Written by: Medha Banerjee
Table of Contents
Key Takeaways:
- Data governance framework implies data quality, security, and consistency across an organization, aligning with business goals.
- A comprehensive structure for data policies, roles, and processes to manage data, ensuring compliance, security, quality, and alignment with business objectives.
- This framework is essential for data privacy by ensuring accuracy, completeness, security, and legal compliance.
- It helps to organizes, secures, manages, and presents data using methods and technologies, focusing on incremental implementation and technological advancements.
Data governance strategy and framework: What to expect
Data governance is an important component of modern business operations, ensuring that data is managed effectively and efficiently across an organization. A data governance framework provides a structured approach to data management, outlining the policies, procedures, and standards that govern the use and protection of data assets. In this guide, we will delve into the depth of data governance frameworks, exploring their advantages, pillars, examples, and best practices for implementation.
What is a Data Governance Framework?
A data governance framework is a cumulative set of guidelines and processes that define how an organization manages its data assets. It encompasses effective data governance strategy that defines the roles, responsibilities, policies, and procedures necessary for ensuring data quality, security, and compliance. The framework serves as a blueprint for data management, ensuring that data is used consistently and effectively throughout the organization.
Book a demo to experience the meaningful insights we derive from data through our analytical tools and platform capabilities. Schedule a demo today!
Request a Free DemoAdvantages of a Data Governance Framework
Implementing a well-executed and thought-out data governance framework offers numerous advantages to businesses of all sizes and industries. Some of the key benefits include:
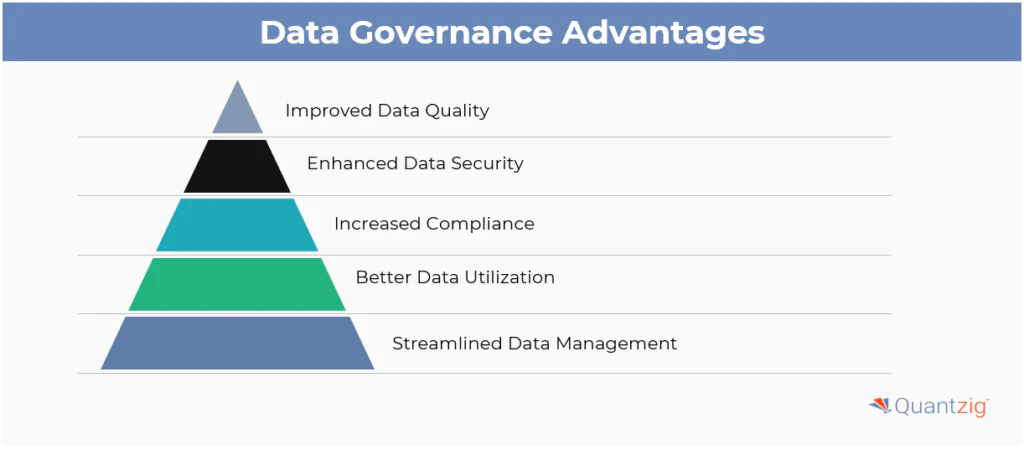
- Improved Data Quality: A well-designed data governance framework ensures that data is accurate, complete, and consistent, enabling better decision-making and reducing the risk of errors.
- Enhanced Data Security: The framework outlines clear policies and procedures for data access, storage, and protection, minimizing the risk of data breaches and unauthorized access.
- Increased Compliance: By aligning data management practices with relevant regulations and industry standards, a data governance framework helps organizations avoid costly penalties and legal issues.
- Better Data Utilization: With a clear understanding of data ownership, roles, and responsibilities, organizations can leverage their data assets more effectively, driving innovation and competitive advantage.
- Streamlined Data Management: A data governance framework provides a centralized approach to data management, reducing duplication of effort and ensuring that data is managed consistently across the organization.
Pillars of a Data Governance Framework
A comprehensive data governance framework typically comprises several key pillars, each of which plays a crucial role in ensuring effective data management. These pillars include:
- Data Policies and Standards: This pillar defines the policies, procedures, and standards that govern data management within the organization, ensuring consistency and compliance.
- Data Roles and Responsibilities: Clearly defined roles and responsibilities are essential for effective data governance, ensuring that everyone understands their role in managing data assets.
