Written By: Sudeshna Ghosh
Table of Contents
Key Takeaways
- Quantzig’s data quality management solution helped a US-based retail brand to experience a 40% uplift in new customer acquisition and improve transparency across stakeholders.
- Data Quality Management (DQM) is the process of maintaining and ensuring the accuracy, completeness, consistency, and timeliness of data throughout its lifecycle, from acquisition to analysis, to support informed decision-making and business operations.
- The utilization of advanced data quality tools that automate data quality checks, detect inconsistencies, and provide real-time insights can enhance data integrity and support better business outcomes.
- Quantzig offers a wide range of data quality management solutions including Data Engineering, Data Strategy Consulting, Business Analytics Services, Data Visualization & Reporting, and Business Process Automation.
Introduction to Data Quality Management
As businesses increasingly rely on data analytics to navigate the complexities of a rapidly evolving market, the integrity of their datasets has become a critical differentiator between success and failure. In an era where a single data error can have far-reaching consequences, from compromised customer trust to substantial financial losses, organizations must prioritize data quality management to ensure the veracity and consistency of their data assets. By doing so, they can unlock the full potential of their data, and maintain a competitive edge in an environment where data-driven insights are the lifeblood of strategic decision-making.
In this article, we will explore the essential aspects of data quality management that B2B businesses need to know to maintain data integrity, drive business growth, and stay ahead of the competition.
Book a demo to experience the meaningful insights we derive from data through our analytical tools and platform capabilities. Schedule a demo today!
Request a Free DemoWhat is The Definition of Data Quality?
Data quality, a critical aspect of any organization, refers to the assessment of the information you have relative to its purpose and ability to serve that purpose. This process is defined by various factors, including accuracy, completeness, consistency, and timeliness, which collectively determine its suitability for operational, planning, and decision-making purposes. In the context of the B2B industry, ensuring high-quality data is essential for informed decision-making and efficient operations, as it directly impacts the accuracy and reliability of insights derived from data analysis.
What is Data Quality Management?
Data Quality management is a systematic approach to guaranteeing the accuracy, reliability, and completeness of data inside the organizations. This entire process includes a collection of methods and procedures covering every stage of the data lifecycle, from obtaining and storing data to analyzing and reporting it.
Establishing a framework that enables businesses to preserve high-quality data in support of regulatory compliance, well-informed decision-making, and operational efficiency is the key objective of DQM. B2B businesses lower the expenses of low data quality, including squandered resources, lost opportunities, and reputation damage, by putting DQM into practice.
Identifying data quality metrics, putting data profiling and cleansing tools into practice, and making data governance policies are all part of DQM. Together with constant monitoring and enhancement of data stewards, data quality, IT specialists, and business stakeholders must work together. Organizations can fully utilize their data assets and obtain a competitive edge in today’s data-driven business environment by placing a high priority on data quality.
What is The Importance of Data Quality Management?
Making sense of your data is a crucial step that can ultimately improve your financial performance. This is where data quality management comes in.
1. Building a Strong Foundation
Effective data quality management establishes a framework for all departments within an organization and provides clear guidelines for data collection, storage, and usage. This framework ensures that data is accurate, complete, and consistent, thereby reducing the risk of errors and missteps. According to the Quantzig experts, 80% of businesses that implement robust DQM processes report significant improvements in data accuracy and reliability.
2. Enhancing Operational Efficiency
Accurate and up-to-date data provides a clear picture of day-to-day operations, enabling businesses to make informed decisions and optimize processes. Data quality management also reduces unnecessary costs by minimizing errors and oversights. For instance, a study by Quantzig found that poor data quality can lead to a 20% increase in operational costs.
3. Meeting Compliance and Risk Objectives
Good data governance requires clear procedures and communication, as well as good underlying data. Data quality management ensures that data is secure, compliant, and trustworthy, thereby meeting compliance and risk objectives. Quantzig experts found that 75% of businesses that implement robust DQM processes report improved compliance with regulatory requirements.
In a nutshell, by ensuring high-quality data, businesses can build a strong foundation for informed decision-making, enhance operational efficiency, and meet compliance and risk objectives. As the data landscape continues to evolve, businesses need to prioritize data quality management and leverage advanced technologies to drive growth and success.
