Author: Associate Vice President, Analytics and Data Strategy, Quantzig.
Table of Contents
What is Predicting Data Analytics?
Within the IT industry, staying abreast of evolving trends is important for organizational growth and success. Data analytics has emerged as a transformative force, enabling IT businesses to harness the power of information for enhanced performance and strategic decision-making. Predicting Trends in the IT Industry is a crucial task that helps businesses stay ahead of the competition and make informed decisions. By leveraging advanced technologies such as artificial intelligence (AI) and machine learning (ML), organizations can gain valuable insights from large amounts of data.
In this analysis, we will explore the implementation, design, and testing phases of product containers, as well as their significance in the financial industry. Additionally, we will discuss the role of AWS in managing data domains and the importance of data lakes and enterprise data catalogs in organizing and accessing high-quality data. Furthermore, we will shed light into the concept of synthetic data and its applications in training AI/ML analytics systems. Let’s explore the current trends shaping the IT industry through the lens of analytic tools.
Book a demo to experience the meaningful insights we derive from data through our analytical tools and platform capabilities. Schedule a demo today!
Request a Free DemoHow does predictive Data analytics work in the IT Industry?
These techniques are an assortment of business intelligence, online analytical processing (OLAP), and other advanced applications with a much broader focus. These services have expansive organizational benefits that help businesses drive revenue, improve operational efficiency, optimize customer experience, and develop effective marketing campaigns. Data analytics coupled with business intelligence and related advanced analytical services can work wonders in every industry, especially in the booming IT industry.
Predictive analytics is a powerful tool that leverages statistical models and machine learning algorithms to uncover patterns and relationships within data, enabling organizations to make informed decisions and predictions about future outcomes. The process of implementing predictive analytics involves several key steps:
Data Collection and Preparation
The foundation of predictive analytics lies in the availability of high-quality data. Data scientists gather relevant data from various sources, such as customer interactions, historical records, and real-time feeds. This data is then cleaned, transformed, and organized into a structured format suitable for analysis.
Feature Engineering
Feature engineering is the process of selecting and transforming relevant variables (features) from the raw data to create more informative and predictive inputs for the statistical models. Data scientists carefully choose and manipulate features to enhance the model’s ability to capture the underlying patterns and relationships in the data.
Model Selection and Training
Based on the problem at hand and the nature of the data, data scientists select appropriate statistical models or machine learning algorithms. These models are then trained on the prepared data, allowing them to learn from past patterns and relationships. The training process involves iteratively adjusting the model’s parameters to minimize the error between the predicted outcomes and the actual outcomes.
Model Evaluation and Validation
To ensure the accuracy and reliability of the predictive models, they are evaluated using various metrics and techniques. Data scientists split the data into training and testing sets, with the testing set being used to assess the model’s performance on unseen data. This process helps identify any potential overfitting or underfitting of the model.
Deployment and Monitoring
Once the predictive models have been developed and validated, they are deployed into production environments, where they can be used to generate predictions and insights. These insights are then integrated into decision-making processes, such as targeted marketing campaigns, fraud detection systems, or inventory optimization strategies. Continuous monitoring of the model’s performance is crucial to ensure that it remains accurate and relevant over time, as new data becomes available.
Predictive analytics has a wide range of applications across various industries, from healthcare to finance to retail. By leveraging the power of data and advanced statistical techniques, organizations can gain a competitive edge, make more informed decisions, and optimize their operations for better outcomes.
Experience the advantages firsthand by testing a customized complimentary pilot designed to address your specific requirements. Pilot studies are non-committal in nature.
Request a free trial nowTypes of Predictive Data Analytics
Predictive modeling analytics or Advanced analytics are made to evaluate past data, find trends, identify patterns, and utilize that knowledge to forecast future trends. Time series, clustering, and classification models are common predictive modeling analytics.
