Table of Contents
Table of Contents
- Introduction
- What is Clinical Data Management (CDM)?
- Why is CDM Important?
- Stages of Clinical Data Management
- CDM Roles and Responsibilities
- CDM Tools
- CDM Best Practices
- Top Challenges in Clinical Data Management System
- Strategies to Overcome the Clinical Data Management System Challenges
- Conclusion
Author: Associate Vice President, Analytics and Data Strategy, Quantzig.
Introduction to Clinical Data Management
Clinical data management is a critical function responsible for the systematic collection, organization, and administration of research data from clinical trials and studies. As the volume and complexity of clinical data continue to grow, clinical data managers face a range of challenges that can impact data quality, integrity, and timeliness. From managing vast data sets to ensuring data security and regulatory compliance, clinical data management teams must navigate a complex landscape of technological, operational, and organizational obstacles.
This article explores the top challenges in clinical data management and discusses strategic approaches that organizations can adopt to overcome these obstacles and optimize their data management practices.
Book a demo to experience the meaningful insights we derive from data through our analytical tools and platform capabilities. Schedule a demo today!
Request a Free DemoWhat is Clinical Data Management (CDM)?
Clinical data management (CDM) is the process of collecting, organizing, validating, and analyzing data generated during clinical trials and healthcare research activities. The primary goal of CDM is to provide high-quality, reliable, and statistically sound data by minimizing errors and missing data and gathering the maximum amount of data for analysis. CDM involves various activities such as case report form (CRF) design, database development, data entry, data cleaning, medical coding, and database locking.
Why is CDM Important?
CDM is critical for the success of clinical trials and healthcare research as it ensures the integrity, accuracy, and completeness of the data collected. High-quality data obtained through rigorous CDM processes is essential for regulatory submissions, data analysis, and drawing valid conclusions about the safety and efficacy of new medical products. Effective CDM also helps accelerate the drug development process by facilitating faster data processing and decision-making.
Stages of Clinical Data Management
Stage | Description |
---|---|
1. Study Planning | Define the study protocol, identify data requirements, and design the case report forms (CRFs) |
2. Data Collection | Collect data from various sources, including electronic data capture (EDC) systems, paper CRFs, and medical records |
3. Data Entry | Enter data into the database, ensuring accuracy and completeness |
4. Data Cleaning | Identify and correct errors, inconsistencies, and missing data |
5. Data Validation | Verify data against predefined rules and standards to ensure accuracy and completeness |
6. Data Coding | Assign standardized codes to data elements, such as medical conditions and adverse events |
7. Data Analysis | Perform statistical analysis to extract insights and draw conclusions from the data |
8. Data Locking | Finalize the database and prevent further changes to ensure data integrity |
9. Data Reporting | Generate reports and summaries of the data for stakeholders and regulatory submissions |
10. Data Archiving | Store the data securely and maintain access for future reference and compliance purposes |
These stages ensure that the data collected during clinical trials is accurate, complete, and reliable, facilitating informed decision-making and regulatory compliance.
CDM Roles and Responsibilities
The key roles and responsibilities in clinical data management (CDM) include:
Role | Responsibilities |
---|---|
Data Manager | – Supervises the entire CDM process and coordinates data management activities – Ensures the accuracy and integrity of the study data – Manages data validation and quality control procedures – Takes responsibility for database locks |
Database Programmer/Designer | – Performs the case report form (CRF) annotation – Creates the study database and designs the data entry screens – Programs the edit checks for data validation – Validates the edit checks with a dummy dataset |
Medical Coder | – Codes variations such as adverse events and medical histories |
Clinical Data Coordinator | – Designs the CRF and creates the CRF instructions – Creates the discrepancy protocols |
Quality Control Associate | – Checks the accuracy of data entry and performs data audits |
Data Entry Associate | – Tracks receipt of the CRF pages and enters the data into the database |
Investigators and Clinicians | – Collect the data during the study using CRFs |
Site and Data Personnel | – Enter the data into the database following receipt of the CRF pages |
Biostatisticians | – Conduct statistical analysis of the study data |
Medical Writers | – Prepare the study reports |
This table clearly outlines the key roles involved in clinical data management and their respective responsibilities, providing a concise overview of the CDM team and their functions.
CDM Tools
Some common tools used in clinical data management include:
- Clinical Data Management Systems (CDMS): Also known as electronic data capture (EDC) systems, these are specialized software applications designed to handle large amounts of clinical trial data and ensure compliance with regulations.
- Oracle Clinical: A comprehensive CDMS solution for managing clinical trials data.
- Rave: A cloud-based EDC system by Medidata for collecting, managing, and reporting clinical trial data.
- eClinical Suite: An integrated suite of clinical trial management tools including EDC, CTMS, and safety systems.
- Clintrial: A CDMS platform for managing clinical data, safety, and regulatory submissions.
- Macro: A flexible CDMS solution that can be customized for specific clinical trial requirements.