- Data Quality Management: This pillar focuses on ensuring that data is accurate, complete, and consistent, with processes in place for data validation, cleansing, and enrichment.
- Data Security and Privacy: Data security and privacy are critical components of a data governance framework, with policies and controls in place to protect sensitive data and ensure compliance with relevant regulations.
- Data Lifecycle Management: This pillar addresses the management of data throughout its lifecycle, from creation to disposal, ensuring that data is retained and disposed of in accordance with organizational policies and legal requirements.
- Data Stewardship: Data stewardship involves the active management and oversight of data assets, ensuring that data is used effectively and efficiently in support of organizational goals.
Examples of Data Governance Frameworks
There are several well-known data governance frameworks that organizations can use as a starting point for developing their own data governance strategies. Some of the most widely recognized examples include:
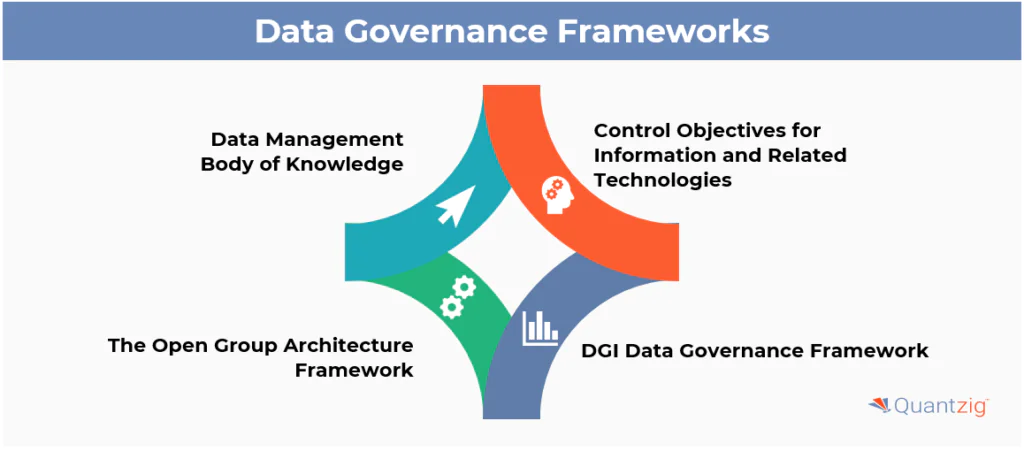
- DAMA DMBOK (Data Management Body of Knowledge): This framework, developed by the Data Management Association (DAMA), provides a comprehensive guide to data management best practices, covering areas such as data architecture, data modeling, and data security.
- COBIT (Control Objectives for Information and Related Technologies): COBIT is a framework developed by ISACA (Information Systems Audit and Control Association) that provides guidance on IT governance and control, including data governance.
- TOGAF (The Open Group Architecture Framework): TOGAF is an enterprise architecture framework that includes a data architecture component, providing guidance on data management and governance.
- DGI Data Governance Framework: Developed by the Data Governance Institute, this framework provides a structured approach to data governance, with a focus on data stewardship and data quality management.
By leveraging these models and examples, organizations can develop data governance frameworks that are tailored to their specific needs and priorities, while also drawing on best practices and industry standards.
5 Types of Data Governance Models
As we explore specific examples of data governance frameworks, it is essential to understand the various models that helps in guiding data governance decisions within an organization. These models determine how data governance decisions flow through the organization, influencing the overall effectiveness of the framework.
