Table of Contents
Introduction to Propensity Modeling
The media and entertainment industry faces significant challenges in predicting customer behavior and driving revenue growth. Technological advancements and digital innovations have transformed the landscape, leading to high churn rates and dissatisfied customers. To address these issues, media and entertainment companies are increasingly turning to data-driven strategies, including customer segmentation and propensity modeling.
Propensity models can help these companies predict customer actions, such as the likelihood to buy, churn, or unsubscribe. By leveraging these insights, they can develop targeted campaigns, personalized offers, and effective retention strategies to boost revenue and customer loyalty. Adopting a data-driven, customer-centric approach empowers media and entertainment firms to navigate the evolving industry dynamics and achieve sustainable growth.
Book a demo to experience the meaningful insights we derive from data through our analytical tools and platform capabilities. Schedule a demo today!
Request a Free DemoWhat is Propensity Modeling?
Propensity modeling is a statistical technique used to predict the likelihood of a customer taking a specific action, such as making a purchase, subscribing to a service, or churning. It involves analyzing historical data on customer behavior and demographics to identify patterns that can be used to forecast future actions. By understanding a customer’s propensity to take certain actions, businesses can tailor their marketing strategies and campaigns to target the right customers, improve customer retention, and ultimately drive revenue growth.
Fundamentals of Propensity Modeling
Propensity modeling is a statistical technique used to predict the likelihood of a specific customer action, such as making a purchase, churning, or responding to a marketing campaign. By analyzing historical data and behavioral patterns, businesses can identify key factors influencing customer decisions and develop targeted strategies to improve engagement, conversion rates, and retention.
Key Fundamentals of Propensity Modeling:
-
Data Collection
Involves gathering historical customer data, including demographics, interactions, and transaction history.
-
Feature Engineering
Identifies and selects the most relevant variables that influence customer behavior.
-
Model Selection
Uses statistical and machine learning techniques like logistic regression or decision trees to predict outcomes.
-
Probability Scoring
Assigns a likelihood score to each customer based on predicted behavior.
-
Segmentation and Targeting
Helps categorize customers for personalized marketing efforts.
-
Continuous Optimization
Requires ongoing model refinement using new data for improved accuracy.
Why Should Optimizers Care About Propensity Modeling?
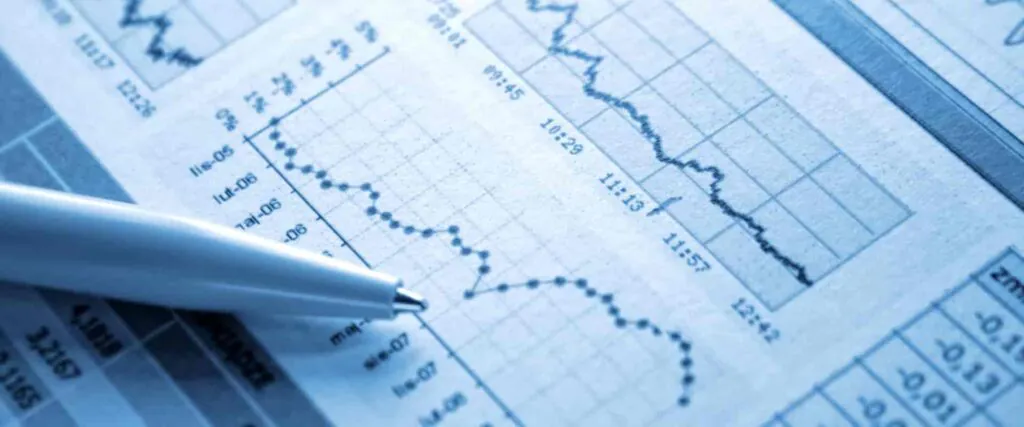
Propensity modeling is crucial for optimizers as it enables them to predict customer behavior, identify high-value customers, and tailor marketing strategies to maximize ROI. By leveraging propensity modeling, optimizers can create targeted campaigns that drive conversions, reduce churn, and enhance customer engagement, ultimately leading to improved business outcomes.
