Predictive analytics is transforming the healthcare industry, offering hospitals the ability to predict outcomes, optimize treatments, and enhance patient care. This data-driven approach leverages advanced statistical techniques, machine learning algorithms, and historical data to forecast future events, making it a vital tool in improving operational efficiency and patient outcomes. In today’s competitive healthcare landscape, hospitals are increasingly relying on predictive analytics to stay ahead, reduce costs, and provide personalized care.
This blog delves into why predictive analytics is crucial for healthcare institutions and how it can drive significant improvements in patient outcomes and hospital performance.
Our advanced analytics solutions help healthcare organizations to improve patient outcomes and reduce the cost of care. Want to know how? Request a FREE demo.
Table of Contents
Understanding Predictive Analytics in Healthcare
At its core, predictive analytics in healthcare involves analyzing historical and real-time patient data to forecast outcomes and guide decision-making processes. Data sources such as Electronic Health Records (EHRs), lab results, genetic data, wearable devices, and public health databases are used to generate these insights.
These predictions assist healthcare professionals in identifying patterns and anticipating future events, from diagnosing diseases early to managing hospital operations more effectively. The growing use of predictive analytics in healthcare is driven by its potential to not only address individual patient needs but also tackle broader challenges such as patient influx, resource management, and disease outbreaks.
Benefits of Healthcare Predictive Analytics
Predictive analytics is revolutionizing healthcare operations and patient management. Here are the primary benefits hospitals can achieve by leveraging predictive analytics solutions:
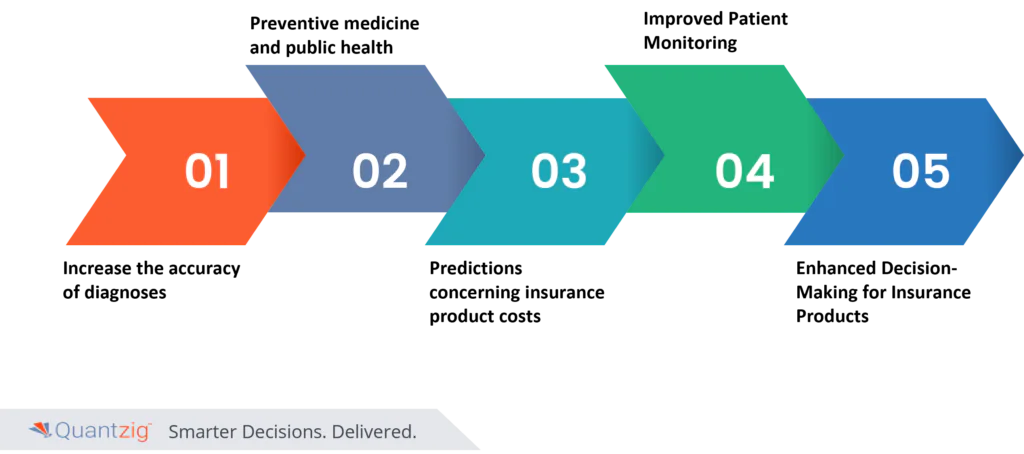
1. Improved Patient Outcomes
Predictive analytics plays a critical role in early intervention. By identifying risk factors and predicting the progression of diseases, healthcare providers can take preventive actions before a patient’s condition worsens. Hospitals that use predictive models to monitor at-risk patients have reported reduced mortality rates, fewer complications, and shorter hospital stays.
For example, predictive models that analyze vital signs, lab results, and patient history can help clinicians identify patients at risk of developing sepsis or other complications, enabling them to administer life-saving interventions sooner.
2. Personalized Treatment Plans
One-size-fits-all medical treatments are being replaced by personalized medicine, a data-driven approach that tailors treatments to individual patients based on their unique characteristics. Predictive analytics models allow physicians to assess how different treatments might impact a patient based on their medical history, genetic predispositions, and lifestyle factors.
This enables healthcare providers to create highly customized treatment plans that not only improve patient outcomes but also enhance patient satisfaction by aligning treatments with personal preferences and needs.
3. Operational Efficiency and Resource Allocation
Hospitals can leverage predictive analytics to optimize their operations, from managing bed occupancy to staffing requirements. By forecasting patient inflows and lengths of stay, hospitals can better allocate resources, reduce bottlenecks, and ensure staff availability during peak times. This level of operational foresight minimizes unnecessary costs and ensures that critical resources are available when needed most.
For instance, predictive models can analyze historical data to predict seasonal trends in hospital admissions, helping administrators plan and allocate resources more effectively during flu seasons or pandemic outbreaks.
4. Predictive Disease Management and Prevention
A significant benefit of predictive analytics is its ability to forecast disease outbreaks and help healthcare institutions prepare for surges in patient volume. Predictive models analyze data trends related to public health, climate, and even social determinants of health to predict the likelihood of disease outbreaks.
Hospitals that adopt these models can preemptively increase staffing, stock medical supplies, and implement patient triage processes to manage surges more effectively. This is particularly critical during global health crises like the COVID-19 pandemic, where predicting case numbers and resource needs became paramount.
5. Reduced Readmission Rates
Preventing unnecessary hospital readmissions is a critical issue for healthcare providers, as readmissions are both costly and detrimental to patient health. Predictive analytics models can help hospitals identify patients who are at high risk of readmission based on their medical history, social factors, and treatment outcomes.
By analyzing this data, hospitals can proactively manage these patients through follow-up appointments, monitoring programs, or remote health tools, ultimately reducing readmission rates and improving patient care quality.
