Since the invention of Digital Twin, the integration of the digital twin technology and predictive analytics has transformed industrial processes. These cutting-edge technologies, when combined, enable real-time decision-making, process optimization, and enhanced efficiency. This blog explores how digital twin predictive models and AI-driven predictive analytics reshape industries by enabling predictive maintenance, asset performance prediction, and smart manufacturing.
Book a demo to experience the meaningful insights we derive from data through our analytical tools and platform capabilities. Schedule a demo today!
Request a Free DemoTable of Contents
Predictive Analytics: A Game-Changer
Predictive analytics involves the use of historical and real-time data, often enhanced by AI, to forecast future events. When integrated with digital twin data analytics, predictive analytics empowers organizations to predict failures, optimize operations, and improve asset longevity.
The Synergy: Digital Twin Meets Predictive Analytics
Combining digital twin technology with predictive analytics creates a robust ecosystem for industrial digital twin analytics. This synergy offers:
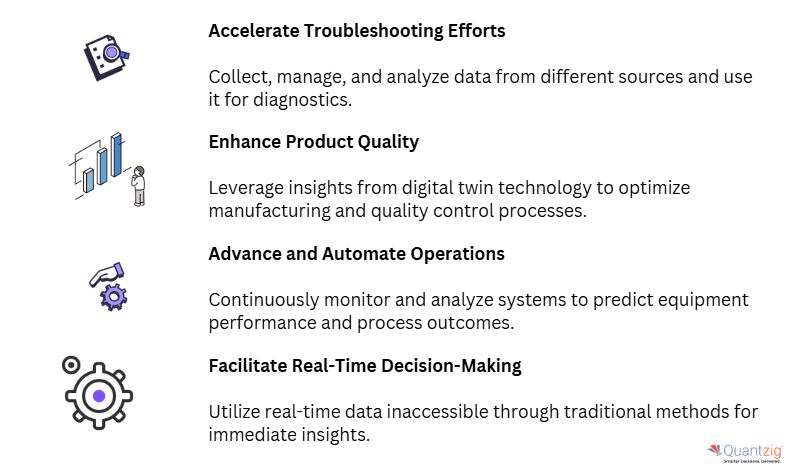
Predictive Maintenance with Digital Twin
Leverage digital twin technology to proactively identify potential equipment issues before they lead to costly failures. By simulating real-world conditions and analyzing historical and real-time data, businesses can minimize downtime and improve reliability.
Real-Time Predictive Analytics
Harness live data streams combined with predictive analytics to make informed, data-driven decisions instantly. This approach enables early detection of anomalies, empowering teams to act swiftly and efficiently.
Digital Twin for Process Optimization
Utilize digital twins to simulate and analyze operational workflows, identifying bottlenecks and opportunities for improvement. This leads to optimized processes, enhanced productivity, and reduced operational costs.
Asset Performance Prediction with Digital Twin
Predict the performance of assets with unparalleled accuracy by integrating digital twin models. These insights help maximize asset utilization, extend lifespan, and minimize maintenance costs.
Smart Manufacturing with Digital Twins
Enable advanced manufacturing systems to become more autonomous and adaptive. Digital twins empower facilities to respond dynamically to changes in demand, resource availability, and operational challenges, paving the way for intelligent and efficient production environments.
Benefits of Integrating Digital Twin and Predictive Analytics
Benefit | Description |
---|---|
Cost Reduction | Minimized downtime and maintenance costs through predictive maintenance. |
Enhanced Operational Efficiency | Real-time insights into process performance enable better resource utilization. |
Improved Decision-Making | Predictive insights from digital twins provide actionable intelligence. |
Increased Asset Lifespan | Accurate asset performance prediction with digital twin prevents unnecessary wear and tear. |
Risk Mitigation | Early detection of potential issues reduces operational and safety risks. |
Key Applications in Industry 4.0
1. Predictive Maintenance
Digital twin predictive maintenance leverages real-time data to forecast equipment failures, enabling timely intervention. By integrating digital twin modeling for predictive forecasting, industries can avoid costly unplanned downtime.