The Dimensions of Data Quality Management
Several dimensions of data quality are employed. A few of the fundamental dimensions are consistent across data sources, but this list keeps expanding as the volume and variety of data increases.
- The degree of accuracy in data values is measured, and it is essential to have accurate data to make meaningful conclusions.
- When a data set is complete, every element has a measurable value.
- The goal of consistency is to have consistent data elements with values derived from a recognized reference data domain across various data instances.
- Age considers the requirement that all data be current and fresh, with values that are up to date.
- To prevent duplication, uniqueness shows that each record or element is only ever represented once in a data set.
The 5 Pillars of Data Quality Management
This process is essential for ensuring the accuracy, reliability, and integrity of data within an organization. High-quality data is the cornerstone of effective data analysis and artificial intelligence and machine learning applications, as it enables precise insights and informed decision-making. Robust data cleansing processes and stringent data governance frameworks help maintain data integrity, facilitating seamless data integration and data transformation across various systems and platforms. Furthermore, maintaining high data quality supports compliance with regulatory standards and enhances security measures, safeguarding sensitive information within the data warehouse. This technique is pivotal for optimizing operational efficiency and driving strategic business growth. To establish a robust data foundation and ensure the accuracy, completeness, and consistency of your data, it is essential to understand the techniques and pillars that underlie Data Quality Management (DQM).
1. The people
Under DQM, technology serves as a powerful enabler, but it is the people behind it who truly drive its success. As organizations face the complexities of managing vast amounts of data, it is crucial to have a well-defined team structure with clearly defined roles and responsibilities. Here are the key roles that form the foundation of an effective DQM system:
a. DQM Program Manager
The DQM Program Manager is a high-level leader who takes ownership of the overall vision and strategy for data quality initiatives. This individual is responsible for providing leadership and oversight, ensuring that business intelligence projects align with organizational goals. The Program Manager oversees the daily activities related to data scope, project budget, and program implementation, serving as the driving force behind the pursuit of quality data and measurable ROI.
b. Organization Change Manager
The Organization Change Manager plays a vital role in facilitating the adoption of advanced data technology solutions. As quality issues are often highlighted through dashboard technology, the Change Manager is responsible for visualizing data quality and communicating the importance of data quality to stakeholders across the organization. By providing clarity and insight, the Change Manager helps drive organizational change and ensures that data quality becomes an integral part of the company culture.
c. Business/Data Analyst
The Business/Data Analyst is the bridge between the theoretical and practical aspects of data quality. This individual defines the quality needs from an organizational perspective and translates them into actionable data models for acquisition and delivery. The Business/Data Analyst ensures that the theory behind data quality is effectively communicated to the development team, enabling them to create data models that meet the organization’s specific needs.
By assembling a team with these key roles, organizations can establish a strong foundation for effective DQM. The DQM Program Manager provides strategic direction, the Organization Change Manager facilitates organizational adoption, and the Business/Data Analyst ensures that data quality initiatives are aligned with business objectives. Together, these roles form the cornerstone of a successful DQM system, empowering organizations to harness the power of data and drive informed decision-making.
2. Data profiling
Data profiling is a pivotal process in the Data Quality Management (DQM) lifecycle, aimed at gaining a deeper understanding of existing data and comparing it to quality goals. This comprehensive process involves several key steps:
- Data Review: A detailed examination of the data to identify any inconsistencies or errors.
- Metadata Comparison: A comparison of the data to its metadata to ensure consistency and accuracy.
- Statistical Modeling: The application of statistical models to analyze data and identify trends and patterns.
- Data Quality Reporting: The generation of reports highlighting data quality issues and areas for improvement.
By initiating this process, businesses can develop a solid foundation for their DQM efforts and establish a benchmark for improving their data quality. Accurate and complete data is crucial for this step, as it helps identify disproportionate numbers and ensures that all data points are whole. This information will be further explored in the third part of this article.
3. Defining data quality
The third pillar of DQM is quality itself, which is achieved by creating and defining quality rules based on business goals and requirements. These rules, both business and technical, serve as the foundation for ensuring that data is viable and reliable. Business requirements take center stage in this pillar, as critical data elements vary by industry and are crucial for informed decision-making.