Top Propensity Models for Revenue Growth in Media
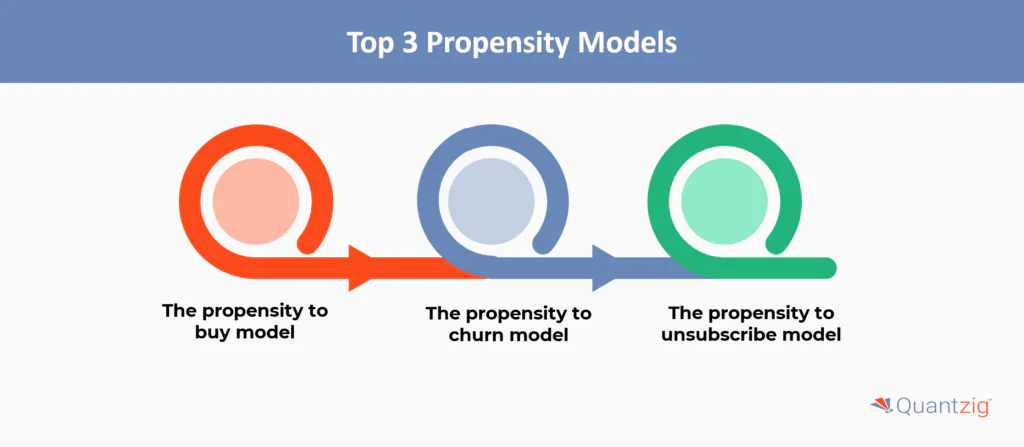
1. The propensity to buy model – How likely are your customers/visitors to make a purchase or turn into loyal customers?
Insights such as these can help media and entertainment companies to identify customers with lower propensity scores and target them through personalized offers.
2. The propensity to churn model – Who are your ‘at-risk’ customers?
Identification of at-risk customer groups with high propensity scores can help media and entertainment companies reduce churn through win-back campaigns. By adopting a churn driven approach to propensity modeling, media companies can also go a step ahead and assign account concierges to help customers reconnect with the company’s core value proposition.
3. The propensity to unsubscribe model – How likely are customers to unsubscribe from your services?
Insights into customer groups that are more likely to unsubscribe from services can help media and entertainment companies analyze their behavior and develop special offers and discounts to reinforce the value of remaining a subscriber. This propensity modeling approach also helps businesses to take the right action at the right time without impacting user experience.
Also Read: Track Business Progress with Marketing Analytics Dashboard
Experience the advantages firsthand by testing a customized complimentary pilot designed to address your specific requirements. Pilot studies are non-committal in nature.
Request a Free PilotBenefits of Propensity Modeling Techniques
- Targeted Marketing: Propensity modeling allows you to identify customers most likely to respond to specific marketing campaigns, enabling you to allocate resources more efficiently.
- Improved Customer Retention: By predicting which customers are at risk of churning, you can proactively take steps to retain them through personalized offers and interventions.
- Enhanced Personalization: Propensity scores can be used to deliver highly personalized experiences, content, and recommendations that resonate with each customer’s unique preferences and behaviors.
- Increased Revenue: Accurate propensity models help you maximize revenue by identifying high-value customers, cross-sell and upsell opportunities, and optimal pricing strategies.
- Reduced Costs: Propensity modeling enables you to focus your marketing efforts on the customers most likely to convert, minimizing wasted spend on less promising prospects.
How to Build a Customer Propensity Model?
Here are the key steps to build an effective customer propensity model:
- Define the Business Objective: Clearly identify the specific business goal you want to achieve, such as predicting customer churn, purchase likelihood, or cross-sell potential. This will guide the model development process.
- Collect Relevant Data: Gather historical customer data from various sources, including customer transactions, demographics, behavior, and interactions. Ensure the data is clean, consistent, and representative of your target customer base.
- Explore and Prepare the Data: Analyze the data to understand patterns, identify key variables, and handle any missing values or outliers. Feature engineering can help create new predictive variables from the raw data.
- Split the Data: Divide the dataset into training and testing sets. The training set will be used to build the model, while the testing set will be used to evaluate its performance.
- Choose the Appropriate Modeling Technique: Select a suitable machine learning algorithm based on the business objective and the nature of the data. Common techniques for propensity modeling include logistic regression, decision trees, random forests, and gradient boosting.
- Train the Model: Use the training data to fit the chosen algorithm and tune the model’s hyperparameters to optimize its performance.
- Evaluate the Model: Assess the model’s accuracy, precision, recall, and other relevant metrics using the testing dataset. Ensure the model is performing well and meeting the defined business objectives.
- Deploy the Model: Integrate the trained model into your business processes, such as marketing campaigns, customer service, or product recommendations. Continuously monitor the model’s performance and update it as needed to maintain its effectiveness.