Classification Models
Classification models, part of supervised machine learning, are essential for predictive analytics in decision-making. They classify data based on past behavior, aiding in customer behavior prediction and risk management using predictive analytics. Used in fraud detection and credit risk, models like neural networks, decision trees, and logistic regression are key in AI in predictive data analytics.
Cluster Models
Cluster models, an unsupervised learning technique, group data by similar traits, enabling predictive data insights. Businesses, like e-commerce platforms, use clustering to target customer segments. Techniques like K-means and DBSCAN are often applied in predictive analytics case studies and are pivotal in industries like healthcare and retail, driving future trends in predictive analytics.
Time Series Models
Time series models track data over time, crucial for sales forecasting with predictive analytics and predictive analytics in healthcare. Models like ARIMA help predict trends and seasonal patterns. These models also play a role in predictive maintenance solutions, showing the importance of time-based predictions in shaping future trends in predictive analytics.
5 Benefits of Predictive Data Analytics
Predictive modeling offers a multitude of advantages for organizations seeking to leverage data-driven insights for strategic decision-making and operational optimization:
Enhanced Business Advantage
By utilizing predictive modeling, organizations can gain a competitive edge by anticipating future trends and outcomes based on historical patterns. This foresight enables better management of inventories, workforce allocation, marketing strategies, and overall operational efficiency.
Strengthened Security Measures
The integration of automation and predictive analytics enhances security protocols by identifying and responding to suspicious or abnormal user behavior patterns. This proactive approach allows organizations to preemptively address security threats and mitigate risks associated with data breaches or unauthorized access.
Risk Mitigation
Predictive analytics aids businesses in reducing risk exposure by providing valuable insights into potential vulnerabilities and opportunities for improvement. For instance, companies can assess credit risks more accurately, optimize insurance coverage, and make informed decisions to minimize financial uncertainties.
Operational Excellence
Efficient workflows are essential for maximizing profitability. Predictive modeling helps organizations streamline operations by predicting maintenance needs, optimizing resource allocation, and ensuring timely deliveries. This proactive approach minimizes downtime, reduces costs, and enhances overall operational efficiency.
Informed Decision-Making
Data-driven insights from predictive analytics empower organizations to make informed and strategic decisions. Whether expanding product lines, entering new markets, or pursuing growth opportunities, predictive modeling provides valuable foresight to assess risks, evaluate potential outcomes, and make decisions that align with business objectives.
By harnessing the power of predictive modeling, organizations can unlock a wealth of benefits that drive growth, enhance security, optimize operations, and enable informed decision-making in an increasingly competitive business landscape.
What are the 6 predictive big data analytics Trends in the IT Industry?
The ultimate goal of any business, irrespective of the industry it operates in, is to boost business performance and drive profitability. The IT industry possesses large amounts of information – historical and real-time – from a variety of sources that can be processed and analyzed with the help of analytical tools. With the help of predictive model building, the IT industry players can leverage this data to reduce costs, optimize processes, and enhance organizational operability. But what are the predictive maintenance solution trends that will revamp the IT industry? Read on.