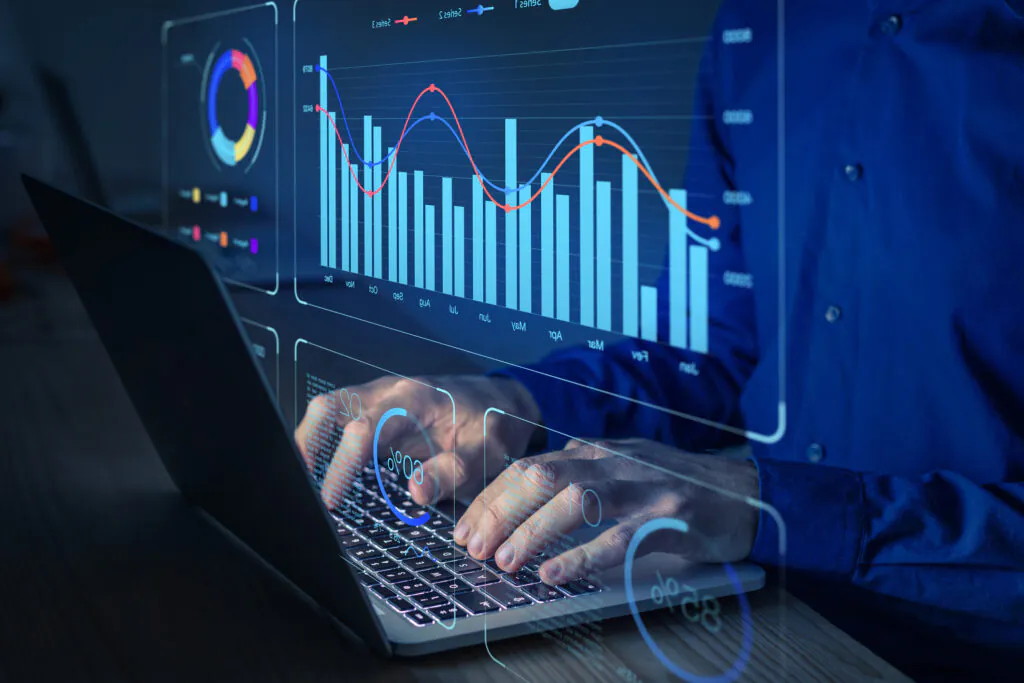
1. Top-Down Model:
Company leadership implements data governance policies that are then cascaded down to individual business units and shared with the rest of the company. This model emphasizes centralized control and standardization.
2. Bottom-Up Model:
Employees at lower levels implement data governance practices, such as standardizing naming conventions, which spread to higher levels of the organization. This model emphasizes grassroots innovation and adaptability.
3. Center-Out Model:
The team or individual responsible for data governance sets data standards that the entire organization follows. This model emphasizes centralized authority and consistency.
4. Silo-In Model:
Various departments come together to align on data governance while keeping in mind the needs of each group. This model emphasizes collaboration and adaptability.
5. Hybrid Model:
Data governance decisions involve different levels of the organization. For example, a company uses a center-out model to suggest a course of action but employs a top-down model to make the final decision. This model emphasizes flexibility and adaptability.
Understanding the Models
Each model has its strengths and weaknesses, and the choice of model depends on the organization’s specific needs and culture. By understanding these models, organizations can select the best approach for their data governance framework and ensure effective data management.
A Bottom-Up Approach to Data Governance
While a top-down approach to data governance, driven by executive leadership, can be effective, a bottom-up approach that involves stakeholders from across the organization can also bring in significant benefits. By engaging data users and subject matter experts in the development and implementation of the data governance framework, organizations can make sure that the framework is customized to the specific needs and challenges of the business.
A bottom-up approach to data governance typically involves the following steps:
- Identify Data Stewards: Identify data stewards within each business unit or functional area who are responsible for managing and overseeing data assets.
- Establish Data Governance Working Groups: Create working groups composed of data stewards and subject matter experts who are responsible for developing data policies, standards, and processes.
- Pilot Data Governance Initiatives: Pilot data governance initiatives within specific business units or functional areas to test and refine the data governance framework.
- Scale Data Governance: Once the data governance framework has been tested and refined, scale it across the organization, leveraging the expertise and buy-in of the data stewards and working groups.
By taking a bottom-up approach to data governance, organizations can make sure that the data governance framework is aligned with the needs and priorities of the business, while also developing a culture of data stewardship and ownership.