Developing quality rules is important for the success of any DQM process. These rules detect and prevent compromised data from affecting the integrity of the entire dataset. By implementing quality rules, organizations can ensure that their data is accurate, complete, and consistent, thereby enabling the detection of trends and the reporting of analytics. When combined with online Business Intelligence (BI) tools, these rules become a powerful tool for predicting trends and making data-driven decisions.
4. Data reporting
Data quality reporting is a crucial process in DQM, aimed at identifying and documenting compromising data. This process should be designed to follow a natural flow of data rule enforcement, ensuring that data quality standards are consistently met. Once exceptions are identified and captured, they should be aggregated to identify quality patterns and trends.
a. Data Modeling and Reporting
Captured data points should be modeled and defined based on specific characteristics, such as by rule, date, source, and so on. Once this data is aggregated, it can be connected to an online reporting platform to provide real-time insights into data quality and exceptions. Automated reporting and “on-demand” technology solutions should be implemented to ensure that dashboard insights are available in real time.
b. The Importance of Real-Time Reporting
Real-time reporting and monitoring are essential components of enterprise data quality management, as they provide visibility into the state of data at any moment. By allowing businesses to identify the location and origin of data exceptions, data specialists can develop targeted remediation strategies to ensure data quality and integrity.
c. Data Quality Management ROI
Effective data quality reporting and monitoring are critical to achieving a positive return on investment (ROI) in data quality management. By providing real-time insights into data quality and exceptions, businesses can identify areas for improvement and optimize their data quality processes to achieve better outcomes.
5. Data repair
Data repair is a critical process in DQM, involving a two-step approach to rectifying data defects. The first step involves determining the best method for remediation and the most efficient way to implement the necessary changes. The second step involves conducting a thorough examination of the root cause of the data defect to identify why, where, and how it originated.
a. Root Cause Analysis
The root cause examination is a crucial aspect of data remediation. By identifying the underlying reasons for the data defect, organizations can develop a targeted remediation plan to address the issue. This examination helps to determine the source of the problem, ensuring that the remediation process is effective and efficient.
b. Impact on Business Processes
Data processes that rely on previously identified data may need to be re-initiated, particularly if their functioning is compromised or at risk by the defective data. This includes campaigns, reports, and financial documentation, which may be impacted by inaccurate or incomplete data. By re-initiating these processes, organizations can ensure that their data is accurate and reliable, leading to improved business outcomes.
c. Review and Update Data Quality Rules
The data repair process also involves reviewing and updating data quality rules to ensure that they are effective and relevant. This review process helps to identify areas where rules need to be adjusted or updated, enabling organizations to refine their data quality processes and improve data integrity.
d. Benefits of High-Quality Data
Once data is deemed high-quality, critical business functions and processes can run more efficiently and accurately, resulting in lower costs and a higher ROI. By ensuring data quality, organizations can make informed decisions, improve operational efficiency, and enhance their competitive edge.
What is The Data Quality Management Lifecycle?
A systematic procedure known as the data quality management lifecycle guarantees high-quality data at every stage of the data’s life cycle. It entails several crucial actions that complement one another to maintain and enhance data quality:
- Data Collection: It involves compiling information from a range of internal and external data sources.
- Assessment: Assessing whether the gathered data satisfies the necessary quality standards.
- Data Cleansing: This is the process of eliminating redundant, improperly formatted, or unnecessary data to guarantee accuracy and relevance.
- Integration: Integration is the process of bringing together data from several sources to present the data comprehensively.
- Reporting: Tracking and monitoring data quality and identifying possible problems with using key performance indicators (KPIs).
- Repairing: Fixing any mistakes or discrepancies discovered throughout the reporting stage to preserve the data’s integrity.
These procedures are pivotal in guaranteeing the precision, entirety, and coherence of data—a prerequisite for well-informed decision-making and efficient business functions.
What are the Best Practices of Data Quality Management?
