Written By: Associate Vice President, Analytics and Data Strategy, Quantzig.
Table of Contents
- What is Data Quality Automation?
- What is automated data quality?
- How does automated data quality work?
- The components of an automated data quality solution
- The benefits of automated data quality
- Choosing the Right Data Quality Automation Solution
- How Quantzig helps with Data Quality Automation Solution?
- How to Enhance Customer Data Integrity
- The Future of Automated Data Quality Management
What is Data Quality Automation?
Data quality automation is the process of using technology to streamline and optimize data quality management. It involves implementing automated processes to detect, correct, and monitor data quality issues in real-time, reducing the need for manual intervention and improving the overall reliability and consistency of data.
The State of Data Quality so far: Growth and Complexity
In the data ecosystem, the importance of Data Quality & Governance Services has continued to grow, as organizations increasingly rely on data-driven decision-making and analytics to drive business success. However, the complexity of data management has also increased, with the proliferation of data sources, formats, and volumes. Manual data quality processes have become increasingly unsustainable, leading to a growing need for automated solutions.
Manual data quality: how it works and why it’s not sustainable
Traditionally, data quality management has been a manual process, involving teams of data analysts and specialists who review data, identify issues, and implement fixes. This approach can be time-consuming, error-prone, and resource-intensive, especially as the volume and complexity of data continue to grow.
Book a demo to experience the meaningful insights we derive from data through our analytical tools and platform capabilities. Schedule a demo today!
Request a Free DemoWhat is automated data quality?
Automated data quality is the use of technology to automate the processes of data validation, cleansing, and monitoring. It involves the implementation of rules, algorithms, and machine learning models to detect and correct data quality issues in real-time, without the need for manual intervention.
Key Features of Automated data quality
By implementing an automated data quality framework, organizations can realize significant benefits, including:
- Reduced manual effort in data quality management by 60-70%
- Improved data quality and consistency, leading to better insights and decision-making
- Increased efficiency and productivity of data management teams
- Enhanced trust and confidence in data among business stakeholders
How does automated data quality work?
Automated data quality solutions typically consist of several key components, including:
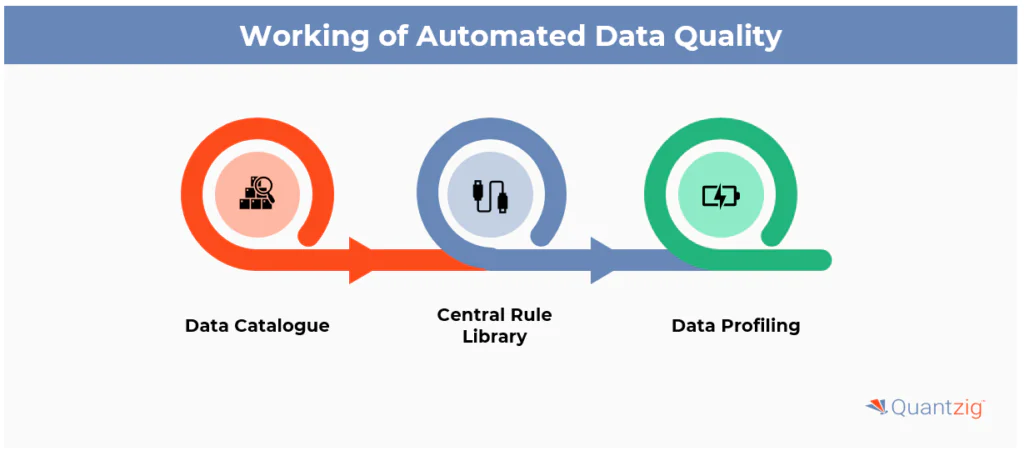
- Data Catalogue: A centralized repository that catalogues and tracks all data assets, their metadata, and their relationships.
- Central Rule Library: A library of pre-defined rules and algorithms for data validation, cleansing, and monitoring, which can be customized and applied across the organization.
- Data Profiling: Automated processes that analyze data to identify patterns, anomalies, and quality issues, and provide insights to guide the development of data quality rules.