How to Create an Enterprise Data Governance Framework
Creating an effective enterprise data governance framework requires a structured approach that involves stakeholders from across the organization. Here are the key steps to follow:
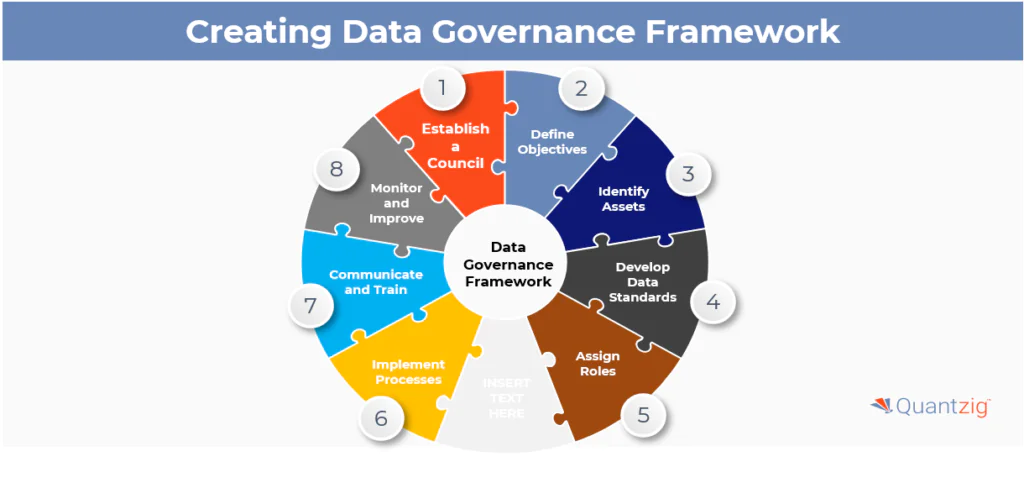
- Establish a Data Governance Council: Assemble a cross-functional team of stakeholders, including representatives from IT, business units, and data management, to oversee the development and implementation of the data governance framework.
- Define Data Governance Objectives: Clearly define the objectives of the data governance framework, aligning them with organizational goals and priorities.
- Identify Data Assets and Stakeholders: Conduct an inventory of the organization’s data assets and identify the key stakeholders responsible for managing and using those assets.
- Develop Data Policies and Standards: Create policies and standards that define how data will be managed, including data quality, security, and privacy requirements.
- Assign Data Roles and Responsibilities: Assign clear roles and responsibilities for data management, including data owners, data stewards, and data custodians.
- Implement Data Governance Processes: Establish processes for data management, including data acquisition, storage, and distribution, as well as processes for data quality management and data security.
- Communicate and Train: Domain data leadership should communicate the data governance framework to all stakeholders and provide training on data management best practices.
- Monitor and Continuously Improve: Regularly monitor the effectiveness of the data governance framework and make adjustments as needed to ensure that it remains relevant and effective.
Experience the advantages firsthand by testing a customized complimentary pilot designed to address your specific requirements. Pilot studies are non-committal in nature.
Request a free pilotQuantzig: Effortless Data Governance for the Modern Data Stack
Quantzig, a leading provider of Data Quality & Governance Solutions, offers a comprehensive suite of tools and services designed to help organizations implement and maintain effective data governance frameworks. Quantzig’s solutions are customized to the needs of modern businesses, leveraging cutting-edge technologies and best practices to streamline data governance processes and make sure that data assets are managed effectively and efficiently.
Quantzig’s Data Quality & Governance Solutions include:
Data Governance Consulting:
Quantzig’s team of data governance experts work closely with their clients to develop and implement customized data governance frameworks that align with organizational goals and priorities.
Data Governance Software:
Quantzig offers a range of data governance software solutions, which includes data cataloging, data lineage, and data quality management tools, to help organizations manage and monitor their data assets.
Data Governance Training:
Quantzig provides comprehensive training and education programs to help organizations build the skills and knowledge that are necessary for effective data governance, including data stewardship, data quality management, and data security.
By partnering with Quantzig, organizations can leverage the power of data governance to drive innovation, improve decision-making, and achieve their business objectives.
How Quantzig helped its client include effective data governance strategy for a better ROI?
Quantzig offers a range of innovative solutions designed to make the best of data governance and management. Their self-healing data governance systems helps in automating data validation, cleansing, and reconciliation processes via the integration of machine learning algorithms and advanced analytics. This system proactively detects and rectifies data issues, ensuring continuous data accuracy and reliability. With real-time data monitoring and automated alerts, clients can gain in-depth data insights, facilitating faster issue resolution and enhancing data-driven decision-making. These transformative solutions have brought in impacts within enhanced productivity, streamlined data management, and a data-centric culture, positioning clients for sustained growth and success.