Some key best practices in clinical data management include:
1. Data Management Plan (DMP) Design
- Develop a comprehensive DMP that outlines data collection, storage, validation, and management procedures.
- Ensure the DMP is aligned with relevant regulations and industry standards like CDASH and SDTMIG.
- Keep the DMP up-to-date as the study progresses and changes occur.
2. Data Quality and Integrity
- Implement robust data validation checks and quality control measures.
- Maintain an audit trail to track all data changes and ensure traceability.
- Adhere to 21 CFR Part 11 compliance for electronic records and signatures.
3. Standardization and Harmonization
- Use standardized data formats, terminologies, and coding systems across studies.
- Leverage industry standards like CDISC to facilitate data sharing and regulatory submissions.
4. Collaboration and Communication
- Foster close collaboration between the CDM team, investigators, sponsors, and other stakeholders.
- Establish clear communication channels and reporting mechanisms.
5. Continuous Improvement
- Regularly review and optimize CDM processes based on lessons learned and industry best practices.
- Invest in training and professional development for the CDM team.
By following these best practices, organizations can ensure high-quality, compliant, and efficient clinical data management to support successful clinical trials and regulatory submissions.
What are the Top Challenges in Clinical Data Management System?
Today, all modern industries and companies are embracing the power of digital technologies. The healthcare industry is also following the trend by using patient data management systems, which keeps track of patient information and medical history thereby replacing handwritten medical files. Since patient data is sensitive, medical companies and professionals should adopt new strategies to manage such healthcare data securely. Clinical data management simplifies a lot of operational tasks thereby increases the efficiency of healthcare providers. However, it can be challenging to manage such large and complex sets of healthcare data. Clinical data management is further complicated by various government regulations and compliance issues.
Challenge 1. HIPAA Compliance
- The Health Insurance Portability and Accountability Act (HIPAA) requires security measures for EHRs to be shared among medical practitioners and be made accessible to patients.
- The majority of healthcare providers have to balance between operating a closed-network system and implementing shared data access and security protocols.
- Also, after providing a platform to share data between practitioners, a data breach could cost healthcare companies significantly higher than in other industries.
Challenge 2. Sharing Patient Data
- Sharing patient data is still a grey area for players in the medical industry. Some regulations restrict sharing of data with a medical representative and other medical professionals.
- To tackle such issues, the medical industry is working on strategies like EHRs and cloud computing to facilitate sharing of clinical data.
- Additionally, integrating and standardizing the sharing platform for every medical professional to grant access to healthcare data is also an ongoing challenge.
Challenge 3. Mobile Computing
- The demanding nature of today’s digital technology has placed great importance on mobile computing. Professionals want access to data at the comfort within their fingertips.
- However, shifting the platform to mobile devices seems quite a challenge in the field of clinical data management. It’s a lot easier to upload medical entries to a tablet directly then scribbling on medical charts for transcription later.
- The problem arises when providing secure wireless access throughout the care facility to medical professionals. It requires developing completely new security and compliance protocols for mobile devices.
Challenge 4. Operational Analytics
- The clinical data management systems and EHRs have helped improve the quality of care that is provided to the patients. Alongside patients, healthcare professionals, and the workforce have also benefitted with a substantial increase in operational efficiency.
- For instance, healthcare workforce management is largely measured using patient care and healthcare data, which isn’t an ideal metric for performance measurement.
- As a result, it has urged HIS managers to look for new strategies to mine healthcare data in order to perform productivity and profitability analytics to identify true measures of performance and identify improvement areas.
Challenge 5. Data Volume and Complexity
- The sheer volume and complexity of clinical data pose significant challenges. With more patient data becoming available, CDM systems struggle to keep up. This can lead to delays in data processing, analysis, and reporting.
- Additionally, the complexity of data formats, terminologies, and standards can make it difficult to ensure data quality and integrity. Effective data management requires robust systems and processes to handle the volume and complexity of data.
Challenge 6: Data Quality and Integrity
- Ensuring the accuracy, completeness, and consistency of clinical data is critical but difficult. Data entry errors, lack of standardized formats, and inconsistent terminology can compromise data quality.
- Moreover, data integrity issues can arise from data migration, integration, and transformation. To address these challenges, CDM professionals must implement robust data validation, verification, and quality control processes to ensure data accuracy and integrity.
Challenge 7: Data Security and Privacy
- Protecting the confidentiality of sensitive patient information is paramount, especially with the rise in digital healthcare records and cyber threats like data breaches and ransomware attacks.
- CDM systems must ensure secure data storage, transmission, and access controls to prevent unauthorized access.
- Additionally, data encryption, access controls, and audit trails are essential to maintain data security and privacy.
Challenge 8. Interoperability
- Integrating diverse data sources, formats, and systems to enable seamless data sharing and communication across the clinical ecosystem is a significant challenge.
- CDM systems must ensure interoperability with various systems, including electronic health records (EHRs), clinical trial management systems (CTMS), and other data management systems. This requires standardization, data mapping, and integration to facilitate data exchange and analysis.
