In the current era of swiftly advancing technology, the integration of artificial intelligence (AI) and machine learning (ML) has become more than just a competitive advantage—it is a necessity for businesses aiming to stay relevant and thrive in the digital age. As AI applications continue to proliferate across industries, the effective management and deployment of machine learning models has become paramount. MLOps, short for Machine Learning Operations, emerges as a transformative force, offering organizations the necessary tools and practices to leverage the ultimate advantage of AI.
MLOps represents the fusion of machine learning, software engineering, and operations. It aims to streamline the development, deployment, and maintenance of ML models. It acts as the bridge between data science and IT operations, offering a systematic approach to managing the entire machine learning (ML) lifecycle.
Table of Contents
Importance of Tech Transformation – Harnessing MLOps for the Ultimate AI Advantage
- Institutionalizing AI thinking encourages all employees to consider the potential benefits of AI and ML across various departments. It promotes a proactive approach to harnessing AI across the organization.
- Effective collaboration between data scientists and engineers is critical. MLOps bridges this gap by promoting shared responsibilities and accountability, streamlining workflows, and reducing conflicts.
- MLOps makes AI more accessible to business stakeholders by simplifying model deployment and monitoring, enabling informed decision-making, and improving business outcomes.
- Streamlined processes and collaboration accelerate the adoption of AI. MLOps automates tasks, expedites AI deployment, and enhances competitiveness.
- Prudent governance is crucial for the ethical and responsible development of AI. MLOps facilitates governance through version control, model monitoring, and compliance checks. It ensures transparency and accountability while mitigating risks associated with biased or unreliable AI.
The challenges of implementing Tech Transformation
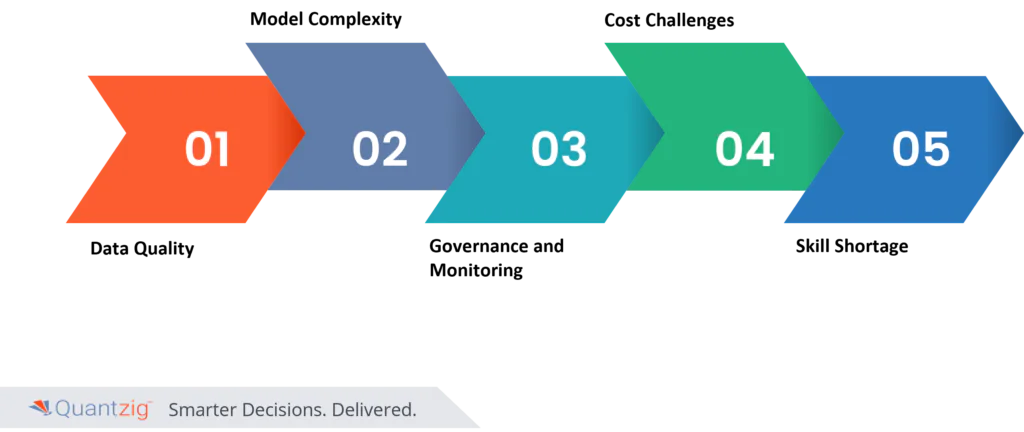
- Data Quality: Ensuring high-quality data is paramount, requiring substantial efforts in data cleansing, augmentation, and governance to prevent erroneous insights. Plagiarism in data collection can lead to legal and reputational consequences.
- Model Complexity: Modern AI models require constant adaptation, but managing their complexity can be daunting. Robust version control, model monitoring, and retraining pipelines are essential. Plagiarism in model development poses a risk of ethical dilemmas and intellectual property (IP) issues.
- Governance and Monitoring: Maintaining transparency and accountability throughout the AI lifecycle are vital. This includes tracking changes in data distribution and model drift. Plagiarism in monitoring processes undermines ethical standards.
- Cost Challenges: Maintenance costs for MLOps can be prohibitive, especially for AI adopters in the early stages. Budgeting and ethical practices are crucial because unethical shortcuts can result in unforeseen expenses and legal consequences.
- Skill Shortage: Finding skilled MLOps, data engineering, and ML professionals is challenging. Plagiarism or inadequate hiring practices can damage an organization’s credibility.
Key Benefits:
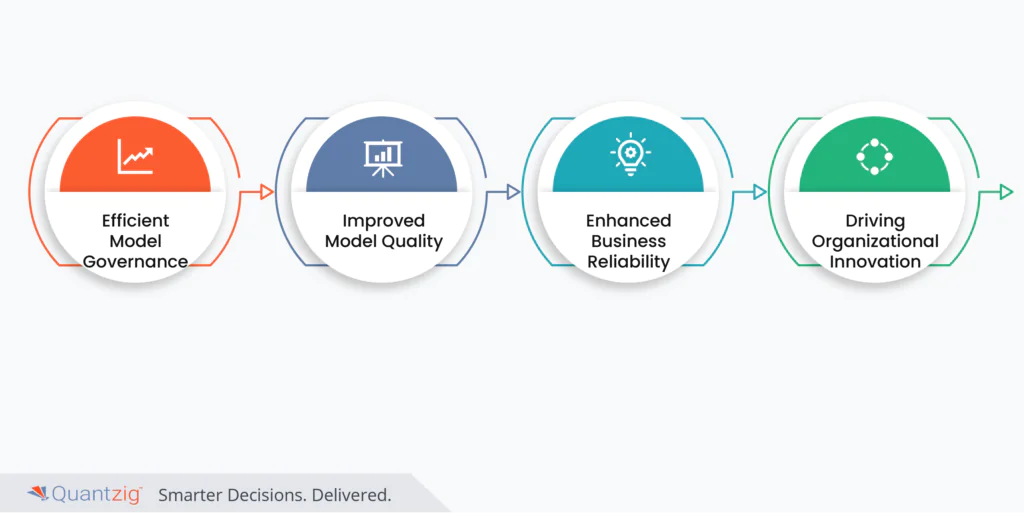
- Implementing MLOps in tech transformation offers several key advantages. Firstly, it streamlines model governance, reducing costs and time while ensuring high-quality models through robust testing, deployment, and ongoing maintenance.