Additionally, Quantzig’s ML-based data quality management solutions makes use of machine learning algorithms in order to automatically identify and rectify data anomalies, significantly improving data accuracy and reliability. Real-time data monitoring and predictive analytics capabilities allow clients to quickly detect and correct data quality deviations, reducing manual efforts in data validation and cleansing. This approach has streamlined processes, improved data integrity, and fortified decision-making, driving business efficiency and competitive advantage. Visual workflow management apps have further revolutionized client operations by providing user-friendly interfaces for visualizing and optimizing workflows, facilitating seamless collaboration, and boosting productivity. The impact of these solutions is evident, with clients experiencing an 80% reduction in time to resolve data issues, 10 times less manual intervention, and a 25% improvement in ROI.
Read more: Effective Data Governance Strategy
Get started with your complimentary trial today and delve into our platform without any obligations. Explore our wide range of customized, consumption driven analytical solutions services built across the analytical maturity levels.
Start your free trialThe Benefits of a Data Governance Framework
Implementing a w ell-planned data governance framework offers a range of benefits to organizations, including:
- Improved Data Quality: By establishing clear policies and processes for data management, a data governance framework helps ensure that data is accurate, complete, and consistent, reducing the risk of errors and improving decision-making.
- Enhanced Data Security: A data governance framework outlines clear policies and procedures for data access, storage, and protection, minimizing the risk of data breaches and unauthorized access.
- Increased Compliance: By aligning data management practices with relevant regulations and industry standards, a data governance framework helps organizations avoid costly penalties and legal issues.
- Better Data Utilization: With a clear understanding of data ownership, roles, and responsibilities, organizations can leverage their data assets more effectively, driving innovation and competitive advantage.
- Streamlined Data Management: A data governance framework provides a centralized approach to data management, reducing duplication of effort and ensuring that data is managed consistently across the organization.
How Do Data Governance Frameworks Work?
Data governance frameworks come to fruition by bringing in a set of policies, procedures, and standards that govern the management of data within an organization. These frameworks typically include the following components:
- Data Governance Policies: Policies that define how data will be managed, including data quality, security, and privacy requirements.
- Data Roles and Responsibilities: Clearly defined roles and responsibilities for data management, including data owners, data stewards, and data custodians.
- Data Governance Processes: Processes for data management, including data acquisition, storage, and distribution, as well as processes for data quality management and data security.
- Data Governance Tools: Tools and technologies that support data governance, such as data cataloging, data lineage, and data quality management software.
By implementing these components, organizations can make sure that data is managed consistently and effectively across the organization, driving better decision-making and improved business outcomes. Implementing effective data governance stewardship is essential for organizations to manage customer data efficiently and comply with regulatory requirements such as the General Data Protection Regulation (GDPR). By utilizing data governance enablers, businesses can break down data silos and foster data democratization, making information available across departments. The Data Management Body of Knowledge (DAMA-DMBOK) brings in a comprehensive framework for data management, ensuring best practices are followed. Leveraging customer data platforms (CDP) and a data tracking plan template helps organizations monitor and secure data, while strong technical skill sets among employees ensure the effective implementation of these systems.
Conclusion
Including an effective data governance is essential for driving innovation, improving decision-making, and achieving competitive advantage. By implementing a robust data governance framework, organizations can ensure that data assets are managed consistently and effectively across the organization, while also minimizing the risks associated with data breaches, compliance issues, and poor data quality.
Effective data governance stewardship is crucial for breaking down data silos and promoting data democratization within organizations. By leveraging data governance enablers such as comprehensive data management frameworks outlined in the Data Management Body of Knowledge (DAMA-DMBOK), companies can ensure that customer data is accurately and securely managed. Implementing customer data platforms (CDP) and utilizing a data tracking plan template helps maintain compliance with regulatory requirements like the General Data Protection Regulation (GDPR). Additionally, having a well-versed technical skill sets within the team is essential in managing and safeguarding customer data effectively.
Whether you are just starting to develop your data governance strategy or looking to refine an existing framework, there are many resources and examples available to help guide your efforts. By partnering with experts like Quantzig, organizations can incorporate cutting-edge technologies and best practices to streamline data governance processes and ensure that data assets are managed effectively and efficiently.