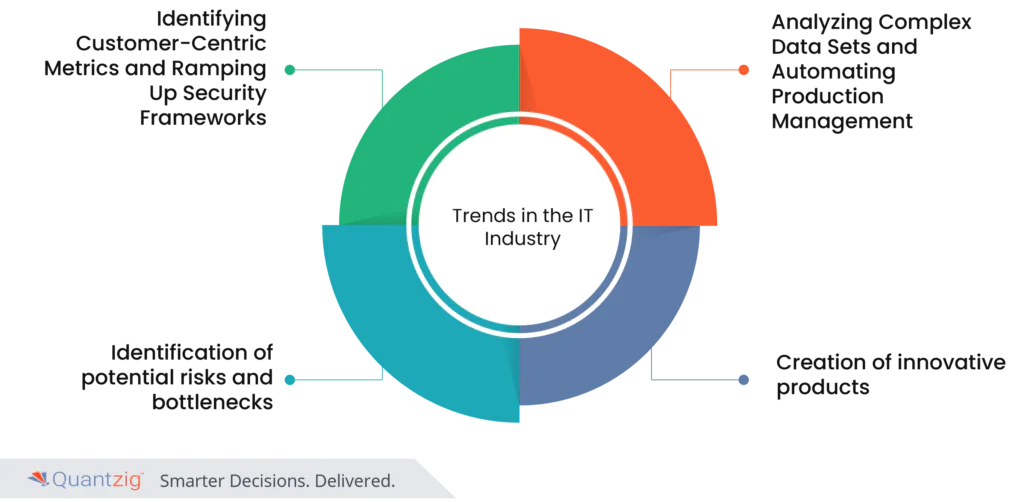
1. Identifying Customer-Centric Metrics:
- Enhanced Customer Experience:
The IT industry is increasingly leveraging this technology to identify customer-centric metrics, measuring and monitoring performance across various parameters in predictive model building. This enables businesses to track brand perception, gauge customer satisfaction, and tailor services for an enhanced customer experience.
2. Ramping Up Security Frameworks:
- Data Security Prioritization:
With the rise of technology, there’s a concerted effort in the IT industry to bolster security frameworks. This proactive approach aims to eliminate security issues, safeguard sensitive information, and fortify systems against vulnerabilities, ensuring time series data mining, integrity and user trust.
3. Analyzing Complex Data Sets:
- Seamless Business Processes:
IT vendors are increasingly relying on advanced software to analyze and interpret complex data sets. This trend simplifies business processes, ensuring operational efficiency, a seamless experience, and extracting valuable insights for informed decision-making.
4. Automating Production Management:
- Reducing Human Intervention:
Technology like Statistical modeling is enabling IT companies to automate production management, minimizing the need for extensive human intervention. By analyzing historical data and real-time information, businesses can automate physical changes to equipment, time series data mining, optimizing manufacturing processes through targeted commands to actuators and advanced robots.
5. Identification of potential risks and bottlenecks
- Optimal Risk Management:
Data analytics in IT plays a crucial role in identifying potential risks and bottlenecks. In the ever-changing landscape of technology, having robust risk management processes is essential. It assists in developing optimal risk management solutions and devising agile strategies to navigate uncertainties.
6. Creation of innovative products
- Customer-Centric Innovation:
This platform empowers IT companies to innovate products by collecting and analyzing extensive Data mining techniques on existing market offerings via Statistical modeling. Insights from customer feedback, product success metrics, and competitor inputs guide businesses in understanding customer needs, driving innovation, and staying ahead in the competitive landscape.
Get started with your complimentary trial today and delve into our platform without any obligations. Explore our wide range of customized, consumption-driven analytical solutions services built across the analytical maturity levels.
Start your free trial nowModern Techniques and Data Analytics
Predictive analytics for decision-making is transforming industries by leveraging AI in predictive data analytics to provide deep insights into customer behavior prediction. Companies use predictive analytics case studies to showcase real-world applications, including sales forecasting with predictive analytics, which helps businesses make data-driven decisions. In healthcare, predictive analytics plays a crucial role in enhancing patient outcomes by predicting potential health risks. Additionally, it aids in risk management using predictive analytics, enabling organizations to mitigate future uncertainties. As future trends in predictive analytics evolve, businesses can expect more refined predictive data insights to stay competitive and innovative.
Predicting trends in the IT industry using Modeling techniques requires a comprehensive understanding of various factors such as cryptocurrency trends, data science advancements, AI and ML technologies, business intelligence tools, edge computing, IoT, and Data mining techniques. By staying updated with these trends, businesses can harness the power of analytical services to drive growth, optimize operations, and gain a competitive advantage in the ever-evolving IT landscape. The implementation of data product containers, the utilization of AWS services for managing data domains, and the adoption of data lakes and enterprise data catalogs are key trends shaping the industry.