Table of Contents
Introduction to Propensity Matching Score Analytics
Propensity score matching (PSM) is a key statistical matching method designed to reduce selection bias in observational study analysis. By mimicking the conditions of a randomized controlled trial (RCT), PSM enhances causal inference techniques and enables valid comparisons between treatment groups while controlling for confounding variables. This article delves into the rationale, methods, advantages, limitations, and applications of propensity score matching in clinical and non-experimental data analysis.
Book a demo to experience the meaningful insights we derive from data through our analytical tools and platform capabilities. Schedule a demo today!
Request a Free DemoWhat is Propensity Score Matching?
Propensity score matching (PSM) is a quasi-experimental design method used in non-experimental data analysis to balance covariates and estimate treatment effects accurately. The propensity score, derived using techniques like logistic regression, represents the probability of receiving a treatment given observed baseline characteristic. By matching individuals with similar propensity scores, this method reduces selection bias and enables researchers to compare outcomes across groups under similar conditions.
In the absence of randomization, propensity score methods create an “apples-to-apples” comparison, often leveraging propensity score weighting or stratification to further enhance covariate balancing.
Rationale for Propensity Score Analysis
Propensity score analysis addresses the challenge of confounding variables in observational studies. Unlike RCTs, observational studies lack random allocation, leading to an imbalance in baseline characteristics. By estimating propensity scores, researchers can balance these covariates across treatment and control groups, achieving a structure akin to randomization. This reduces bias and allows for robust treatment effect estimation.
When the propensity scores of subjects are balanced, the distribution of baseline characteristics becomes similar between treated and untreated groups. This ensures that differences in outcomes are due to the treatment itself, rather than underlying confounders.
Methods of Propensity Score Analysis
The following are widely used statistical matching methods for propensity score analysis:
Method | Description |
---|---|
Propensity Score Matching | Matches treated and untreated individuals with similar propensity scores to compare outcomes. |
Stratification on Propensity Score | Divides subjects into strata based on propensity scores and compares outcomes within each stratum. |
Inverse Probability Weighting (IPW) | Weighs individuals by the inverse of their propensity scores to create a pseudo-population for analysis. |
Covariate Adjustment | Includes propensity scores as covariates in regression models for estimating treatment effects. |
The choice of method depends on the research objectives, data structure, and the degree of overlap in propensity scores between groups.
What are the Advantages and Limitations of Propensity Matching Score Analytics?
Advantages:
- Reduces Selection Bias: By balancing covariates, PSM ensures unbiased comparisons and enhances causal inference.
- Improves Transparency: PSM results are often presented using visualizations and interactive dashboards, simplifying interpretation.
- Supports Generalizability: PSM extends findings to broader populations, including subgroups often excluded from RCTs.
- Enhances Observational Study Analysis: It complements RCTs, refining randomization and improving external validity.
- Enables Robust Insights: By focusing on covariate balancing, researchers can effectively gauge the impact of treatments.
Limitations:
- Relies on Observed Variables: PSM adjusts only for measured covariates; unmeasured confounders can still bias results.
- Sample Size Dependency: Requires significant overlap between treatment and control groups, limiting its use in small datasets.
- Complex Matching Algorithms: The choice of matching algorithms (e.g., nearest neighbor, optimal matching) impacts results and requires expertise.
- Assumes No Unmeasured Confounding: Violations of this assumption may lead to invalid conclusions.
Despite its limitations, PSM remains a cornerstone of propensity score analysis in observational research.
In summary, while PSM offers several advantages in clinical research, it has important limitations related to hidden bias, sample size requirements, and the need for careful implementation. Integrating PSM with randomized trials can help leverage its strengths while maintaining the robustness of randomization.
Experience the advantages firsthand by testing a customized complimentary pilot designed to address your specific requirements. Pilot studies are non-committal in nature.
Request a Free PilotWhat are the Applications of Propensity Matching Score Analytics?
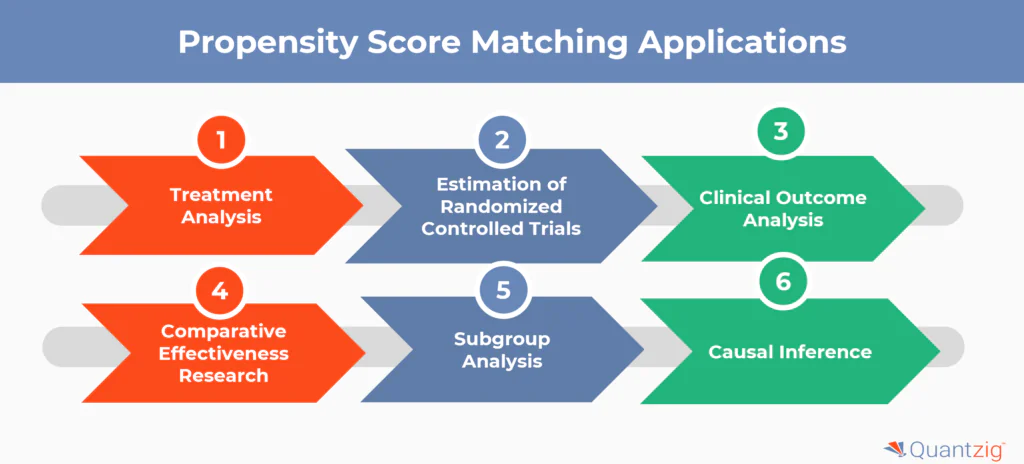
1. Treatment Effect Analysis
PSM is extensively used for treatment effect estimation in observational studies, enabling researchers to compare the impact of interventions on patient outcomes while controlling for confounding variables.
2. RCT Complementation
Propensity score methods in healthcare complement RCTs by accounting for non-compliance and improving external validity. This approach helps researchers estimate real-world treatment effects.
3. Clinical Outcome Analysis
PSM facilitates the comparison of clinical outcomes between groups in observational studies where randomization is impractical. By balancing covariates, researchers ensure valid comparisons.
4. Comparative Effectiveness Research
In comparative effectiveness research, PSM is a powerful tool for evaluating the relative benefits and risks of different interventions in real-world settings.
5. Subgroup Analysis
PSM helps identify and analyze specific patient subgroups to assess differential treatment effects. This is particularly valuable in personalized medicine.
6. Causal Inference in Marketing
In marketing, propensity score applications are used to evaluate campaign effectiveness by balancing covariates such as customer demographics and purchase history.
In summary, propensity score matching has become a widely adopted statistical technique in clinical research, enabling researchers to leverage large, complex datasets to generate meaningful insights, compare treatment groups, and draw more robust causal conclusions – complementing the insights from randomized controlled trials.
Get started with your complimentary trial today and delve into our platform without any obligations. Explore our wide range of customized, consumption driven analytical solutions services built across the analytical maturity levels.
Start your Free TrialConclusion
Propensity score matching is a pivotal technique in observational study analysis, offering robust solutions for reducing selection bias and enhancing causal inference. By employing methods like logistic regression for propensity scores, stratification, and weighting, PSM ensures covariate balancing and accurate treatment effect estimation. Despite its reliance on measured variables and large sample requirements, PSM remains indispensable in clinical research, healthcare, and beyond.
Leverage Quantzig’s expertise in propensity score matching and other advanced statistical matching methods to derive actionable insights from your data. Schedule a free demo today and experience the transformative power of analytics tailored to your unique needs.