- Iterate and Refine: Regularly review the model’s performance and gather feedback from end-users. Incorporate new data sources, adjust the modeling approach, and fine-tune the model to improve its predictive power over time.
Key considerations when building customer propensity models:
- Ensure data quality and representativeness
- Experiment with different modeling techniques
- Interpret the model’s feature importance to gain insights
- Continuously monitor and update the model for optimal performance
- Integrate the model into your business workflows for maximum impact
By following these steps, you can develop robust customer propensity models that drive actionable insights and support your organization’s strategic decision-making.
How to Use Your Propensity Model for Smarter Experimentation?
Here are the key ways to leverage a propensity model for smarter experimentation:
- Targeted Audience Selection: Use the propensity scores to segment your audience and target the experiments towards the customers/prospects most likely to respond positively. This allows you to focus your resources on the high-potential segments.
- Personalized Experiences: Incorporate the propensity scores into your personalization algorithms to deliver tailored experiences, offers, and content to each customer based on their predicted likelihood to convert, churn, or take other actions.
- Lift Analysis: Compare the performance of your test and control groups, factoring in the propensity scores. This allows you to measure the true incremental impact of your experiment, beyond just the baseline differences between the groups.
- Predictive Optimization: Feed the propensity scores into your optimization algorithms to dynamically adjust your campaigns, website content, product recommendations, and other experiences in real-time to maximize the desired outcomes.
- Experiment Design: Use the propensity model to inform the design of your A/B and multivariate tests. For example, you can stratify your randomization by propensity score buckets to ensure balanced test groups.
- Causal Inference: Leverage propensity score matching or weighting techniques to estimate the causal effect of your treatments, accounting for potential confounding factors in observational data.
- Exploration and Hypothesis Generation: Analyze the drivers of your propensity model to uncover new hypotheses about customer behavior that can be tested through further experimentation.
By integrating propensity modeling into your experimentation workflow, you can make your tests more targeted, personalized, and statistically rigorous – ultimately driving greater ROI from your optimization efforts.
Experience the advantages firsthand by testing a customized complimentary pilot designed to address your specific requirements. Pilot studies are non-committal in nature.
Request a Free PilotHow Quantzig’s Propensity Modeling Boosted Sales for a Media Services Provider
Component | Description |
---|---|
Client | The Asian arm of a global media and entertainment company |
Challenges faced by the Client | – Failing to drive sales volumes and enhance brand visibility through promotional campaigns – Prior attempts to target customers based on their behavior and content consumption patterns had not delivered the desired results |
Solutions Offered by Quantzig | Quantzig’s propensity modeling experts developed a robust model to help the client: |
– Create and implement new strategies to drive ROI through targeted campaigns – Retain loyal customers year-over-year | |
Impact Delivered | – Empowered the client to target customer segments based on their needs and interests – Achieved double-digit growth in MROI – Witnessed a stellar response to the targeted promotional offers – Achieved a remarkable growth of over 200% increase in subscription rate compared to the previous year – The devised propensity model continues to be used to launch promotional offers for their subscription platforms and other services |
Also Read: Maximizing Marketing Budgets with Campaign ROI Analysis
Get started with your complimentary trial today and delve into our platform without any obligations. Explore our wide range of customized, consumption driven analytical solutions services built across the analytical maturity levels.
Start your Free Trial TodayWhy Choose Quantzig?
Quantzig’s expertise in propensity modeling empowers businesses to unlock the full potential of data analytics, offering tailored solutions for sales forecasting, lead scoring, and customer segmentation. Our advanced use of machine learning ensures real-time, data-driven insights for optimizing targeted campaigns and reducing churn rates.
At Quantzig, we leverage advanced customer segmentation analytics solutions to help media and entertainment companies to:
- Enhance CX and drive better campaign outcomes
- Leverage data from disparate sources and apply customer behavior analytics to better understand consumers
- Apply social listening to understand the impact of customer sentiments
Conclusion
Propensity score modeling, a powerful tool in data analytics, plays a critical role in understanding customer behavior through customer propensity modeling and predictive propensity modeling. By leveraging propensity models for marketing, businesses can conduct comprehensive propensity analysis to better predict customer actions. Advanced propensity modeling techniques and propensity modeling software enable companies to optimize sales forecasting, enhance customer segmentation, and improve lead scoring. With the integration of machine learning for propensity modeling, organizations can develop real-time models that drive targeted campaigns and predict customer churn. Ultimately, real-time propensity modeling transforms customer data into actionable insights for better decision-making.