client was a leading telecom company, a prominent player in the dynamic and competitive telecommunications industry. With a strong presence across more than 7 countries, they deliver cutting-edge connectivity solutions to a wide range of customers worldwide. The company boasts a workforce of 40000 dedicated employees, who contribute to their long-term success and drive their business forward.
Challenges
- In today’s fast-paced world, where choices abound, the battle to retain customers has become more critical than ever. Our client offers a diverse range of services, including telecommunication, broadband, streaming service, and international telephone service, and was suffering from the daunting challenge of reducing churn and keeping customers satisfied. Faced with sky-high customer acquisition costs, they set out to unravel the secrets of customer retention through the cutting-edge marvel of churn propensity modeling.
- Challenges faced by the Client:
- Service Diversity: One of the key challenges our client encountered was managing customers who subscribed to multiple services as well as those with limited-service subscriptions. This required understanding the varying preferences and behaviors of customers across different service types, ensuring tailored retention strategies for each segment.
- High Customer Acquisition Costs: The astronomical expense of acquiring new customers intensified the urgency to reduce churn. Each churned customer meant not only lost revenue but also the need to invest heavily in finding a costly replacement. The client faced the challenge of developing an effective churn propensity model to identify customers most likely to churn and intervene before it happened.
- Complex Data Integration: Integrating and analyzing data from multiple service offerings, customer profiles, and usage patterns posed a considerable challenge. The client needed to unify disparate data sources, ensuring accuracy and reliability to extract valuable insights for effective churn prediction and intervention.
- Customer behavior understanding: Gaining deep insights customer behavior patterns and identifying the early signs of potential churn required comprehensive analysis. The client had to overcome the challenge of deciphering complex customer interactions, usage patterns, and preferences to develop accurate churn propensity models.
To overcome these types of challenges our Quantzig professionals have built effective solutions, have a look.,
Request a free demoSolutions
- In a world where customer retention is paramount, we revolutionized our client’s approach with our comprehensive end-to-end solution. By seamlessly merging churn probability prediction across diverse service portfolios, we equipped the client with invaluable insights into their customer base. Our solution empowered their team to identify customers at risk of churn with pinpoint accuracy. Armed with this knowledge, they could take proactive measures, ensuring their precious clientele remained loyal and satisfied.
- We recognized that effective customer engagement required more than just predicting churn. That’s why our solution also integrated a response model to identify the most effective nudge for the potential customer and the right message and campaign to target the customer. As a result, our client witnessed a surge in customer engagement and response rates as the tailored nudges struck a chord with their audience. Customers felt understood and valued, leading to strengthened relationships and increased customer lifetime value. The days of generic campaigns and missed opportunities were replaced with a strategic and customer-centric approach that set the client apart.
- Customer churn has become a significant hurdle faced by a lot of companies, from banking to telecommunications, where most of the customers are typically lost to business competitors. Nowadays, the telecom industry focuses more on retaining existing customers rather than acquiring new customers as it usually costs more to attract many new customers. Amazon SageMaker offers a suite of pre-trained models, pre-built solution templates, and built-in algorithms to help machine learning practitioners and data scientists get started on deploying ML models quickly.
- AWS SageMaker, coupled with the power of Python, revolutionized churn modeling for our client. With SageMaker’s cutting-edge machine-learning capabilities and Python’s versatility, they harnessed the potential to predict and prevent customer churn like never before. Leveraging SageMaker’s pre-built algorithms and Python’s dynamic libraries, they effortlessly sifted through vast amounts of customer data, extracting invaluable insights. Its automatic model tuning empowered them to identify the most accurate predictive models swiftly. It was a game-changer, putting the client at the forefront of customer retention strategies.
In conclusion, churn prediction in the telecom industry plays a pivotal role in enabling companies to proactively address customer attrition, enhance customer retention efforts, and sustain business growth in a competitive landscape. By leveraging machine learning techniques and sophisticated predictive analytics, telecom operators can optimize their operations, maximize customer lifetime value, and maintain a strong foothold in the ever-evolving telecommunications sector.