The components of an automated data quality solution
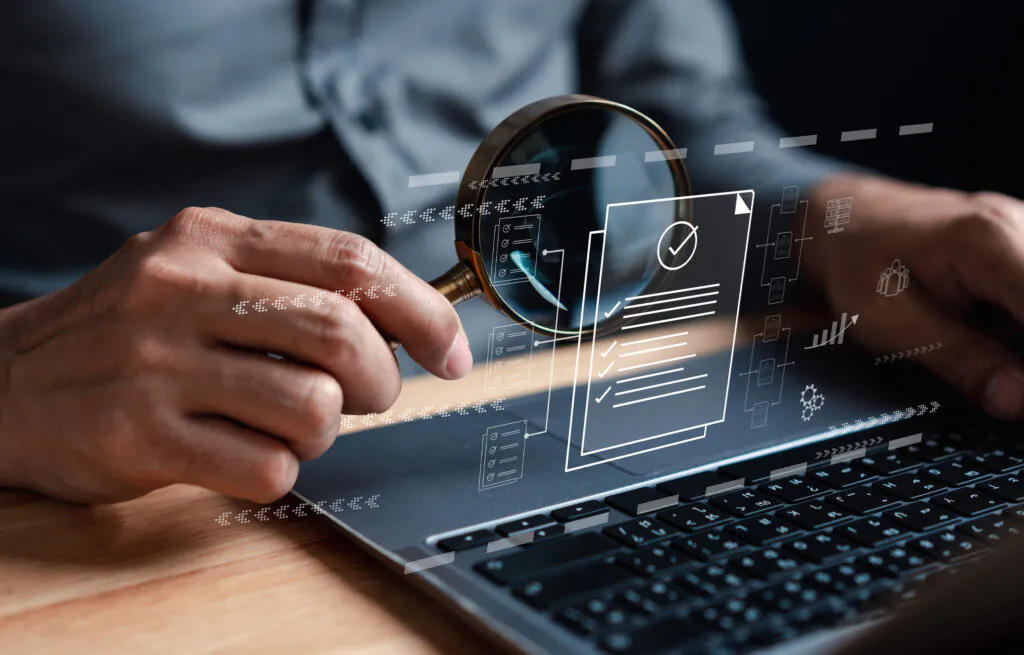
Data Catalog
A data catalog is a centralized repository that catalogues and tracks all data assets within an organization, including their metadata, lineage, and relationships. This helps to ensure that data quality rules and processes are applied consistently across the organization.
Central Rule Library
The central rule library is a collection of pre-defined rules and algorithms for data validation, cleansing, and monitoring. These rules can be customized and applied across the organization, ensuring consistent data quality standards and reducing the need for manual intervention.
Data Profiling
Data profiling is the process of analyzing data to identify patterns, anomalies, and quality issues. Automated data profiling tools can provide valuable insights that guide the development of data quality rules and help to ensure that the right rules are in place to address the most critical data quality issues.
Experience the advantages firsthand by testing a customized complimentary pilot designed to address your specific requirements. Pilot studies are non-committal in nature.
Request a Free PilotThe benefits of automated data quality for Data Streamlining
Automated data quality, or Data Quality Automation, offers several benefits for organizations. By using AI-driven DQM automation, businesses can enhance their data quality management (DQM) processes. Here are some key benefits:
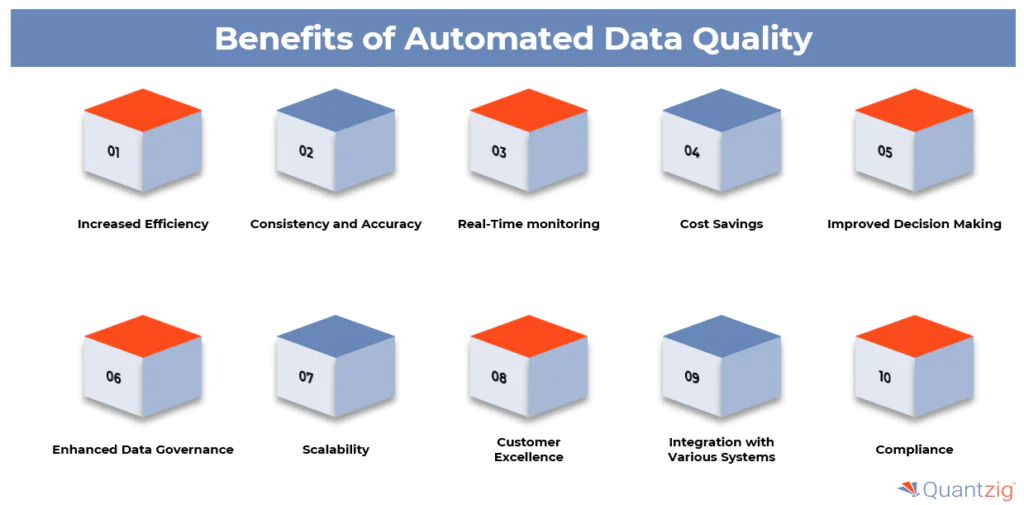
- Increased Efficiency: Automated data quality rules and processes handle large volumes of data quickly, reducing the time and effort spent on manual tasks.