Also Read: Pharma Customer Segmentation Helped a Client Improve Customer Satisfaction by 10%
Experience the advantages firsthand by testing a customized complimentary pilot designed to address your specific requirements. Pilot studies are non-committal in nature.
Request a Free PilotWhat are the Strategies to Overcome the Clinical Data Management System Challenges?
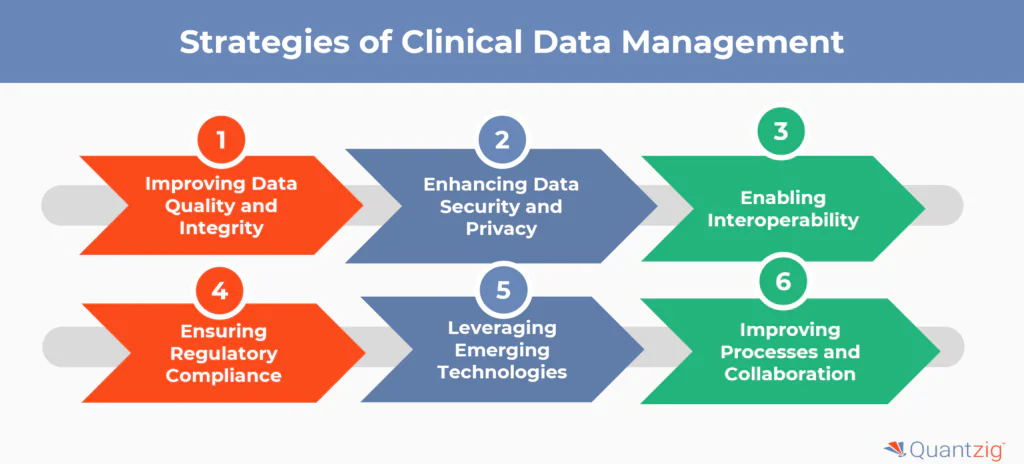
Some key strategies to overcome the challenges in clinical data management system include:
1. Improving Data Quality and Integrity
- Implement robust data validation checks and automated validation protocols to ensure data accuracy
- Conduct regular audits and employ statistical methods for anomaly detection to maintain data integrity
- Standardize data formats and terminology to reduce complexities
- Provide comprehensive training to data entry personnel on proper data entry techniques
2. Enhancing Data Security and Privacy
- Employ advanced encrypted technologies and stringent access controls to protect sensitive patient data
- Regularly conduct statistical risk assessments and audits to identify vulnerabilities and address potential security threats
- Ensure compliance with data privacy regulations like HIPAA and GDPR
- Implement robust backup and disaster recovery plans to safeguard against data loss
3. Enabling Interoperability
- Adopt common data standards and terminologies like CDISC to facilitate data exchange
- Develop APIs and middleware to integrate diverse systems and data sources
- Participate in industry initiatives for interoperability like the CDISC Interchange
4. Ensuring Regulatory Compliance
- Stay informed about evolving regulations through industry associations and regulatory bodies
- Conduct statistical analysis to assess the impact of regulatory changes and ensure timely adjustments
- Maintain thorough documentation and audit trails for inspections and audits
- Implement quality management systems to proactively identify and mitigate compliance risks
5. Leveraging Emerging Technologies
- Utilize AI and machine learning for data cleaning, anomaly detection, and predictive modeling
- Adopt cloud computing for scalable data storage, processing, and collaboration
- Explore blockchain technology for secure, decentralized data sharing and provenance
- Implement natural language processing (NLP) to extract insights from unstructured data like clinical notes
6. Improving Processes and Collaboration
- Adopt agile methodologies for faster, iterative development and deployment of CDM systems
- Involve stakeholders like investigators, sponsors, and CROs in process re-engineering
- Streamline workflows and eliminate redundancies to improve efficiency
- Foster cross-functional collaboration and communication to align on goals and priorities
By strategically addressing these challenges through a combination of process improvements, technology adoption, and collaborative approaches, organizations can enhance the quality, efficiency, and impact of their clinical data management practices.
Also Read: Customer Loyalty Analysis for a Leading Pharma Player in Europe
Get started with your complimentary trial today and delve into our platform without any obligations. Explore our wide range of customized, consumption driven analytical solutions services built across the analytical maturity levels.
Start your Free Trial TodayConclusion
Top challenges in Clinical Data Management (CDM) include ensuring data integrity across diverse clinical data sources, adhering to Good Clinical Practice (GCP), and maintaining compliance with The Clinical Data Acquisition Standards Harmonization (CDASH). Clinical trial management systems (CTMS) must effectively handle complex data from drug and device studies and healthcare research in the biotech, pharmaceutical sector, and life sciences field. Developing a robust Clinical DMP is critical, as is the collaboration between data managers and biostatisticians to ensure accurate data analysis. These challenges are compounded by the need for seamless integration of new technologies in healthcare and clinical research environments.