Table of Contents
Table of Contents
- Introduction to Demand Forecasting Challenges
- What is Retail Demand Forecasting?
- Why is Demand Forecasting Important?
- What are the Retail Demand Forecasting Challenges?
- Benefits of AI in Solving Retail Demand Forecasting Challenges
- Quantzig’s Demand Forecasting Solution
- Conclusion
Author: Associate Vice President, Analytics and Data Strategy, Quantzig.
Introduction to Demand Forecasting Challenges
In today’s data-driven retail landscape, businesses face increasing complexity in demand forecasting. Accurate forecasting is crucial for enhancing customer service and gaining a competitive edge. Effective demand forecasting models leverage historical consumer data to strategically plan for future trends and anticipate peak demand periods, enabling retailers to make data-driven decisions and drive business growth. However, developing robust forecasting models that address the complexities of the modern retail environment presents significant challenges. This article outlines the key demand forecasting challenges confronting the retail industry, as identified by industry experts.
Book a demo to experience the meaningful insights we derive from data through our analytical tools and platform capabilities. Schedule a demo today!
Request a Free DemoWhat is Retail Demand Forecasting?
Retail demand forecasting is the process of predicting future customer demand for products or services based on historical data, market trends, and other relevant factors. Accurate demand forecasting enables retailers to optimize inventory, improve customer satisfaction, and make better strategic and operational decisions.
Why is Demand Forecasting Important?
Demand forecasting is important for retailers for several key reasons:
1. Improved Inventory Management
Accurate demand forecasting allows retailers to optimize inventory levels, ensuring they have the right products available to meet customer demand without overstocking. This helps reduce costs associated with excess inventory and stockouts.
2. Enhanced Customer Satisfaction
By forecasting demand accurately, retailers can ensure product availability and avoid stockouts, leading to improved customer satisfaction and loyalty. Customers are more likely to return to a retailer that consistently has the products they want in stock.
3. Better Financial Planning
Reliable demand forecasts enable retailers to make more informed decisions about production, purchasing, staffing, and other financial aspects of the business. This leads to better resource allocation and improved profitability.
4. Competitive Advantage
Retailers who can effectively forecast demand and respond to market changes gain a competitive edge over those relying on guesswork or outdated methods. Accurate forecasting allows them to be more agile and responsive to customer needs.
5. Reduced Waste and Costs
Precise demand forecasting helps retailers avoid overstocking or understocking, minimizing waste, spoilage, and the costs associated with excess inventory or stockouts.
In summary, demand forecasting is crucial for retailers to optimize operations, enhance the customer experience, improve financial performance, and stay competitive in the rapidly evolving retail landscape. By leveraging data-driven forecasting techniques, retailers can make better-informed decisions and adapt more effectively to changing market conditions.
What are the Retail Demand Forecasting Challenges?
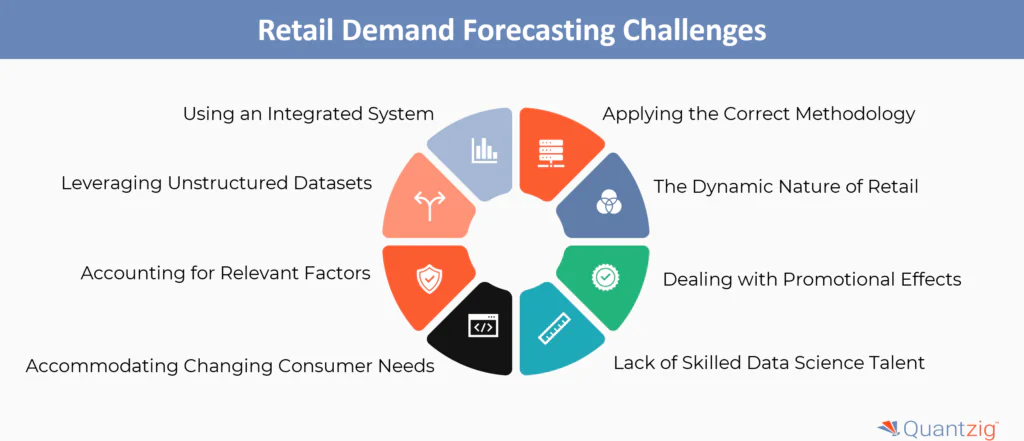
Challenge 1. Using an Integrated System
Retailers often have siloed systems for enterprise resource planning and customer relationship management, leading to duplication of information and difficulty gaining actionable insights from unstructured data. Lack of an integrated system makes it challenging for retailers to improve their business operations.
Challenge 2. Applying the Correct Methodology
Many retailers make strategic decisions based on company-wide goals rather than focusing on specific regions. This approach lacks visibility into growth expectations and fails to identify underperforming or overperforming regions. Having a proper demand management process is crucial for data-driven decision-making.
Challenge 3. Leveraging Unstructured Datasets
Retailers struggle to effectively utilize external data or customer profiles to gain detailed insights into customer demands and preferences. Building demand forecasting models can help retailers segment customers and analyze the demands of target customer segments.
Challenge 4. The Dynamic Nature of Retail
Factors influencing demand are constantly changing, including product mix, competition, geodemographics, promotions, seasonality, and vendor pricing. Basing forecasts on historical sales data alone yields inaccurate results.
Challenge 5. Identifying and Accounting for Relevant Factors
Determining all the variables influencing demand and their interplay is extremely challenging. As the number of variables increases, computing demand becomes exponentially more complicated. Traditional spreadsheet-based forecasting is time-consuming, expensive, and prone to errors.