- Consistency and Accuracy: AI-driven DQM automation applies uniform rules to all data, ensuring consistent and accurate results, reducing human error.
- Real-Time Monitoring and Alerts: Continuous monitoring identifies and flags issues in real-time, allowing for immediate resolution and minimizing business impact.
- Cost Savings: Automation reduces the need for manual intervention, leading to significant cost savings and preventing costly mistakes due to poor data quality.
- Improved Decision-Making: High-quality data supports better business decisions, providing accurate insights and effective strategies.
- Enhanced Data Governance: Automated tools enforce data governance policies across various data domains and data types, ensuring data integrity and regulatory compliance.
- Scalability: Automation easily scales to manage growing data volumes and complex environments, crucial for diverse datasets.
- Better Customer Experience: High-quality data enables personalized services, improving customer satisfaction and loyalty.
- Integration with Other Systems: Tools can integrate with data catalogs, data platforms, and data fabric systems, enhancing data processing and analysis workflows.
- Compliance and Risk Management: Ensuring high data quality helps meet regulatory requirements, reducing the risk of non-compliance and associated penalties.
The State of Data Quality survey highlights that automated tools for data profiling and managing multiple data sources are essential for maintaining high data standards and supporting organizational success.
Choosing the Right Data Quality Automation Solution
When selecting a data quality automation solution, it’s important to consider factors such as:
- Scalability and flexibility to handle growing data volumes and complexity
- Ease of use and integration with existing data infrastructure
- Customization and rule-building capabilities
- Reporting and analytics features to track data quality metrics
- Vendor reputation, support, and long-term roadmap
How Quantzig helps with Data Quality Automation Solution?
Optimizing Total Cost to Serve for a Leading Broadline Foods Distributor
Category | Description |
---|---|
Client Details | A leading broadline foods distributor in the US |
Client Challenges | Complex network operations, a diverse customer base, and the need for optimized cost-to-serve calculations to enable informed decision-making |
Solutions Provided | Developed a Total Cost to Serve model incorporating direct, business rule-driven, and algorithm-driven cost calculations at SKU levels and across distribution stages. Implemented a one-stop control tower to monitor cost and time throughout operations. |
Impact Achieved | Identified high-cost customers, SKUs, routes, geographies, and seasons. Facilitated efficiency initiatives and enhanced decision-making, leading to substantial cost savings and optimized operations. |
Overview
A leading broadline foods distributor in the US faced significant challenges in managing their complex and intense network operations. Serving a diverse set of customers with various product types, the distributor needed to optimize the total cost to serve customers to make informed decisions about marketing, discounts, rewards, promotions, market share optimization, and collaborative demand planning.