6. Enhanced Risk Management
Predictive analytics models help hospitals in mitigating clinical and operational risks. Predicting adverse patient outcomes, such as complications during surgery or adverse reactions to medications, allows healthcare providers to minimize these risks by adjusting treatment plans or taking preventive measures.
Similarly, operational risks, such as equipment failures or supply shortages, can be anticipated and mitigated. Hospitals can proactively schedule equipment maintenance or stock up on critical supplies based on predictive models that forecast potential failures or supply chain disruptions.
7. Improved Patient Engagement and Satisfaction
Predictive analytics empowers hospitals to offer more personalized and proactive care, ultimately improving patient engagement. By providing patients with the right information at the right time—whether it’s a personalized treatment plan, medication reminder, or health forecast—hospitals can improve adherence to treatment plans and foster trust between patients and healthcare providers.
This proactive approach not only boosts patient outcomes but also enhances patient satisfaction and loyalty, as patients feel more cared for and understood by their healthcare providers.
Experience the advantages firsthand by testing a customized complimentary pilot designed to address your specific requirements. Pilot studies are non-committal in nature.
Request a Free PilotUse Cases of Predictive Analytics in Healthcare
Predictive analytics is being deployed in a variety of innovative ways across hospitals. Here are some use cases that highlight its potential:
1. Early Disease Detection
Hospitals use predictive analytics to forecast the likelihood of patients developing chronic diseases like diabetes, cardiovascular disease, or cancer. By assessing risk factors such as age, genetics, lifestyle habits, and lab results, physicians can intervene early, offering preventive treatments that can significantly alter the course of the disease.
2. Hospital Workflow Optimization
Hospitals are using predictive models to streamline workflows by forecasting daily or weekly patient admissions, allowing them to assign staff more efficiently. Predictive analytics is also being used to optimize surgery schedules, reducing patient wait times and improving operating room utilization.
3. Population Health Management
Predictive analytics models can assess population-level data to predict disease outbreaks or public health trends. Hospitals can partner with public health organizations to prevent large-scale health crises and allocate resources for vulnerable populations, such as ensuring flu vaccines are available for at-risk groups before flu season.
4. Reducing Emergency Room Crowding
Crowded emergency departments lead to suboptimal care, long wait times, and stressed staff. Hospitals can use predictive analytics to forecast ER traffic, allowing administrators to implement diversion strategies, prepare overflow areas, or adjust staffing based on the predicted patient volume.
Challenges in Implementing Predictive Analytics in Healthcare
Despite the clear benefits, hospitals face challenges in implementing predictive analytics solutions:
- Data Quality and Availability: Predictive models rely on vast amounts of high-quality data. Inconsistent data from different EHR systems or fragmented patient records can limit the accuracy of predictions.
- Integration with Existing Systems: Many hospitals struggle to integrate predictive analytics solutions with their existing IT infrastructure, limiting the full potential of predictive models.
- Privacy and Security Concerns: The handling of sensitive patient data comes with strict regulatory requirements. Ensuring patient data privacy while leveraging large datasets remains a challenge for hospitals.
- Adoption and Training: Many healthcare professionals are not familiar with the intricacies of data analytics. Hospitals need to invest in training and education to ensure clinicians can effectively use predictive models in their decision-making processes.
How Quantzig Helps Hospitals Harness Predictive Analytics
Quantzig is a leader in providing data-driven solutions to healthcare organizations, offering tailored predictive analytics models designed to improve both patient care and hospital operations. Our expertise lies in leveraging healthcare data to build customized solutions that align with hospitals’ unique needs.
At Quantzig, we help hospitals:
- Build predictive models that offer real-time insights into patient health and operational performance.
- Personalize treatment plans and enhance patient engagement through data analytics.
- Optimize resources, reduce costs, and improve hospital workflows using data-driven insights.
- Address challenges such as readmissions, disease management, and risk mitigation.
By partnering with Quantzig, healthcare organizations can unlock the full potential of predictive analytics and transform the way they deliver care.
Get started with your complimentary trial today and delve into our platform without any obligations. Explore our wide range of customized, consumption driven analytical solutions services built across the analytical maturity levels.
Start your Free TrialConclusion
Predictive analytics is rapidly becoming a cornerstone of modern healthcare. Its ability to enhance patient outcomes, optimize operations, and forecast health trends positions it as a game changer for hospitals. As the healthcare industry continues to evolve, hospitals that invest in predictive analytics will not only provide superior patient care but also achieve long-term operational success.
Predictive analytics in healthcare is revolutionizing the industry by providing predictive analytics for healthcare providers with powerful tools to enhance decision-making. By leveraging healthcare data analytics and predictive modeling in healthcare, providers can utilize healthcare analytics tools and advanced healthcare predictive analytics software to generate accurate data-driven healthcare predictions. These predictive healthcare solutions not only improve predictive analytics for patient care but also enhance predictive analytics for hospital management through insights into how predictive analytics improves patient outcomes, staffing needs, and predictive analytics for healthcare fraud detection. From predicting outcomes in chronic disease management to optimizing predictive analytics for emergency room staffing, the role of predictive analytics is critical. Furthermore, AI in healthcare predictive analytics strengthens these systems, ensuring better efficiency and improved outcomes across the board.
Partnering with experts like Quantzig can help hospitals seamlessly integrate predictive analytics into their systems, driving innovation and delivering data-driven, personalized care for patients.