- MLOps enhances the reliability of AI and ML solutions for businesses. Its enterprise-ready approach instils lconfidence in these technologies, facilitating innovation and transformation across various organizational functions.
- By fostering a culture of quality assurance and continuous improvement, MLOps elevates the overall model quality. This approach minimizes risks associated with model inaccuracies and errors when deployed in real-world scenarios.
- MLOps drives organizational innovation. It seamlessly integrates AI and ML into existing processes, empowering companies to explore new applications, optimize operations, and develop innovative products or services.
Success Story:
“Mastering MLOps: Elevating AI Transformation for Competitive Excellence”
Client Details: A large financial institution with a complex legacy IT infrastructure located in the Europe.
Challenges Faced by the Client:
The client was facing the issue of data fragmentation and incompatibility among its systems. The challenge lied in integrating these disparate data sources and systems to create a unified data ecosystem efficiently. The current data architecture lacked compatibility, necessitating manual efforts for data extraction, transformation, and loading (ETL), resulting in operational inefficiencies, increased error risks, and delayed decision-making processes.
Moreover, the institution was grappling with stringent regulatory requirements, making data accuracy and compliance a top priority. The absence of an integrated data solution posed a significant risk of non-compliance, potentially leading to severe legal and financial consequences.
In addition to these operational hurdles, the institution recognized the imperative of leveraging data for competitive advantage. The inability to efficiently access and analyze data restricted their ability to identify opportunities, mitigate risks, and offer personalized services to customers.
Overall, the challenge revolved around the pressing need for a streamlined data integration and management solution to unlock the institution’s data potential, ensure compliance, and drive competitive success in the highly regulated financial sector.
Solutions:
The comprehensive solution offered by our company addresses critical aspects of modern data-driven operations. It begins with an efficient data collection and streamlining process, automating data processing to create high-availability products and tools. This not only saves time but also ensures that your data is easily accessible and dependable.
Moreover, our solution emphasizes robust feature engineering, which involves creating a systematic data catalogue, meticulous labelling, and implementing workflows for feature engineering. This results in a unified data library that caters to your current and future data needs, promoting scalability and adaptability.
To stay ahead of evolving business landscapes, our solution includes continuous model enhancements. This means that your AI models will stay relevant and resilient in response to evolving market dynamics, processes, and live data feeds.
When it comes to deployment and governance, we prioritize portability and reproducibility. Our containerized deployments facilitate seamless integration into your current infrastructure, while our audit trail mechanisms ensure that model results can be replicated at any given time. This plug-and-play setup guarantees a seamless and reliable AI solution for your organization, aligning with your technological transformation objectives.
Why opt our Offering?
Efficient Process Ownership: Our solutions ensure a smooth transition as we take over control of your processes within just 100 days. This seamless onboarding is facilitated by our team of experienced Subject Matter Experts (SMEs) and purpose-built solution accelerators, designed to expedite the modernization of your data. With our approach, you can expect a seamless and efficient transformation of your data operations, minimizing any disruptions.
Proven Solutions: Our offerings have undergone rigorous testing and have demonstrated success across diverse industries and markets. We have successfully navigated various data and technology landscapes, validating the reliability and adaptability of our solutions. By choosing our offering, you gain the confidence of a well-tested solution tailored to your unique needs. This reduces implementation risks and expedites your journey toward data-driven excellence.
Conclusion
In today’s fast-paced technological landscape, the integration of AI and MLOps is imperative for maintaining business relevance. MLOps serves as a catalyst for efficient AI model management, acting as a vital bridge between data science and IT operations. The essence of AI lies in instilling AI thinking across all areas of an organization, promoting collaboration, and simplifying AI for business stakeholders. While obstacles such as data quality, model intricacies, and cost persist, the rewards are substantial. These rewards include streamlined model governance and enhanced quality. Our solutions, validated across diverse industries, ensure a seamless transition, address these challenges, and expedite the journey towards data-driven excellence. Thus, the integration of AI is not just advantageous; it is imperative in today’s digital landscape.
Related Articles
- Crafting the Future of Tech Trends with AI, MLOps, and Process Engineering
- 3 Amazing Retail Analytics Trends You May Not Have Known
- 3 Best Practices Businesses for Managing a Successful Retail Analytics Campaign
- 3 Ways to Improve Retail Price Optimization Strategy
- 30% Reduction in Employee Turnover Rates Achieved by Retail Chain Through the Implementation of Turnover Prediction Models
- AI Art Breeder: Revolutionizing Art Perception Through Artificial Intelligence