Challenges Faced by Client
The distributor’s operational landscape required accurate calculations of the total cost to serve customers at SKU levels and different stages of the distribution network. The main challenges included:
- Managing diverse and complex network operations
- Identifying cost drivers across customers, SKUs, routes, geographies, and seasons
- Implementing efficiency initiatives based on cost analysis
Solutions Delivered using Quantzig’s Data
Quantzig assisted the distributor in building a comprehensive Total Cost to Serve model. This model involved:
- Calculations, appropriations, and attribution of costs through direct, business rule-driven, or algorithm-driven methods
- Analysis of costs at SKU levels and different distribution network stages
- Development of a one-stop control tower to monitor both cost and time across network operations
Impact Delivered
The implementation of the Total Cost to Serve model resulted in significant improvements, including:
- Enhanced ability to analyze cost and time across network operations
- Identification of high-cost customers, SKUs, routes, geographies, and seasons
- Improved decision-making for efficiency initiatives, leading to cost savings and optimized operations
Through Quantzig’s solutions, the leading broadline foods distributor achieved a comprehensive understanding of their cost structures, leading to more informed decisions and greater operational efficiency. This case study demonstrates the importance of a well-implemented Total Cost to Serve model in complex distribution networks.
Get started with your complimentary trial today and delve into our platform without any obligations. Explore our wide range of customized, consumption driven analytical solutions services built across the analytical maturity levels.
Start your Free Trial TodayHow to Enhance Customer Data Integrity for Automated Data Management Platform?
Maintaining high-quality customer data is crucial for delivering personalized experiences, improving customer engagement, and driving business growth. Automated data quality solutions can help organizations enhance customer data integrity by:
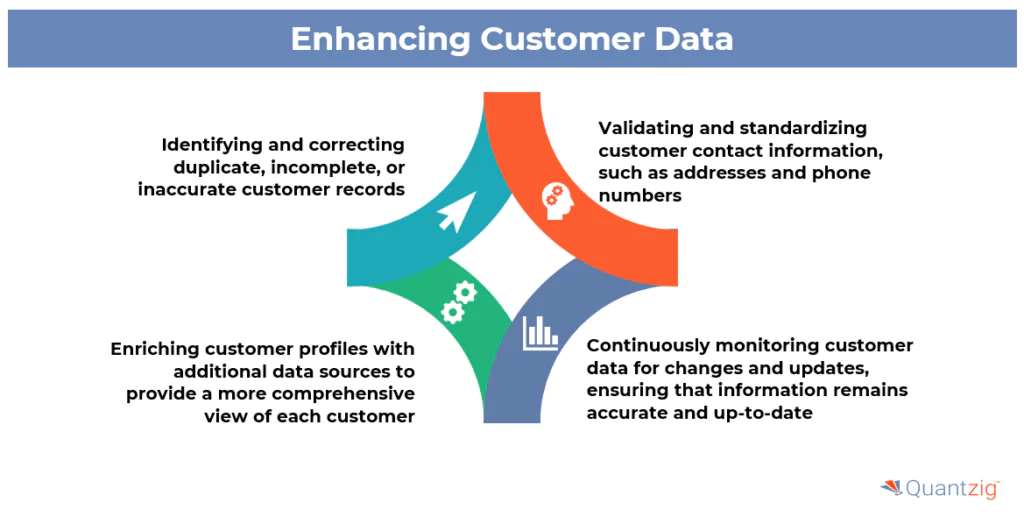
By implementing an automated data quality framework, organizations can ensure that their customer data is accurate, consistent, and reliable, enabling them to make more informed decisions and deliver better customer experiences.
The Future of Automated Data Quality Management
The future of automated Data Quality Management (DQM) is poised for significant advancements, driven primarily by AI-driven DQM automation. AI will enhance data quality checks, reducing the need for manual intervention and enabling real-time monitoring. By integrating with data catalogs, automated DQM tools will provide a comprehensive view of data assets, enhancing governance across various data domains and data types. Advanced data profiling techniques and dynamic data quality rules will allow organizations to better understand their data and identify quality issues more effectively. Regular State of Data Quality surveys will help organizations benchmark their efforts, providing valuable insights into industry trends and best practices.
Unified data platforms and data fabrics will facilitate seamless data integration and management across multiple data sources, ensuring consistency and reliability throughout the data lifecycle. Data Quality Automation will become increasingly scalable, capable of handling large datasets and maintaining high standards in complex ecosystems. Real-time data quality management will become the norm, enabling organizations to address issues as they arise and minimize the impact of poor data quality on operations and decision-making. These advancements will collectively transform the landscape of DQM, making it more efficient, comprehensive, and proactive.