Challenge 6. Dealing with Seasonality and Promotional Effects
Retail demand is highly seasonal, with significant fluctuations around holidays, events, and promotional periods. Accurately forecasting the impact of these factors on demand is critical, yet extremely complex. Retailers struggle to incorporate the nuanced effects of seasonality, holidays, and promotions into their forecasting models.
Challenge 7. Accommodating Rapidly Changing Consumer Preferences
Consumer preferences and buying behaviors are evolving at an unprecedented pace, driven by factors like social media, e-commerce, and emerging technologies. Retailers must quickly adapt their forecasting approaches to keep up with these dynamic shifts in consumer demand.
Challenge 8. Lack of Skilled Data Science Talent
Developing robust demand forecasting models requires specialized expertise in areas like statistics, machine learning, and data engineering. Many retailers lack the in-house talent and resources to build and maintain sophisticated forecasting systems, hindering their ability to leverage data-driven insights.
To address these challenges of demand forecasting, leading retailers are adopting AI-powered demand forecasting technologies that can process millions of data points and scale with the business. By leveraging machine learning and advanced analytics, retailers can improve forecast accuracy, optimize inventory, and enhance customer experience.
Also Read: Track Business Progress with Marketing Analytics Dashboard
Experience the advantages firsthand by testing a customized complimentary pilot designed to address your specific requirements. Pilot studies are non-committal in nature.
Request a Free PilotWhat are the Benefits of AI in Solving Retail Demand Forecasting Challenges?
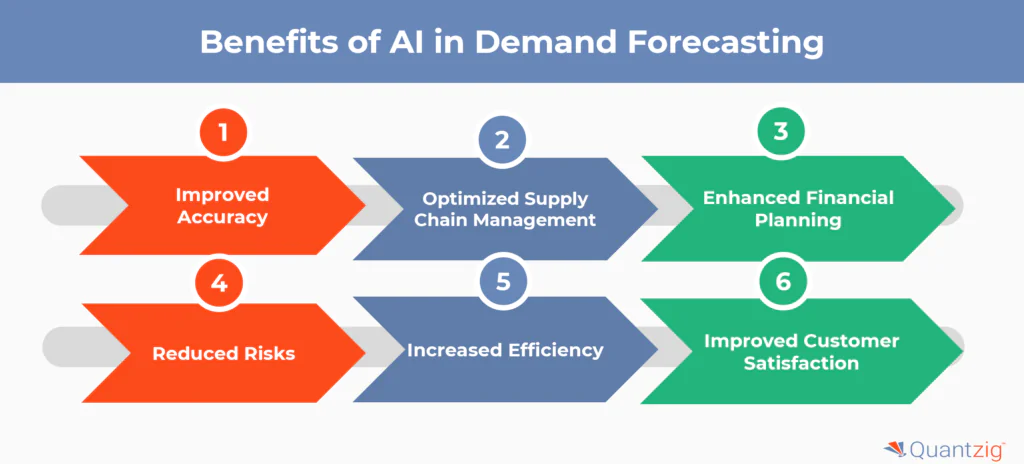
The key benefits of AI in solving challenges of demand forecasting include:
1. Improved Accuracy
- AI algorithms can analyze vast amounts of historical data to identify complex patterns and trends that traditional methods may miss
- Machine learning models are more robust to noise and find fundamental relationships in data, even when similarities between sales patterns are not obvious
2. Optimized Supply Chain Management
- AI factors in various variables like seasonality, promotions, market activities, and consumer behavior shifts to optimize supply chain dynamics
- Accurate forecasting reduces inefficiencies and improves overall supply chain management
3. Enhanced Financial Planning
- AI-driven forecasting provides precise insights into future demands, enabling effective resource allocation and minimizing financial risks
- Businesses can make informed decisions and stay ahead in the dynamic market landscape
4. Reduced Risks
- The accuracy of AI-based forecasting lowers risks associated with inventory management, production planning, and financial decision-making
- AI contributes to a more resilient and adaptable business strategy by providing reliable forecasts
5. Increased Efficiency
- AI can automate the process of collecting and analyzing data, freeing up employees to focus on other tasks
- This leads to increased productivity and efficiency in demand forecasting
6. Improved Customer Satisfaction
- Accurate forecasting ensures businesses have the right products available when customers want them
- This enhances customer satisfaction and loyalty
By leveraging the power of AI, businesses can generate more accurate and reliable demand forecasts, leading to better decision-making, optimized operations, and improved business outcomes in the face of increasing complexities in the retail environment.
How can Quantzig Help You with Demand Forecasting Solution?
Quantzig offers demand forecasting in retail solutions that help businesses create accurate demand capacity and plans. Our demand forecasting solutions help companies boost their forecast accuracy, improve service levels, manage portfolios, and maximize return on demand planning efforts.
Quantzig, with its expertise in offering demand planning and forecasting solutions, empowers businesses to discover hidden performance drivers, increase profitability, improve collaboration, validate their business strategies, gain a 360-degree view of demand, and optimize return on investment (ROI) on operational spends.
Also Read: Maximizing Marketing Budgets with Campaign ROI Analysis
Get started with your complimentary trial today and delve into our platform without any obligations. Explore our wide range of customized, consumption driven analytical solutions services built across the analytical maturity levels.
Start your Free Trial TodayConclusion
In the retail industry, demand forecasting presents several challenges, including managing inventory levels, optimizing pricing, and enhancing customer experience. Leveraging analytics and AI-powered technologies is essential to tackle these issues effectively. Advanced analytics helps in reducing inventory cost and improving inventory turnover, while minimizing manual labor and resource costs. Retailers must adopt robust inventory management and distribution strategies to stay competitive. Accurate forecasting models are crucial for improving GMROI (Gross Margin Return on Investment) and ensuring a resilient supply chain. Addressing these challenges enables better decision-making and operational efficiency in the dynamic retail environment.