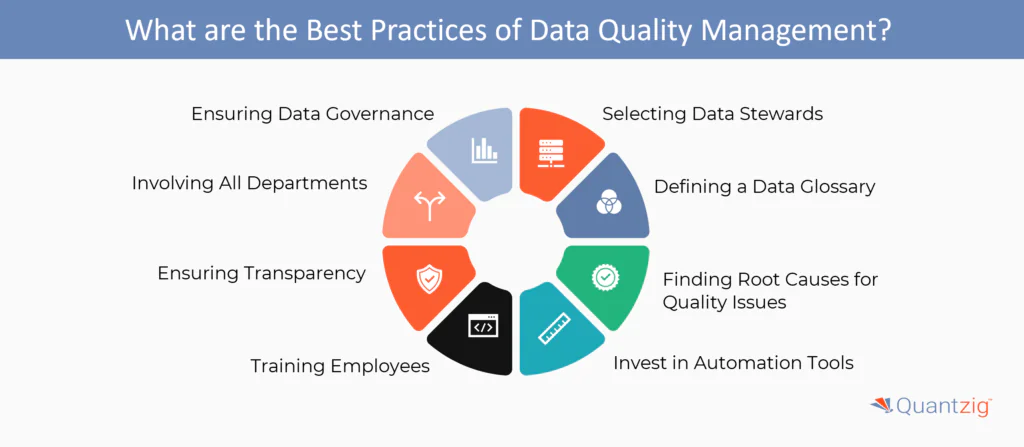
In our comprehensive overview of the 5 pillars of Data Quality Management (DQM), we have highlighted the essential techniques and tips for ensuring a successful process. To help you effectively implement these strategies, we have compiled a concise summary of the key points to remember when assessing your data. By following these best practices, you can ensure that your data is accurate, complete, and consistent, making it ready for analysis and informed decision-making.
1. Ensuring Data Governance
Data governance is a crucial aspect of data quality management, encompassing a set of roles, standards, processes, and key performance indicators (KPIs) that ensure organizations use data efficiently and securely. Implementing an effective governance system is a crucial step in defining DQM responsibilities and roles, and it is essential to keep every employee accountable for how they access and manipulate data.
2. Involving All Departments
While specialized roles are necessary for DQM, it is also essential to involve the entire organization in the process. This includes data analysts, data quality managers, and other stakeholders who play a critical role in ensuring data quality.
3. Ensuring Transparency
To successfully integrate all relevant stakeholders, it is necessary to offer them a high level of transparency. Ensure that all rules and processes regarding data management are informed across the organization to avoid any mistakes that could damage your efforts.
4. Training Employees
Assessing employees’ data literacy is fundamental to ensuring data quality. If employees do not know how to manage information successfully and identify bad-quality signs, all your efforts are worth nothing. We recommend offering training instances to whoever needs them and providing the necessary tools to boost communication and collaboration for this process.
5. Selecting Data Stewards
Another best practice for integrating everyone in the process is setting up data stewards. While investing in training is a great way to ensure data quality across your organization, selecting data stewards responsible for ensuring quality in specific areas can be very beneficial, especially for large enterprises with many areas and departments. Stewards will ensure governance policies are respected, and quality frameworks are implemented.
6. Defining a Data Glossary
Producing a data glossary as part of your governance plan is a good practice. This glossary should contain a collection of all relevant terms used to define company data in a way that is accessible and easy to navigate. This ensures a common understanding of data definitions across the organization.
7. Finding Root Causes for Quality Issues
If you find poor data quality issues in your business, it is not necessary to just toss it all out. Bad-quality data can also provide insights that will help you improve your processes in the future. A good practice here is to review the current data, find the root of the quality issues, and fix them. This will not only help you set the grounds to work with clean, high-quality data but will also help you identify common issues that can be avoided or prevented in the future.
8. Invest in Automation Tools
Due to the high likelihood of human error, manual data entry is thought to be one of the most frequent causes of poor data quality. Businesses that need a large workforce to enter data are more vulnerable to this threat. Investing in automation tools to handle the entry process is a good practice to prevent this. These tools can guarantee that all your data is accurate and set to your rules and integrations.