Example: A manufacturing plant uses IoT-enabled digital twins to monitor the health of machinery, predicting when parts will fail based on historical and current data.
2. Process Optimization
Digital twin for process optimization simulates different operational scenarios, allowing businesses to identify the most efficient workflows.
Example: A refinery uses digital twin simulation to optimize production lines, improving output while reducing energy consumption.
3. Asset Performance Prediction
Through industrial digital twin analytics, businesses can forecast asset performance under various conditions, ensuring they operate at peak efficiency.
Example: Wind turbines equipped with digital twin predictive models analyze environmental data to predict power generation efficiency.
4. Smart Manufacturing
Smart manufacturing with digital twins integrates AI and IoT to create adaptive production environments. Predictive analytics enhances automation, reducing human intervention.
Example: Automotive factories use digital twin predictive maintenance to optimize robotic assembly lines, ensuring continuous operation.
How to Implement Digital Twin and Predictive Analytics?
Steps for Implementation
Define Objectives
Begin by clearly identifying the specific processes, equipment, or systems that can benefit from digital twin-based predictive maintenance. Outline measurable goals, such as reducing downtime, improving equipment efficiency, or enhancing operational workflows. This step ensures alignment between business objectives and technological implementation.
Gather Data
Utilize IoT-enabled devices and sensors to collect real-time data from physical assets. Digital twins rely on a continuous flow of accurate and up-to-date data, capturing parameters such as temperature, pressure, vibration, and usage patterns. Ensure that data streams are reliable, securely transmitted, and stored for analysis.
Develop Models
Create sophisticated digital twin models by leveraging advanced AI and machine learning algorithms. These models simulate physical systems and predict potential issues by identifying patterns and trends. Training the model with historical data and real-time inputs enhances its accuracy and forecasting capabilities.
Integrate Systems
Seamlessly integrate digital twin data with existing predictive analytics platforms or enterprise systems. This integration enables cross-functional insights by combining data from multiple sources, creating a unified view of operations. Use APIs or middleware to facilitate smooth communication between systems.
Monitor and Optimize
Continuously track the performance of digital twin systems through real-time analytics dashboards. Use the insights to refine operations, improve accuracy, and address any inefficiencies. Regularly update the models to reflect changes in operational conditions or newly acquired data, ensuring long-term effectiveness and scalability.
Technologies Driving the Synergy
Technology | Role in Integration |
---|---|
IoT | Enables data collection for digital twin technology. |
AI and Machine Learning | Drives AI-driven predictive analytics and enhances model accuracy. |
Cloud Computing | Facilitates real-time analysis and scalability for digital twin simulation. |
Quantzig’s Expertise in Digital Twin and Predictive Analytics
At Quantzig, we empower businesses to unlock the full potential of digital twin technology combined with predictive analytics, revolutionizing operations in the Industry 4.0 era. Our solutions drive real-time predictive insights, streamline process optimization, and enable smarter, data-driven decision-making across industries.
By integrating cutting-edge technology with tailored strategies, we help organizations transition into the future of smart manufacturing, where efficiency and innovation redefine success. Partnering with Quantzig ensures your business stays ahead of the curve, leveraging these transformative tools to gain a competitive edge in the evolving industrial landscape.
Conclusion
The integration of digital twin technology and predictive analytics is reshaping Industry 4.0, unlocking unprecedented opportunities for businesses to innovate and thrive. This powerful combination enables real-time predictive analytics, providing actionable insights that allow organizations to identify potential issues, optimize processes, and make informed, data-driven decisions with unparalleled precision.
By leveraging digital twins, industries can simulate and analyze operations, uncover inefficiencies, and predict outcomes with accuracy, leading to enhanced productivity and reduced downtime. This transformative synergy paves the way for smart manufacturing, where adaptive systems respond dynamically to changes in demand, resource availability, and operational challenges—fostering an era of heightened efficiency and innovation.
Adopting this cutting-edge technology today positions businesses for sustained success, ensuring a decisive competitive edge in tomorrow’s rapidly evolving industrial landscape. Digital twin-powered predictive analytics is not just a tool; it’s a strategy to future-proof operations and achieve long-term growth in the age of Industry 4.0.