5 Data Quality Metrics to Measure Data Quality
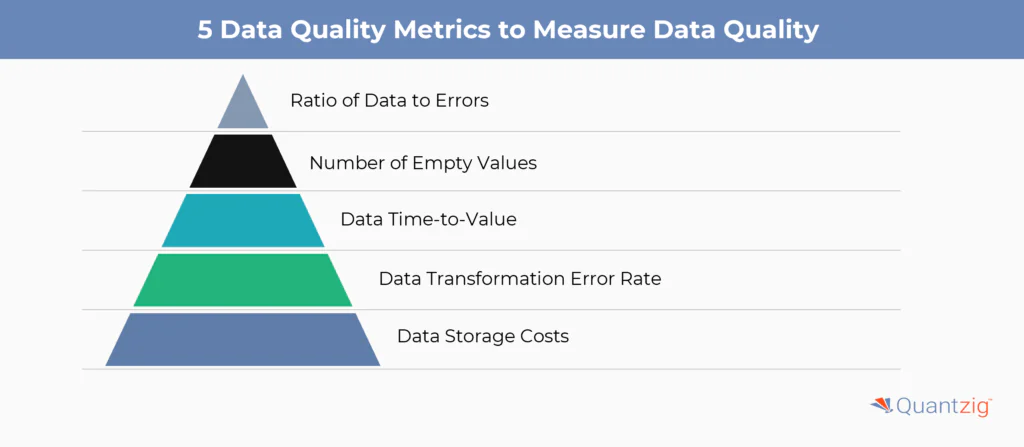
To ensure the reliability and trustworthiness of your data, it is crucial to measure its quality. Here are five data quality metrics that can help you assess the health of your data:
1. Ratio of Data to Errors
This indicator keeps track of how many known data errors there are in the total data set. It provides a clear approach to assessing data quality by dividing the total number of errors by the total number of items. If you find fewer errors while the size of your data remains constant or increases, you know your data quality is improving.
2. Number of Empty Values
This metric counts the number of empty fields within a particular data set. It is a simple yet effective way to track data quality issues. Focus on fields that add significant value to the data set, such as zip codes or phone numbers.
3. Data Time-to-Value
This metric identifies how long it takes to gain in-depth insights from a large data set. While other factors influence data time-to-value, data quality is a significant contributor. If data transformations generate a lot of errors or require manual cleanup, it can indicate poor data quality.
4. Data Transformation Error Rate
This metric keeps track of how often a data transformation operation fails. It is essential to monitor data transformation errors to ensure that data is accurately converted and processed.
5. Data Storage Costs
This metric monitors storage costs when the amount of data used remains the same or decreases. If storage costs increase, it may indicate that a significant portion of the data stored has poor quality, making it unusable. By tracking these data quality metrics, you can identify areas for improvement and ensure that your data is reliable and trustworthy.
By tracking these data quality metrics, you can identify areas for improvement and ensure that your data is reliable and trustworthy.
Quantzig’s Expertise in Data Quality Management Framework for a US-Based E-commerce Retail Brand
Category | Details |
---|---|
Client Details | An e-commerce retailer based in the United States with annual revenue of $10bn+. |
Challenges Faced by The Client | The e-commerce retailer faced significant operational inefficiencies due to manual processes, stakeholder dependencies, and extended turnaround times for customer queries. |
Solutions Offered by Quantzig | Quantzig offered solutions that included identifying high-impact processes for automation, creating a centralized platform with robust access controls and alerts, and developing a centralized AI-based auto-response platform leveraging natural language processing (NLP) to enhance customer service capabilities. |
Impact Delivered | Improved transparency across stakeholders and reduced errors. Process execution was accelerated by 3x. Customer satisfaction increased by 70%, with 95% of customer queries responded to in less than 5 seconds. A 40% uplift in new customer acquisition. |
Client Details
An e-commerce retailer based in the United States was struggling with inefficient manual processes and stakeholder dependencies, hindering their ability to respond quickly to market changes and customer needs.
Challenges in Data Quality Management Faced by the Client
The e-commerce retailer faced significant operational inefficiencies due to manual processes and stakeholder dependencies. These processes were error-prone, time-consuming, and hindered the organization’s ability to respond swiftly to market changes and customer needs. The client struggled with extended turnaround times for customer queries, which negatively impacted customer satisfaction and loyalty. Additionally, they faced challenges in initiating their digital transformation journey, including identifying the right starting point and selecting appropriate technologies.
Data Quality Management Solution Offered by Quantzig for the Client
- Quantzig played a pivotal role in the client’s digital transformation journey by first identifying high-impact processes that needed automation. Through a meticulous assessment, Quantzig mapped out the stakeholders involved in these processes and delineated their respective responsibilities.
- Subsequently, they spearheaded the creation of a centralized platform equipped with robust access controls, alerts, and other essential functionalities. This platform served as a unified hub, ensuring that accurate and up-to-date information became readily accessible across teams and processes.
- By establishing a single source of truth, Quantzig enabled streamlined operations, reduced errors, and facilitated faster decision-making, ultimately alleviating the bottlenecks that had been hindering the client’s operational efficiency.
- Quantzig further revolutionized the client’s customer service capabilities by developing a centralized AI-based auto-response platform. This cutting-edge system harnessed the power of natural language processing (NLP) to swiftly decode and understand customer queries. Leveraging a comprehensive backend Q&A repository, the platform provided standardized responses to 95% of the queries in less than 5 seconds, ensuring rapid and consistent interactions with customers.
- For more complex queries, the system intelligently directed them to customer care executives for personalized assistance. This platform’s accessibility across various devices, including laptops, mobiles, and iPads, made it effortless for customers to engage with the brand, significantly enhancing the overall customer experience and bolstering the client’s reputation for responsiveness and reliability.
Impact Delivered Using Quantzig’s Expertise
The efficient data quality management led to significant improvements in the client’s operations and customer satisfaction. Key outcomes included:
- Centralized processes: Improved transparency across stakeholders and reduced errors.
- Faster process execution: Process execution was accelerated by 3x, enabling the client to respond more quickly to market changes and customer needs.
- Customer satisfaction: Customer satisfaction increased by 70%, with 95% of customer queries responded to in less than 5 seconds.
- New customer acquisition: The client saw a 40% uplift in new customer acquisition, driven by enhanced customer experiences and responsiveness.
Quantzig’s data quality management solutions empowered the e-commerce retailer to streamline operations, enhance customer experiences, and drive business growth. By leveraging automation, data-driven insights, and agility, the client was able to stay competitive and thrive in a rapidly evolving market.
Also Read: Understanding Omnichannel Marketing Analytics for Businesses
Get started with your complimentary trial today and delve into our platform without any obligations. Explore our wide range of customized, consumption driven analytical solutions services built across the analytical maturity levels.
Start your Free Trial TodayHow Can Quantzig’s Data Quality Management Solutions Help B2B Businesses?
Quantzig’s comprehensive data management solutions empower organizations to harness the full potential of their data. Their portfolio includes a range of services designed to streamline data infrastructure, enhance accessibility, and ensure data integrity. Key offerings include:
- Data Engineering: Leveraging state-of-the-art industry frameworks, Quantzig simplifies data stacks, optimizing data flow and ensuring data integrity.
- Data Strategy Consulting: Quantzig crafts tailored analytics roadmaps, identifies process gaps, and introduces plug-and-play business acceleration modules to drive sustainable growth and informed decision-making.
- Business Analytics Services: Quantzig specializes in identifying solutions to complex business problems, enhancing performance across the entire value chain, and driving informed decisions.
- Data Visualization & Reporting: Intuitive, three-click visualizations simplify decision-making, providing actionable insights and driving efficiency across the organization.
- Business Process Automation: Custom apps streamline and automate business processes, reducing manual intervention and unlocking substantial time and cost savings.
By investing in Quantzig’s data management solutions, organizations can unlock the full potential of their data, drive innovation, and maintain a competitive edge in the market.
Also Read: Guide to Advanced Marketing Analytics Solutions and Its Business Benefits
Experience the advantages firsthand by testing a customized complimentary pilot designed to address your specific requirements. Pilot studies are non-committal in nature.
Request a Free PilotConclusion
In conclusion, data quality management is a critical component of any business strategy, as it ensures the accuracy, completeness, and consistency of data. By implementing effective data quality management practices, businesses can improve data quality, reduce errors, and increase efficiency. This article has highlighted the importance of DQM, the five pillars of DQM, and the techniques and tips for ensuring a successful process. By following these best practices, businesses can ensure that their data is reliable, trustworthy, and ready for analysis.